Exploring Cutting-Edge Innovations in AI Technology
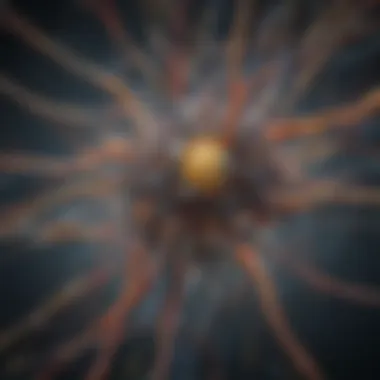
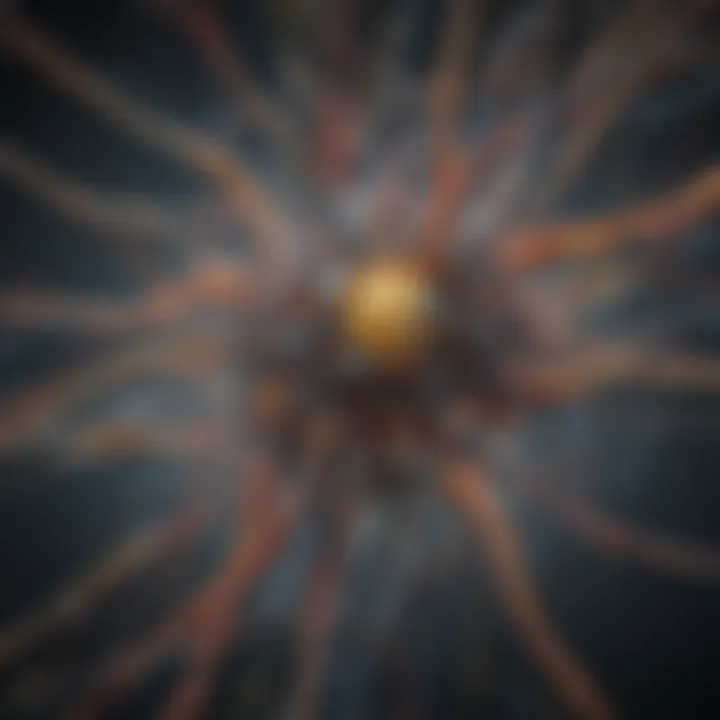
Intro
Artificial Intelligence (AI) technology has transformed rapidly in recent years. It goes beyond simple automation of tasks and delves into complex algorithms and machine learning systems. This article aims to illuminate the latest advancements in AI technology, looking at innovations and applications across various industries. Additionally, it addresses the ethical issues and future directions that are becoming increasingly important in discussions surrounding AI.
Research Overview
Summary of Key Findings
Recent developments highlight several fascinating trends in AI. Key findings include:
- Deep Learning Advancements: New neural network architectures are improving accuracy in areas such as image recognition and language processing.
- Natural Language Processing: Systems like OpenAI's GPT-3 have improved understanding and generation of human language, allowing for more nuanced interactions.
- AI Ethics: Discussions around fairness, accountability, and transparency in AI decision-making processes are now at the forefront of research.
Relevance to Current Scientific Discussions
Understanding AI is vital for students, researchers, and professionals as it permeates various sectors from healthcare to finance. Ongoing conversations in scientific circles emphasize the importance of interdisciplinary collaboration, ensuring that diverse perspectives are represented in AI development.
Methodology
Research Design and Approach
This article employs a qualitative research design. It reviews existing literature on AI technology, focusing on publications from reputable journals and conferences. Primary sources include peer-reviewed articles and white papers from leading organizations in AI research.
Data Collection and Analysis Techniques
Data for this review is collected from academic databases and established AI publications. The synthesis involves thematic analysis, identifying trends, and compiling them into coherent narratives. By organizing content thematically, the discussion remains focused and relevant to the current state of AI advancements.
"AI technology is not just a trend; it is reshaping the fabric of society, ensuring a future that is more productive and efficient."
Epilogue
In summary, a detailed understanding of the latest AI technology enables individuals and organizations to leverage its potential effectively. From deep learning innovations to pressing ethical concerns, keeping abreast of these developments is essential for stakeholders across multiple fields.
The Evolution of AI Technology
The evolution of AI technology is crucial for understanding the current and future landscape of artificial intelligence. This section delves into the historical milestones and recent breakthroughs that have shaped AI as we know it today. By exploring these elements, we can comprehend the benefits, implications, and potential paths ahead for AI technology.
The development of AI technology is not just about creating intelligent machines; it reflects the changing relationship between humans and technology. With each milestone, we notice an increase in both the capability and the complexity of AI systems. Recognizing these advancements aids students, researchers, educators, and professionals in contextualizing and anticipating future developments in AI.
Historical Milestones in AI
The history of AI is marked by several key milestones that illustrate its evolution. In the 1950s, pioneers like Alan Turing laid the groundwork by proposing the concept of machines that could think. His famous Turing Test spurred debate on intelligence and computation.
In the 1960s, programs such as ELIZA showcased early natural language processing, allowing machines to engage in basic conversation. This period also saw advancements in rule-based systems, which formed the basis for expert systems used in various industries.
The 1980s introduced a wave of interest in neural networks, driven by a quest to mimic human brain function. These networks remained limited until the late 1990s when researchers began to see significant improvements in computational power.
Today, AI has matured, influenced by developments in deep learning and vast amounts of data available. The transition from rule-based systems to machine learning models marks a significant turning point, and it allows for more sophisticated and adaptable AI applications.
Recent Breakthroughs
In recent years, AI technology has experienced unprecedented breakthroughs that have accelerated its adoption and integration into various sectors. For instance, deep learning techniques, now prevalent, have led to remarkable achievements in fields such as computer vision, language processing, and robotics. The success of models like OpenAI's GPT-3 and Google's BERT illustrates how advanced algorithms can generate human-like text and comprehend context effectively.
Moreover, AI's impact on industries has become widespread. In healthcare, applications like IBM Watson have enhanced diagnostics and personalized treatment plans. In finance, algorithms analyze market trends and optimize trading strategies. The automotive industry is leveraging AI for the development of self-driving cars, pushing boundaries on safety and efficiency.
Through these breakthroughs, AI is not just a technological novelty; it is a driving force reshaping our world. Each advancement opens new possibilities while also posing challenges to understand and address, especially in areas such as privacy and ethics.
"The evolution of AI technology is not a linear path; it is a tapestry woven from myriad innovations and experiments."
In summary, the evolution of AI technology is essential for grasping its present capabilities and future potentials. By appreciating the historical milestones and recent breakthroughs, we gain valuable insights into the trajectory of artificial intelligence and its implications for society.
This understanding allows us to engage with the ethical questions and challenges that arise as AI technology continues to evolve and integrate deeper into our daily lives.
Core Areas of AI Research
In the field of artificial intelligence, core areas of research are pivotal to the understanding and development of AI technologies. These areas, including machine learning, natural language processing, and computer vision, form the backbone of modern AI applications. They not only drive innovation but also provide frameworks for addressing complex real-world problems. By delving into these core research areas, we gain insights into how AI can be applied effectively across various sectors.
Machine Learning
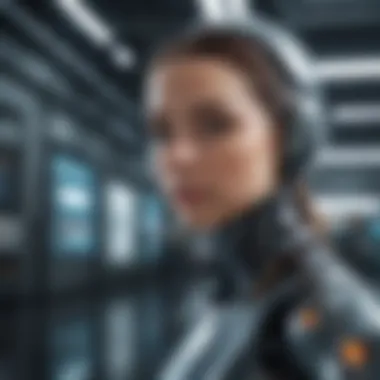
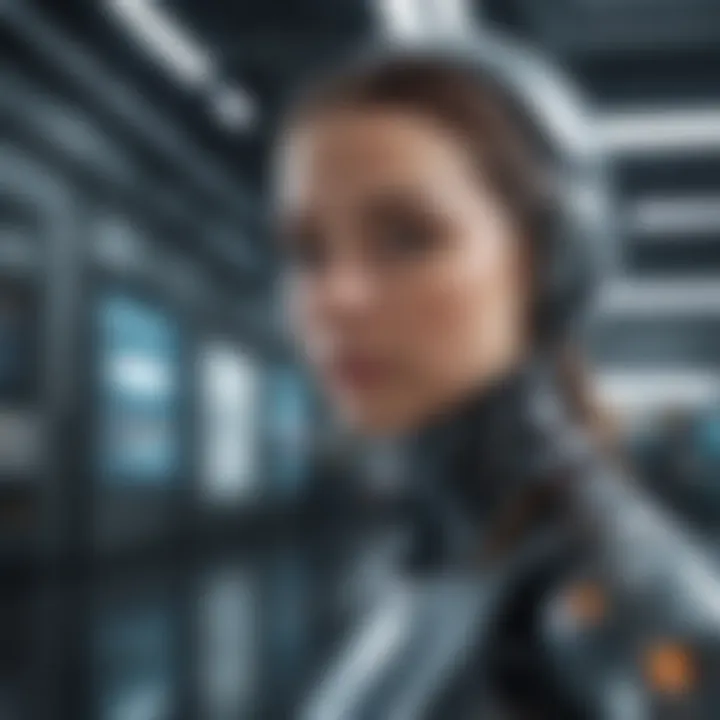
Machine learning is a subset of AI focusing on the development of algorithms that allow computers to learn from and make predictions based on data. Its importance lies in its ability to automate processes and generate insights from vast amounts of information.
Supervised Learning
Supervised learning is a type of machine learning where the algorithm is trained on labeled data. This means the input data is paired with corresponding output labels. A key characteristic of supervised learning is its straightforward approach to modeling relationships in data, making it a popular choice for tasks such as classification and regression.
Its unique feature is the necessity of a training dataset that includes the correct answers. One advantage is its relatively high accuracy in predictions, which is vital for applications in sectors like healthcare and finance. However, a disadvantage is that it requires a substantial amount of labeled data, which can be time-consuming and costly to obtain.
Unsupervised Learning
Unsupervised learning, in contrast, involves training algorithms on data without labeled responses. This method is crucial for discovering hidden patterns or intrinsic structures in data. A key characteristic is its ability to operate on unlabeled data, making it beneficial for exploratory data analysis and clustering.
A unique feature of unsupervised learning is its capacity to automatically categorize data, such as grouping customers by purchasing behavior. While its advantage lies in the lack of need for labeled datasets, a disadvantage can be the difficulty in interpreting the results, as it may produce classifications that lack clear meaning.
Reinforcement Learning
Reinforcement learning is an area focused on how agents ought to take actions in an environment to maximize cumulative rewards. The core characteristic is its use of a reward system to evaluate the effectiveness of actions taken. It is particularly beneficial in situations where the optimal action sequence is not clear, such as in robotics and games.
This method's unique feature is its emphasis on trial and error, allowing algorithms to learn from past experiences. While it can lead to highly effective strategies, a notable disadvantage is the potential inefficiency in learning if the reward signals are sparse or delayed.
Natural Language Processing
Natural language processing (NLP) involves the interaction between computers and humans through natural language. This area is vital as it helps computers understand, interpret, and respond to human language in a meaningful way.
Sentiment Analysis
Sentiment analysis focuses on determining the emotional tone of a piece of text. This is significant for businesses to gauge public opinion and customer feedback. A key characteristic is the ability to classify text into positive, negative, or neutral sentiments. Its benefit lies in informing marketing strategies and customer relationship management.
The unique feature of sentiment analysis is its application to vast text datasets, such as social media comments. However, a disadvantage is the challenge in accurately capturing context and sarcasm, which can lead to misinterpretations.
Language Translation
Language translation aims to convert text from one language to another. This capability is critical in facilitating global communication and information dissemination. A key characteristic of this technology is its ability to process and analyze multiple languages efficiently.
One unique feature is the development of models like Google Translate, which harness extensive bilingual corpora for training. While the advantages include increased accessibility of information, a disadvantage is the difficulty in maintaining nuances and idiomatic expressions during translation.
Text Generation
Text generation focuses on creating coherent and contextually relevant text from prompts. This is crucial for applications like chatbots and content creation. The core characteristic is the use of predictive models to generate human-like text.
Its unique feature is the ability to produce diverse outputs from the same input due to underlying probabilistic models. While it excels at creativity, a disadvantage is potential issues with accuracy and factuality, as automated generation may lead to misleading or incorrect information.
Computer Vision
Computer vision aims to enable machines to interpret and make decisions based on visual data from the world. This area is essential for applications in industries ranging from healthcare to security.
Image Classification
Image classification involves assigning a label to an image based on its content. This is instrumental in applications such as medical imaging and autonomous vehicles. A key characteristic is its reliance on deep learning algorithms to achieve high accuracy.
A unique feature is its application to large image datasets, leading to improved model performance over time. However, a disadvantage includes the challenge of variability in images, which can affect the accuracy of classifications.
Object Detection
Object detection identifies and localizes objects within an image. This technology is vital for self-driving cars and surveillance systems. The key characteristic is its ability to provide both classification and localization information in one process.
The unique feature is the use of techniques like YOLO (You Only Look Once), which enables real-time object detection. While highly effective, a disadvantage is the computational resources required, especially for high-resolution images.
Facial Recognition
Facial recognition involves identifying or verifying a person from an image or video. This technology's importance spans security, marketing, and user authentication. A key characteristic is its ability to process and analyze facial features.
Its unique feature is the application of deep learning to enhance accuracy in various real-world conditions. However, ethical concerns regarding privacy and potential misuse present significant disadvantages in its adoption.
By exploring these core areas of AI research, it becomes evident how they interconnect and contribute to technological advancements in AI. They serve as critical building blocks that inform both theoretical and practical applications in diverse fields.
Current Applications of AI Technology
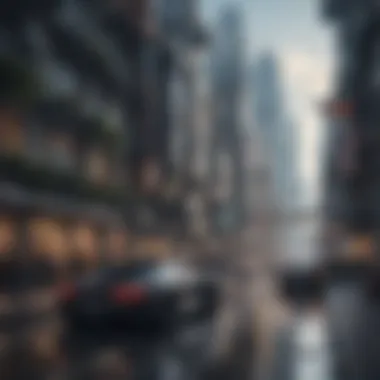
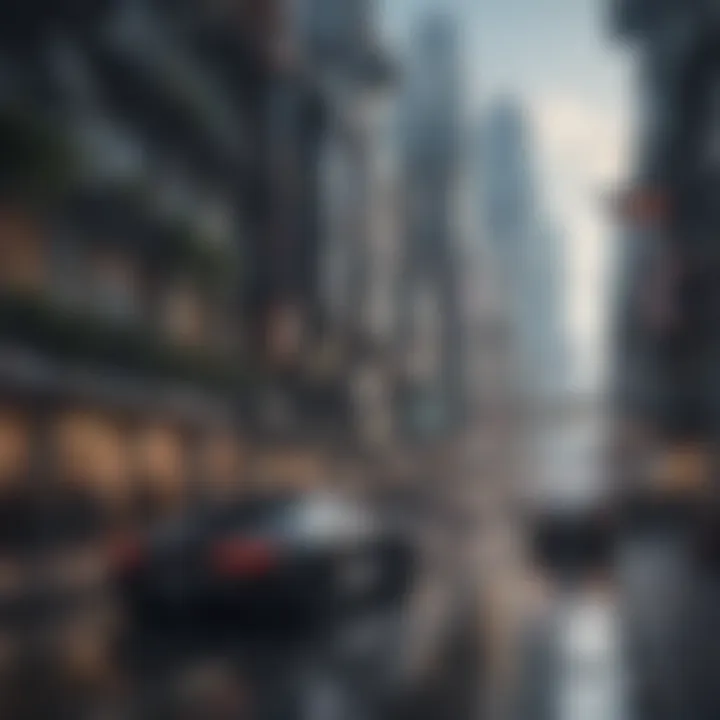
As artificial intelligence technology continues to develop, its current applications are vital components shaping various sectors. AI's real-world implementations demonstrate its potential to enhance operational efficiency, improve decision-making processes and provide better services in various industries. Understanding these applications provides insights into how AI is transforming healthcare, business, and education.
Healthcare Innovations
AI technology significantly impacts healthcare through innovations that enhance patient care and streamline processes.
Predictive Analytics in Patient Care
Predictive Analytics in patient care involves analyzing data to foresee potential health issues, enabling proactive management of diseases. This aspect of AI offers healthcare providers valuable insights based on patient history and data trends. It stands out due to its focus on prevention rather than reactive treatments.
The key characteristic of predictive analytics is its ability to analyze vast amounts of information quickly, highlighting patterns that may indicate future health risks. This capability makes it a popular choice among healthcare professionals aiming to improve patient outcomes.
A unique feature of this technology is its integration of machine learning algorithms, allowing it to refine its predictions as more data becomes available. However, there are disadvantages, such as potential data privacy concerns and the need for high-quality data to ensure accuracy.
AI in Medical Imaging
AI in medical imaging revolutionizes diagnostic processes by providing tools that can analyze and interpret images quickly and accurately. This technology contributes to efficiency in medical diagnostics, enabling faster identification of conditions like cancers or fractures.
The key characteristic of AI in medical imaging is its ability to process images with precision, often outperforming human interpretation in certain contexts. Its benefit lies in reducing the workload on radiologists, allowing them to focus on more complex cases.
A unique feature of AI in this area is its continual learning from new imaging data, which can improve diagnostic accuracy over time. The disadvantages may include reliance on systems that can be costly and the risk of misdiagnosis if algorithms are improperly calibrated.
Drug Discovery
AI in drug discovery accelerates the process of identifying new medications, thereby reducing costs and decreasing the time to market. With this approach, pharmaceutical companies can analyze biological data and simulate drug interactions efficiently.
The key characteristic here is the capacity to process massive datasets, enabling researchers to pinpoint promising compounds quickly. This makes AI a beneficial choice for the industry that traditionally faces lengthy development cycles.
The unique feature of this application is its ability to predict outcomes of clinical trials earlier in the process. However, there are disadvantages, such as the limited availability of high-quality datasets that can hinder the development of accurate models.
AI in Business
Businesses are increasingly leveraging AI technology to gain insights, automate operations, and enhance their supply chains. These applications are becoming integral to staying competitive in a rapidly changing market.
Customer Insights and Analytics
Customer insights using AI allow businesses to understand consumer behavior and preferences better. This application contributes significantly to enhancing marketing strategies and improving customer satisfaction.
The key characteristic of customer insights is the analyzing of consumer data in real-time, providing businesses with timely intelligence. This makes it a popular choice among data-driven companies.
A unique feature of this technology is its ability to segment customers based on behavior patterns, leading to more targeted marketing campaigns. However, there are disadvantages, including the risk of over-reliance on data, which may overlook the human aspects of customer relationships.
Automation of Operations
Automation of operations streamlines workflows through AI-driven processes. This aspect of AI reduces operational costs and minimizes human error by automating repetitive tasks.
The key characteristic of operational automation is increased efficiency. It offers a beneficial aspect of freeing employees to focus on more strategic initiatives.
A unique feature is its adaptability across various functions, from customer service to manufacturing. Yet, disadvantagesmay include workforce displacement concerns and challenges in integrating these systems into established workflows.
Supply Chain Management
AI plays a critical role in improving supply chain management by optimizing logistics, forecasting demand, and enhancing the management of inventory.
The key characteristic of AI in supply chain is its predictive capabilities. This allows businesses to make informed decisions, which is a beneficial factor in avoiding stockouts or excess inventory.
A unique feature is real-time tracking, enabling organizations to respond quickly to disruptions. However, certain disadvantages may involve the theoretical complexity and the necessity for initial investments in technology.
Role of AI in Education
AI is transforming education by creating personalized learning experiences, implementing tutoring systems, and improving assessment methods.
Personalized Learning Environments
Personalized learning environments leverage AI to tailor educational experiences to individual student needs. This deployment enhances engagement and effectiveness in learning.
The key characteristic is adaptability, allowing learning programs to adjust based on student progress. This makes it a beneficial choice for educators aiming for inclusivity and diversity in learning styles.
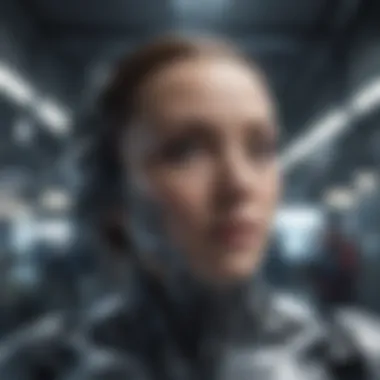
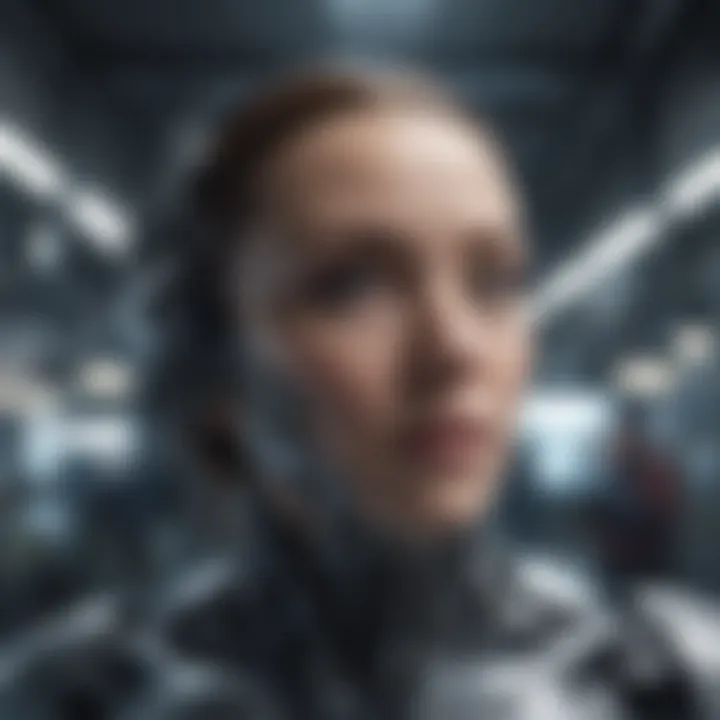
A unique feature of personalized education is tracking student activity and adjusting materials accordingly. Disadvantages could include the necessity for substantial initial programming and data input.
AI Tutors and Assistants
AI tutors and assistants provide additional support to students, complimenting traditional education methods. They serve as accessible resources at any time for learners needing help.
The key characteristic is availability outside standard classroom hours, making them a valuable tool for additional learning.
These systems can offer feedback and educational resources. Still, there are disadvantages, including the possibility of tutors lacking understanding of nuanced student needs.
Assessment and Feedback
AI-driven assessment systems offer innovative ways to evaluate student performance. These systems can provide immediate feedback and in-depth analysis.
The key characteristic of this application is efficiency in grading and the provision of personalized feedback. This makes it a popular choice for educational institutions looking to support learners more effectively.
A unique feature is the capability to analyze student work in real-time. Nonetheless, disadvantages may involve concerns regarding the accuracy and fairness of the evaluation process.
Ethical Considerations in AI
The advent of artificial intelligence has transformed numerous sectors, from healthcare to entertainment. However, with such rapid advancement comes a set of ethical dilemmas that necessitate careful consideration. Ethical considerations in AI technology are essential for ensuring that the innovations we embrace do not inadvertently reinforce societal inequities or erode fundamental human rights. This segment examines critical elements such as bias, privacy, and accountability to offer a balanced view of AI's implications.
Bias and Fairness in AI Systems
The issue of bias in AI is not only technical; itโs profoundly social. AI systems often reflect the biases present in the data used to train them. If the training data is skewed towards a certain demographic, the AI's decisions can also become biased, leading to unfair treatment of individuals from underrepresented groups. For example, in hiring algorithms, a focus on past employee data may inadvertently privilege candidates from particular backgrounds, leaving deserving candidates on the sidelines.
To mitigate this risk, ongoing research emphasizes developing fair AI algorithms. Some methods involve diverse data sourcing, testing algorithms across different demographic groups, and implementing transparency in AI decision-making processes.
Privacy Concerns
As AI systems become more integrated into everyday life, the collection and processing of personal data raise significant privacy concerns. Itโs not just about how data is collected, but about how it is used and who has access to it. With AI algorithms processing vast amounts of data, maintaining user privacy becomes a daunting task.
Regulatory frameworks like the General Data Protection Regulation (GDPR) in Europe aim to protect individualโs privacy rights. However, compliance challenges arise, especially for companies employing extensive AI technologies. Adhering to ethical standards around data usage is not merely a legal obligation but a necessary practice for sustaining public trust in AI.
Accountability and Transparency
Accountability in AI refers to the responsibility of individuals and organizations that create and deploy AI systems. As AI technologies grow in complexity, establishing clear lines of accountability becomes paramount. Users often do not understand how AI systems reach their conclusions since many are
The Future Landscape of AI Technology
The future landscape of AI technology is not just an area of speculation; it is a crucial point of inquiry for various sectors. Understanding how AI will evolve helps companies, researchers, and policymakers to strategize effectively. The fast pace of change means that staying informed is essential for leveraging potential benefits while identifying upcoming challenges. As AI systems become more integrated into everyday life, comprehending their trajectory becomes increasingly important.
Potential Advancements
Next-Gen Machine Learning Models
Next-gen machine learning models are transforming the way data is processed and utilized. These models focus on more efficient algorithms that enhance predictive accuracy and analytical capabilities. One notable characteristic is their propensity for deep learning techniques that facilitate layer-by-layer processing of information. This feature allows for improved understanding of complex data sets. In this article, such models are significant for their ability to adapt to new findings with minimal human intervention, although they can require substantial computational resources.
Integration with Quantum Computing
Integration with quantum computing promises to revolutionize AI capabilities. Quantum computing introduces a unique ability to perform calculations at speeds exponentially faster than traditional computers. This characteristic enables AI systems to analyze vast amounts of data almost instantaneously. Consequently, applications in fields such as cryptography and complex simulations could become practical reality. However, the nascent stage of quantum technology presents challenges, including hardware availability and the necessity for new algorithms designed specifically for quantum systems.
Human-AI Collaboration
Human-AI collaboration highlights the synergy between human intelligence and machine learning capabilities. This relationship improves decision-making processes across various sectors. A key characteristic of this collaboration is the enhancement of user experience through tailored AI solutions. By observing patterns and learning from interactions, AI can support humans in their tasks. Nevertheless, dependency on AI tools may risk undervaluing human expertise if not managed carefully. This balance is necessary to avoid potential downsides.
Challenges to Overcome
Regulatory Hurdles
Regulatory hurdles are a critical aspect that can impede AI's development and application. As innovations unfold, legal frameworks struggle to keep pace, creating uncertainties for businesses and researchers alike. Notably, regulatory bodies must establish guidelines that protect users without stifling innovation. An effective regulatory framework should ensure accountability for AI systems while promoting ethical standards. Misalignment can cause skepticism around AI's reliability and its implications for society.
Technical Limitations
Technical limitations persist as a considerable barrier in achieving full potential in AI applications. Many AI systems still grapple with challenges such as insufficient data quality or constraints in computational power. These limitations lead to problems like model overfitting and less robust predictions. Addressing these issues fundamentally affects the field's growth. Ongoing research focuses on devising more adaptable algorithms, but these improvements take time and rigorous testing.
Public Perception and Trust
Public perception and trust play indispensable roles in the diffusion of AI technology. As the general populace encounters AI more frequently, their understanding and attitudes toward it evolve. Positive sentiment can lead to broader adoption and integration in various sectors. However, challenges remain with distrust resulting from fears surrounding job displacement and privacy issues. Increasing transparency and educating the public can help bridge this gap in trust, yet it requires concerted efforts from all stakeholders.
"Understanding the interplay between advancements and challenges in AI is vital for meaningful future applications."
Acknowledging both potential advancements and the challenges of AI allows a more nuanced perspective on where the technology is heading. AI stands at the intersection of possibility and caution, requiring informed discussions to harness its potential responsibly.