Exploring Explainable AI Conferences: Insights & Trends
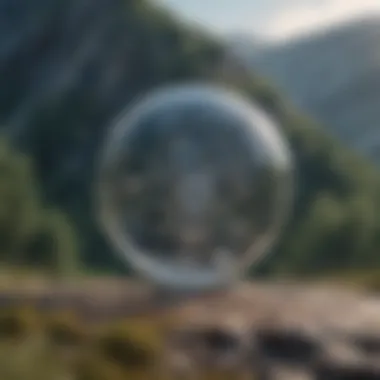
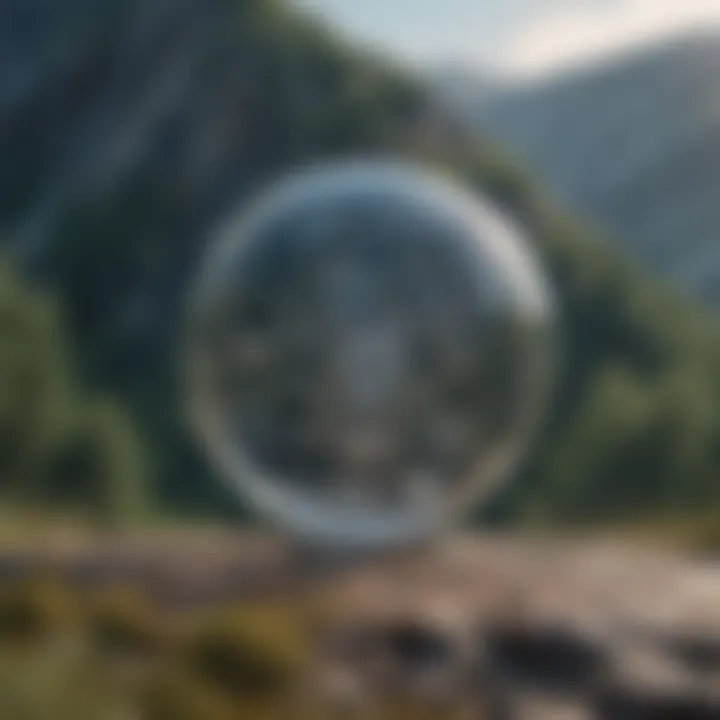
Intro
The discourse surrounding explainable artificial intelligence (AI) has gained substantial traction in recent years. As AI systems infiltrate various domains from healthcare to finance, the demand for transparency in decision-making processes has grown correspondingly. Organizations are increasingly aware that understanding AI mechanisms not only drives innovation but also mitigates risks associated with bias and accountability. This emergent need has precipitated the organization of numerous conferences focused on explainable AI.
These conferences serve as vital platforms where researchers, industry experts, and policy makers converge to discuss the implications of AI interpretability. Attendees can foster collaboration, share cutting-edge research, and debate regulations that govern AI applications. With a focus on transparency, these events play an instrumental role in shaping the future landscape of AI implementation.
By examining the key themes and future directions outlined in this article, stakeholders can better navigate the evolving field of explainable AI. The synthesis of knowledge provided here will be indispensable for those seeking to remain at the forefront of this transformative technology.
Prologue to Explainable AI
The realm of artificial intelligence is vast and continues to expand rapidly. Amid this growth, the concept of Explainable AI emerges as a crucial focal point. This term refers to methods and techniques in AI that make the behavior and decisions of machine learning models more understandable to human users. The increasing complexity of models often leads to what some term the "black-box" dilemma, where the reasoning behind AI outputs is obscured. Therefore, fostering comprehension around AI processes not only enhances user trust but also ensures compliance with ethical standards and regulations.
In discussions about AI, especially at conferences, practitioners must navigate a landscape filled with technical advances, societal impacts, and regulatory challenges. The integration of explainability into AI not only addresses practical concerns but also aligns with broader initiatives aimed at responsible AI deployment. This is why understanding the fundamentals of Explainable AI is vital for anyone engaged in AI research, development, or policy creation.
Definition of Explainable AI
Explainable AI encompasses a variety of techniques designed to provide insight into how AI systems operate. One can categorize these techniques broadly into two groups: model-agnostic and model-specific methods. While model-agnostic methods, like LIME or SHAP, work across different algorithms by focusing on the output, model-specific methods are tailored to the inner workings of particular algorithms, such as decision trees or neural networks. The key objective remains the same across categories: to uncover the reasoning behind predictions and decisions made by AI systems.
The inability of many models to provide clear explanations leads to challenges in fields where accountability is paramount, such as finance and healthcare. It is in these sectors where Explainable AI plays a critical role, as it allows practitioners to understand not just the conclusions drawn but also the pathways taken to reach them.
Importance of Explainability in AI
Explainability in AI is not merely a technical requirement; it serves several essential functions. First, it enhances accountability. When decisions are made, particularly those affecting individuals or communities, it is imperative that those responsible can justify outcomes. With strong mechanisms for understanding AI decisions, stakeholders can better address any adverse effects.
Second, explainability fosters trust among users. In scenarios like criminal justice or medical diagnostics, the consequences of AI errors can be significant. By providing clear explanations of decision-making processes, organizations can create confidence in their systems. Users are more likely to support and utilize tools that they can understand and trust.
Lastly, the regulatory landscape is evolving to emphasize the need for explanation. Regulatory bodies are beginning to require transparency in AI systems, making it a legal necessity in certain contexts. Understanding the importance of explainability, therefore, becomes vital, as it aligns both ethical and legal considerations.
"The integration of Explainable AI is not just a trend; it is a necessity for ethical AI deployment necessary for societal acceptance."
In summary, the dialogue surrounding Explainable AI is crucial as it intersects with technical innovation, ethical deployment, and legal compliance. This sets the stage for deep explorations into Explainable AI within conferences that draw a diverse array of participants and stakeholders from academia, industry, and policy.
Overview of Explainable AI Conferences
Explaining the role of Explainable AI conferences is crucial. These events serve as platforms for discourse and collaboration among diverse groups. They gather experts from academia, industry, and government, fostering an environment for dialogue and learning. Such forums highlight advancements in AI interpretability, addressing key challenges and sharing new methodologies. The relevance of these conferences cannot be overstated. They contribute to a better understanding of how AI can offer transparency and accountability, vital elements for trust in AI technologies.
Purpose and Goals of Conferences
The purpose of Explainable AI conferences revolves around several core aims. First, they aim to disseminate knowledge. Researchers present their findings, sharing insights on the latest trends and discoveries. Attendees gain access to cutting-edge research that informs future directions in the field.
Second, these conferences facilitate networking. Participants have many opportunities to connect and collaborate. Such interaction can lead to partnerships or projects that push boundaries of what's possible in AI. These conferences also play a significant role in shaping policy discussions. Influencers from various sectors come together to discuss ethical considerations, aiming to establish frameworks that guide responsible AI development.
Participants and Stakeholders
Exploring the landscape of attendees reveals a rich mixture of stakeholders. Researchers from universities participate extensively, bringing academic perspectives to practical problems. Industry leaders share their experiences, exposing academia to real-world applications of AI technologies.
In addition, government representatives often engage in these discussions. They contribute to dialogues on regulation and policy, ensuring that the needs and concerns of society are considered.
Moreover, non-profit organizations and advocacy groups are significant participants in these conferences. They help to elevate discussions surrounding ethical implications of AI technologies. This mix of participants leads to comprehensive insights, enriching the conversations and findings discussed.
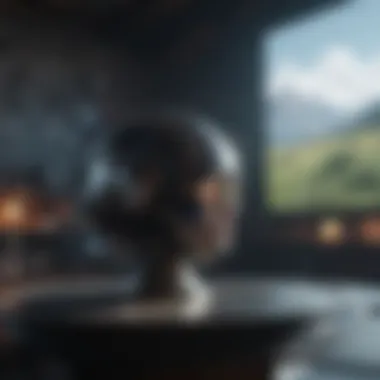
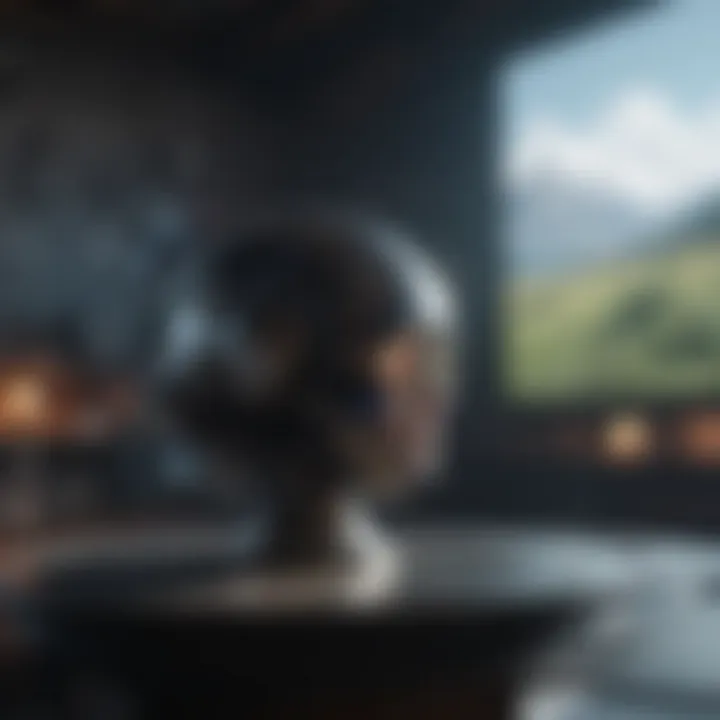
Understanding the full spectrum of stakeholders helps build a robust framework for addressing challenges in Explainable AI.
Through organizing and attending these conferences, all involved can drive the conversation toward solutions. The interaction among students, educators, professionals, and policymakers emphasizes the collaborative effort needed to advance the field of Explainable AI.
Notable Explainable AI Conferences
The significance of notable explainable AI conferences cannot be overstated. These events serve as pivotal platforms for sharing knowledge, sparking discussions, and fostering collaborations among stakeholders in the AI community. They bring together researchers, practitioners, and policymakers, creating an environment conducive to innovation and growth. Whether one is a student, a researcher, or a professional in the field, attendance at these conferences provides invaluable insights into the latest trends and advancements in explainable AI.
A Comprehensive Listing of Key Events
The landscape of explainable AI conferences is rich and varied, showcasing events organized around the globe. Here are key conferences that play an important role in this domain:
- Explainable AI Workshop at NeurIPS: Focuses on new research in explainable AI and its applicability across diverse fields.
- ACM Conference on Fairness, Accountability, and Transparency (FAccT): This conference discusses accountability in automated decision-making systems and the role of explainability in promoting fairness.
- IEEE International Conference on Data Mining (ICDM): Features a segment dedicated to interpretability in data mining, including novel methods for explainability in machine learning algorithms.
- SIGKDD Conference on Knowledge Discovery and Data Mining: This event also includes tracks that concentrate on the explanation of model decisions and approaches to provide better transparency to users.
- International Conference on Machine Learning (ICML): Focuses on a broad range of AI topics, including methodologies to improve model interpretability.
Each of these events not only highlights the cutting-edge research being conducted but also sets the tone for discussions around regulation and ethical considerations regarding AI technologies.
Highlighting Influential Talks and Panels
Conferences in the explainable AI space often feature influential talks and panels that shape the narrative surrounding AI interpretability. Renowned speakers from academia and industry share their findings and perspectives, contributing to an enriched understanding of explainable AI. For instance:
- Dr. Kate Crawford has spoken on the societal impacts of AI and advocated for transparency in AI systems. Her talks emphasize the need for comprehensive dialogues about AI ethics.
- Dr. Fei-Fei Li, a prominent figure in the AI community, often addresses the balance between innovation and ethical responsibilities in AI development.
- Panel discussions frequently bring together leading experts to address pressing questions. For example, topics may revolve around the implications of black-box models and their transparency in real-world applications.
"The most effective way to demystify AI for users is through collaborative discussions that involve multiple perspectives."
In summary, notable explainable AI conferences are essential for facilitating knowledge exchange, highlighting advancements, and addressing challenges within the field. These conferences emphasize the importance of continuous engagement and dialogue among all stakeholders for the advancement of explainable AI.
Themes and Topics in Explainable AI
The exploration of explainable AI (XAI) requires an understanding of its key themes and topics. Addressing the complexities of AI systems demands that researchers and practitioners engage with significant trends and applications. This section will illuminate recent research trends, offering insights into how these inform practice and policy. Furthermore, it will outline the broad applications of explainable AI across various industries, demonstrating its importance in real-world scenarios and influencing stakeholder decisions.
Recent Research Trends
In recent years, the landscape of explainable AI research has grown considerably. New methodologies have emerged, emphasizing the necessity of transparency in AI systems. Some notable trends include:
- Interpretable Machine Learning: Researchers are focusing on developing machine learning models that are inherently interpretable. This means that these models provide explanations that can be understood by human users, simplifying the complexities often associated with black-box models.
- Algorithmica l Fairness: With increasing scrutiny over bias in AI, findings in this area are shaping a new direction for explainability. This trend supports the necessity for equitable AI systems with explanations that clarify how fairness is maintained or compromised within model outputs.
- Evaluation Metrics for Explainability: There is a rise in research devoted to establishing metrics assessing the effectiveness of explanations. Defining what it means for an explanation to be good or useful is pivotal for moving forward in this field.
Engaging with these trends is important not just academically, but also for industries relying on AI, as they can anticipate future developments and enhance their systems accordingly.
Applications Across Industries
The applications of explainable AI span multiple sectors, each presenting unique challenges and opportunities. The benefits of implementing explainable techniques are evident in numerous industries:
- Healthcare: In healthcare, explainable AI plays a crucial role in diagnostic systems. Clinicians require transparent models to trust AI-generated recommendations. Interpretability allows for better patient care, as professionals can understand and justify AI suggestions.
- Finance: Within finance, algorithmic decisions influence lending and investment strategies. XAI can help in auditing systems to ensure fairness and accountability. Explanations support regulatory compliance and bolster consumer trust.
- Autonomous Vehicles: In the realm of self-driving technology, understanding how decisions are made is vital. Explainability contributes to safety, allowing developers and users to grasp the operational rationale behind the driving decisions made by the AI.
- Manufacturing: Predictive maintenance in manufacturing utilizes explainable AI to reduce downtime. Manufacturers can understand how predictions are made, allowing for better decision-making.
The compelling benefits of these applications highlight the need for continued dialogue and development in the field of explainable AI.
"Transparency and accountability are just as important in AI as they are in any other field, as reliance on machines grows."
As industries grapple with the integration of AI, themes and topics of explainability will persist as central facets for the efficacy and ethical operation of AI systems.
The Role of Government and Regulation
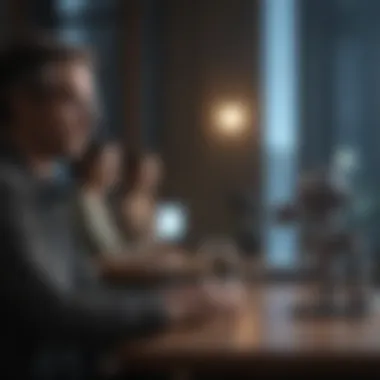
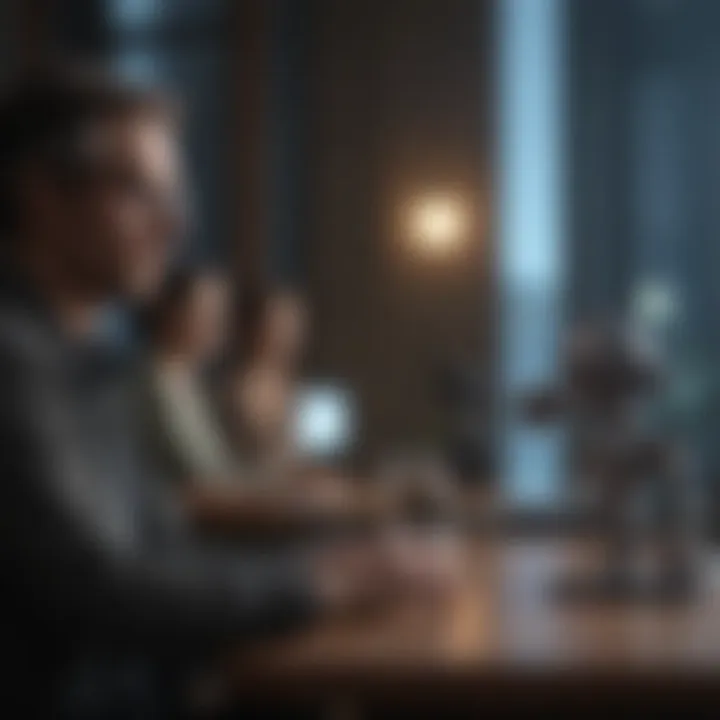
Government and regulation play a crucial role in the development and adoption of explainable AI technologies. As AI applications become more integrated into various sectors, regulatory frameworks are necessary to ensure transparency, accountability, and ethical standards. The implementation of policies that guide the usage of AI can enhance public trust and facilitate responsible innovation. Accordingly, this section explores the concept of policy frameworks and guidelines as well as the ethical considerations surrounding AI.
Policy Framework and Guidelines
A robust policy framework for explainable AI encompasses a set of guidelines that aim to govern its deployment across industries. These guidelines can highlight best practices for organizations developing AI solutions. Specific aspects to consider include:
- Transparency: Policies should advocate for clear communication regarding how AI systems work, which models are being used, and the data they rely upon.
- Accountability: Organizations must be required to disclose developers and decision makers responsible for AI outcomes, allowing for traceability and evaluation of AI-driven decisions.
- Inclusive Design: Policy should encourage creating AI systems that serve diverse populations, mitigating bias and discrimination.
Effective regulatory measures can assist in setting standards for both researchers and practitioners. The frameworks should not only focus on compliance but also promote innovation by providing guidelines that foster a conducive environment for improvement and collaborations.
Ethical Considerations in AI
The integration of explainable AI intersects with various ethical considerations that must be addressed by both developers and regulators. Key ethical themes to contemplate include:
- Bias and Fairness: AI models may inherit biases from historical data. The consequences can be profound, especially in sectors like healthcare, finance, and law enforcement. Regulations must demand methodologies that actively assess and mitigate these biases.
- User Privacy: AI systems often require substantial amounts of data to function efficiently. Policies need to protect individual privacy and ensure that data collection is justified and transparent.
- Autonomous Decision-Making: The extent to which AI makes autonomous decisions raises questions about the implications for human oversight. Clear guidelines are necessary for situations where AI systems demonstrate self-guided performance, with policies addressing the limits of such autonomy.
Emerging Technologies in Explainable AI
Emerging technologies in explainable AI play a crucial role in enhancing the transparency and interpretability of AI systems. As the use of AI proliferates across various industries, the need for clear explanations of decisions made by these systems grows also. Based on current trends, several key areas are evolving swiftly, each presenting unique benefits and considerations.
Advancements in Machine Learning Algorithms
Advancements in machine learning algorithms are making significant contributions to the field of explainable AI. The introduction of interpretable models such as decision trees, linear regression, and more advanced techniques like LIME (Local Interpretable Model-agnostic Explanations) and SHAP (Shapley Additive exPlanations) allow for a better understanding of model behaviors. These algorithms provide insights into how input features affect outputs.
Key points of interest include:
- The development of algorithms that prioritize explainability without sacrificing accuracy.
- The adaptability of these models to a variety of data types which enhances their usability across different domains.
- Focused efforts on producing more user-friendly interpretations that can cater to non-experts in AI fields.
These advancements ensure that stakeholders can trust and engage with AI-driven outcomes, essential for sectors like healthcare, finance, and autonomous vehicles.
Integrative Approaches to AI Interpretability
Integrative approaches to AI interpretability are becoming increasingly important. These methods combine various techniques to enhance the transparency of AI models. By employing a combination of rule-based systems with neural networks, practitioners can better explain complex behaviors of AI systems.
Some integrative approaches include:
- Model distillation, where complex models are simplified into more interpretable forms.
- Visualization techniques, that graphically represent decision processes aiding comprehension.
- Post-hoc analysis, facilitating explanations after model deployment to assess performance and fairness in decision-making.
The use of multiple approaches allows for a more holistic view of AI efficacy while accommodating different audience needs.
"A well-designed interpretability framework can bridge the gap between model performance and user understanding."
In summary, emerging technologies continue to shape explainable AI by enhancing machine learning algorithms and adopting integrative approaches. These developments are key to establishing trust and accountability in AI systems.
Challenges and Solutions in Explainable AI
The field of Explainable AI faces numerous challenges that are crucial to address for the advancement of technology. Understanding these hurdles is essential for the development of effective solutions. In conferences dedicated to Explainable AI, experts gather to discuss these issues and work towards practical applications of explainable methods. The collaborative environment fosters innovation and encourages diverse perspectives, which can lead to significant advancements in the understanding of AI systems.
Technical Hurdles
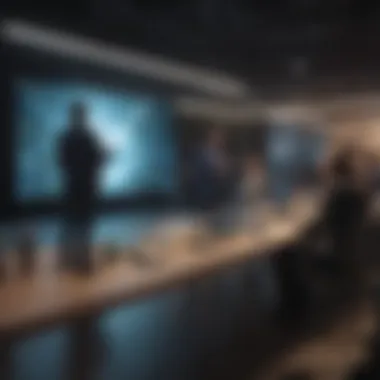
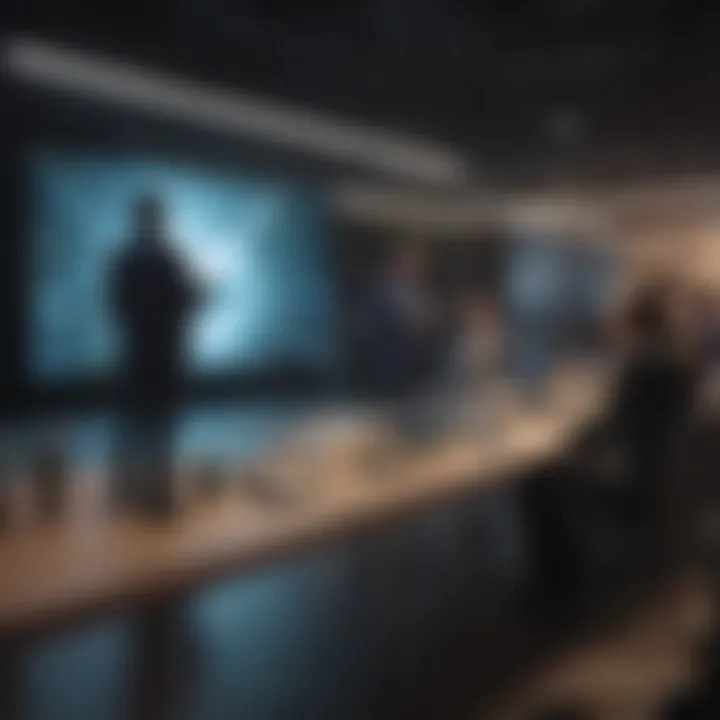
Technical challenges in Explainable AI often stem from the complexity of machine learning algorithms. Many of these models are inherently intricate, making it difficult to decipher their internal workings. For instance, deep learning models, while powerful, can act as "black boxes" where the decision-making processes remain hidden. This lack of interpretability raises concerns about their reliability, especially in sensitive areas like medicine or finance. Achieving transparency without sacrificing model performance is a key issue.
Efforts to enhance interpretability include developing tools and frameworks that allow researchers and practitioners to visualize model outputs. For example, techniques like LIME (Local Interpretable Model-agnostic Explanations) help understand model decisions on a local scale. However, there is still much work to be done to create universally accepted methods for interpreting various models.
Moreover, the integration of explainability into existing workflows can prove challenging. Organizations might need to invest in training and resources to understand how explainable models function. This requires a cultural shift, fostering an environment that values transparency and ethics in AI development.
User-Centric Challenges
User-centric challenges also play a significant role in the broader perspective of Explainable AI. It is not enough for AI systems to be interpretable; they must also resonate with the users who rely on them. AI practitioners must consider how different stakeholders, including decision-makers and laypersons, perceive and understand model explanations. There is often a gap in the interpretation skills of end-users, which can lead to misinterpretation of AI recommendations.
Research indicates that a one-size-fits-all approach does not work when it comes to explanations. Different users might require various types of explanations based on their background and expertise. For example, technical users may prefer quantitative data, while non-technical users may respond better to visual aids or simple narratives. Tailoring explanations is necessary to ensure user trust and adherence to AI systems.
To make strides in this area, conferences can feature workshops and sessions focusing on best practices for user training and communication strategies. By sharing insights on effective user engagement, stakeholders can build systems fostering trust and comprehension among varying audiences.
Addressing challenges in Explainable AI is a twofold endeavor, requiring attention to both technical and user-experience aspects to truly enhance AI transparency and usability.
In summary, overcoming technical hurdles and user-centric challenges in Explainable AI is paramount for achieving effective implementation and trust in AI systems. Continuing discussions and collaborations in conferences can help refine strategies to navigate these complexities.
Future Directions for Explainable AI Conferences
Explorable dynamics in the field of Explainable AI (XAI) are increasingly taking center stage in various discussions. The future of explainable AI conferences is critical for shaping the research agenda and fostering collaboration among different sectors. As AI systems become more complex, the potential for misunderstandings and misuse grows. Thus, the evolution of conferences in this domain can help address emerging issues and create pathways for effective solutions.
Potential Innovations in Conference Structure
Future conferences on explainable AI can benefit from structural innovations that enhance participant engagement and knowledge sharing. Online formats have become more prevalent due to recent global events, showcasing the advantages of virtual accessibility. However, hybrid models, combining in-person and online interactions, could provide a balance, allowing for broader attendance and participation.
Topics addressed at conferences may need to evolve to include not only technical advancements but also socio-ethical discussions. Workshops that focus on best practices in AI implementation can foster practical skills among attendees.
Other innovations might include using collaborative platforms for real-time discussions. These could allow participants to share insights, ideas, and questions live, enhancing the overall dialogue and making it more inclusive. Incorporating varied formats, such as round tables and panel discussions, would enrich the participants' experience.
"Innovative structures can transform conferences into interactive learning environments, encouraging diverse perspectives in explainable AI."
Expanding Global Participation
The issue of global participation is paramount. Explainable AI is an area that can benefit from diverse insights. Bringing together voices from different regions can foster inclusive discussions and broaden the understanding of local challenges and solutions. Therefore, efforts must be made to create conferences that are accessible to participants from underrepresented areas.
This could involve offering support for travel grants, financial assistance, or providing conference stipends. Additionally, translating materials and presentations into multiple languages will make the conferences more approachable.
Engagement with international researchers and practitioners can also lead to collaborative projects, promoting the exchange of knowledge and technology. Recent advancements in communication technology now allow for effective networking across borders, making global participation more feasible than before.
Enhancing diversity in participation could contribute significantly to the richness of discussions and research outcomes in the explainable AI field.
The continual evolution of explainable AI conferences will play a vital role in shaping its future, facilitating neglected yet urgent discussions, fostering collaboration, and encouraging the adoption of best practices among industry professionals, researchers, and policymakers.
Finale
In the field of explainable artificial intelligence (AI), the conclusion signifies an essential component in the discourse surrounding the topic. As we have explored throughout this article, various facets of explainable AI conferences play a pivotal role in shaping understanding and advancing research.
Summarizing Insights on Explainable AI
The insights acquired from these conferences highlight the increasing recognition of explainability as a crucial element in AI development. Participants, ranging from researchers to industry professionals, gather to discuss challenges and breakthroughs. Topics featured can encompass advancements in methodologies, ethical considerations, and real-world applications across numerous industries. Eventually, this collective knowledge contributes to refining AI systems, improving transparency, and fostering trust among users.
"Understanding the outcomes of these conferences is vital for anyone involved in the AI ecosystem as they showcase the growing need for machines that not only make decisions but also explain their reasoning behind them."
The Importance of Continued Dialogue
Continued dialogue in the sphere of explainable AI is indispensable. The rapid development of AI technologies necessitates ongoing conversations among stakeholders. This dialogue keeps everyone informed about emerging trends, regulatory issues, and ethical challenges. It serves as a forum for dialogue that inspires innovation and collaboration across multiple sectors. By engaging in these discussions, researchers and practitioners can anticipate and address potential problems before they escalate.