Exploring the Satori Knowledge Graph: An Overview
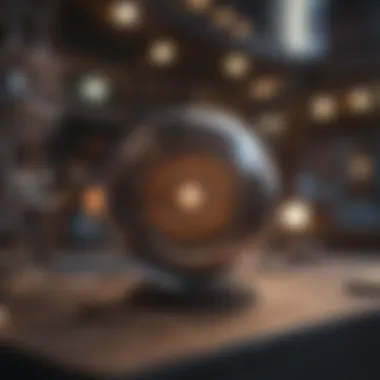
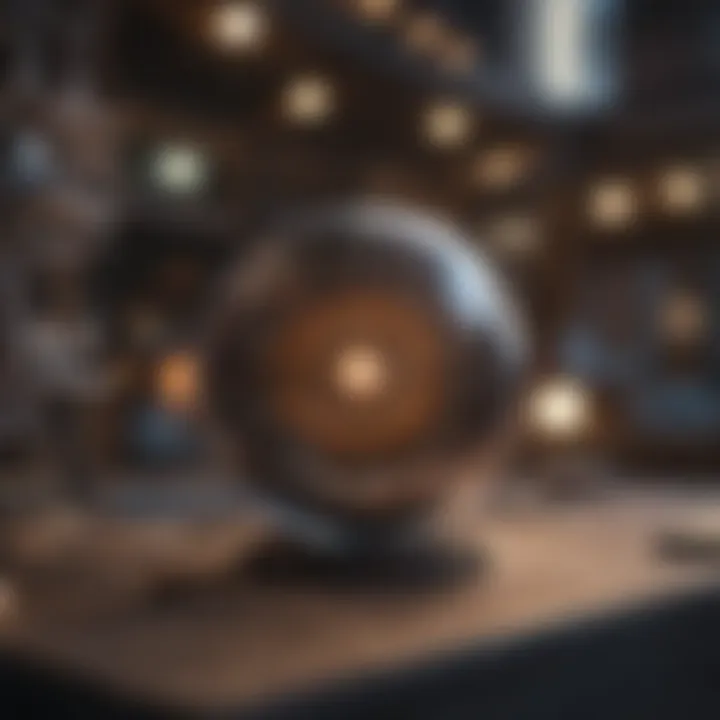
Intro
In the context of todayβs data-driven landscape, knowledge organization has never been more critical. The Satori Knowledge Graph emerges as a pivotal tool in enhancing knowledge retrieval systems. It symbolizes a synergy between vast datasets and intuitive frameworks that make accessing information more user-friendly. This comprehensive exploration will dissect the intricacies of the Satori Knowledge Graph by analyzing its architecture, functionalities, and role in advancing both scientific research and knowledge dissemination.
Through this inquiry, we aim to elucidate how the graph integrates data from various domains into a unified system that not only supports informed decision-making but also paves the way for transformative learning experiences.
Research Overview
Summary of Key Findings
The Satori Knowledge Graph showcases several critical advancements in the sphere of knowledge management. One of the principal findings is its capability to consolidate disparate data sources into a coherent structure. This allows for a more seamless interaction between users and the information they seek.
Secondly, the adaptability of the Satori Knowledge Graph in accommodating new data types has been noted. This ensures that users receive current and relevant insights. Moreover, its integration with artificial intelligence enhances the graph's ability to categorize and prioritize information, making retrieval not just easier but also smarter.
Relevance to Current Scientific Discussions
Since the inception of knowledge graphs, their role in enhancing machine learning and AI has been a topic of intense discussion among scholars and practitioners alike. The Satori Knowledge Graph emerges as a significant example that reflects these themes. It contributes to ongoing dialogues about data interoperability and knowledge sharing in research networks.
The implications of the Satori Knowledge Graph extend beyond theoretical frameworks. Its application in research and academia signifies a shift towards more organized, data-informed practices. As scholars grapple with the increasing volume of information, tools like the Satori Knowledge Graph are indispensable in navigating complexities.
Methodology
Research Design and Approach
This article adopts a qualitative approach to unpack the Satori Knowledge Graph's functionalities. The investigation centers on existing literature about knowledge graphs and how Satori fits within this broader context. Each facet of the graph's architecture is explored to understand its implications effectively.
Data Collection and Analysis Techniques
Data for this overview has been derived from multiple reputable sources, including journals and technical reports focusing on knowledge management and AI integration. Analyses were conducted to identify patterns and trends in the Satori Knowledge Graphβs usage and functionality. This triangulation of sources enhances the credibility and depth of the findings presented.
"The Satori Knowledge Graph epitomizes the future of knowledge organization, propelling disciplines towards a new era of informed decision-making."
Prelude to Knowledge Graphs
Knowledge graphs have emerged as vital tools for organizing and retrieving information, particularly in our data-driven world. They unify disparate data sources into a singular, navigable structure that reflects the relationships and contexts of knowledge. For this article, understanding knowledge graphs sets a strong foundation for exploring the Satori Knowledge Graph in detail. By detailing their core elements and advantages, we can appreciate how the Satori framework takes the concept further.
Definition and Purpose
A knowledge graph is a way of structuring information so that both humans and machines can easily access it. Typically, knowledge graphs represent entities, their attributes, and relationships in a graph format. Each node signifies a unique entity, while the edges connecting them illustrate how these entities relate to each other.
The purpose of a knowledge graph extends beyond mere information storage. It enhances semantic understanding, enabling advanced query capabilities and facilitating richer data discovery. This is particularly useful in domains such as artificial intelligence and scientific research, where interconnected information yields deeper insights.
The Evolution of Knowledge Graphs
The development of knowledge graphs traces back to the early days of artificial intelligence and semantic web technologies. Initially, information was organized in silos, making inter-domain research challenging. As technology progressed, the need for a more integrated understanding of information became clear.
The creation of schemas and ontologies marked the first significant advancement. These allowed data to be categorized and related more meaningfully. The advent of the internet then accelerated this evolution as vast amounts of information needed to be processed more intelligently.
Today, modern knowledge graphs like the Satori framework exemplify these advancements. They leverage improved algorithms for data integration, natural language processing, and machine learning. This has led to increased adoption across various sectors, including healthcare, finance, and education. Each new iteration aims to improve user experience, data accessibility, and collaborative research.
"Knowledge graphs represent a paradigm shift in how we organize and interact with information, transforming the way knowledge is disseminated and utilized."
In understanding the trajectory of knowledge graphs, we establish a crucial context for exploring specific implementations, such as Satori. By grasping their definition and evolution, we can better appreciate the intricate capabilities that modern knowledge graphs offer.
Understanding the Satori Knowledge Graph
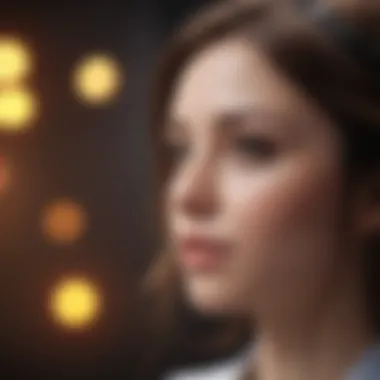
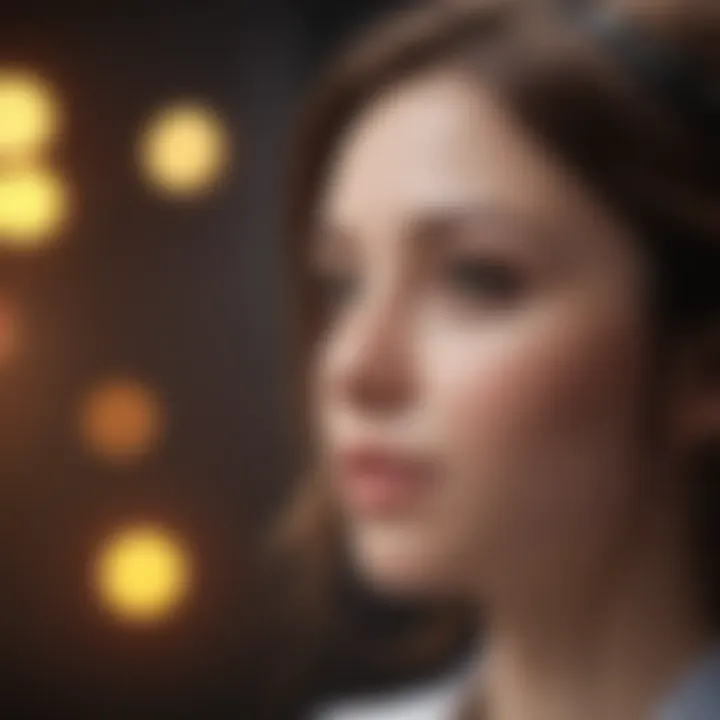
The Satori Knowledge Graph serves as a pivotal tool in the way knowledge is organized and utilized. Its significance lies in its ability to synthesize vast amounts of data from various sources into a structured representation of knowledge. This enhances comprehension and enables effective retrieval of information across a spectrum of domains. By dissecting the components and functionality of the Satori Knowledge Graph, one can appreciate its role in facilitating informed decision making and its utility in research frameworks.
Historical Context
To understand the Satori Knowledge Graph, a look back at its historical context is essential. The concept of knowledge graphs has evolved significantly over the past decade. Initially, traditional databases held structured information, but they lacked the capability to interlink diverse datasets effectively. The emergence of the Semantic Web introduced new methods for data representation, making way for knowledge graphs to gain traction.
The Satori Knowledge Graph is influenced by these developments. It extends beyond simple data storage by emphasizing the relationships between entities and concepts. This evolution is crucial because it underscores how such frameworks can support dynamic knowledge retrieval and understanding. For example, the transition from isolated data repositories to interconnected knowledge systems is a marking point for innovations like the Satori Knowledge Graph.
Core Components
The Satori Knowledge Graph consists of several core components that contribute to its functionality. These include entities, relationships, and attributes.
- Entities: These are the building blocks of the graph, representing distinct items such as people, organizations, or concepts.
- Relationships: They define how entities connect with each other. A well-defined relationship adds context to the data, making it more meaningful.
- Attributes: These provide additional details regarding entities and can include descriptions, categories, and various quantitative metrics.
Having a clear structure that integrates these components allows Satori to function optimally in retrieving and presenting data. With its interconnected structure, users can find relevant information quickly without wading through irrelevant data. Thus, this organization not only improves efficiency but also fosters deeper understanding.
Mechanisms of Functionality
Understanding how the Satori Knowledge Graph functions is vital in appreciating its application in various fields. The mechanisms that underpin its operation are focused on data integration, querying, and inference.
- Data Integration: The system amalgamates data from diverse sources, ensuring that the information is coherent and representative of various viewpoints. This reduces redundancy and inconsistencies.
- Querying: Users can perform queries that traverse relationships between entities, enabling nuanced discoveries. This capability is key for researchers looking to extract specific insights from large datasets.
- Inference: The knowledge graph can draw conclusions based on existing data connections, providing users with inferred knowledge that was not explicitly stated.
"Knowledge is not a static entity but a dynamic construct, constantly evolving with context and relationships."
Together, these mechanisms allow Satori to serve as a powerful framework for knowledge retrieval and management. As reliance on data-driven insights grows, understanding these functionalities becomes increasingly important.
The Technical Architecture of Satori
Understanding the technical architecture of the Satori Knowledge Graph is crucial for appreciating its functionality and versatility. This architecture serves as the backbone for handling vast amounts of data and ensuring that information is easily retrievable and applicable in various contexts. Key components of this architecture include the data models utilized and the integration of diverse data sources. Each of these elements plays a significant role in optimizing the performance and effectiveness of the knowledge graph.
Data Models Utilized
The data models used in the Satori Knowledge Graph are integral to its operational success. Primarily, the architecture employs both structured and unstructured data models.
- Structured Data Models: These models typically follow a predefined schema, organizing data into tables with rows and columns. This format facilitates straightforward querying and analysis.
- Unstructured Data Models: These models manage data that does not fit neatly into tables, such as texts, images, and videos. By utilizing advanced algorithms and natural language processing techniques, the Satori Knowledge Graph can extract meaningful information from this type of data.
The combination of these data models enhances Satori's adaptability and efficiency. By handling both types of data, the graph can support a broad array of applications, catering to the needs of researchers, educators, and professionals.
Integration of Data Sources
Effective integration of data sources is another pillar of the Satori Knowledge Graph's architecture. The ability to aggregate data from varied origins greatly enhances the knowledge graph's utility.
- Diverse Source Inclusion: Satori integrates data from academic journals, research databases, online repositories, and social media platforms. This diversity ensures a comprehensive knowledge base that is both relevant and current.
- Interoperability: The architecture supports interoperability, allowing different software systems to communicate and share data. This feature is essential for collaborative research and promotes a seamless flow of information among various stakeholders.
The integration process involves using specific protocols and standards to ensure that data is accurately combined without loss of integrity. As a result, users can access a unified view of information, which simplifies the data discovery process and aids in informed decision-making.
In summary, the technical architecture of the Satori Knowledge Graph not only supports advanced functionalities but also establishes a foundation for further developments in knowledge management and retrieval. By embracing a structured yet flexible approach to data modeling and integration, Satori stands out as a significant tool for promoting collaborative research and effective knowledge dissemination.
Applications in Scientific Research
The Satori Knowledge Graph plays a crucial role in the realm of scientific research. Its architecture fosters a systematic approach to knowledge organization, making it indispensable for researchers, educators, and professionals. The integration of diverse data sources creates an enriched environment for knowledge discovery, collaboration, and informed decision-making. In this section, we will discuss three key areas where the Satori Knowledge Graph demonstrates its significance in scientific research: facilitating data discovery, enhancing research collaboration, and supporting evidence-based decision-making.
Facilitating Data Discovery
In scientific research, uncovering relevant data can be challenging, particularly when dealing with vast amounts of information spread across multiple platforms. The Satori Knowledge Graph streamlines this process by providing a unified framework that draws connections between disparate datasets. This interconnectedness not only simplifies data retrieval but also empowers researchers to derive insights that may have previously gone unnoticed.
With Satori's advanced query capabilities, users can conduct in-depth searches to find data based on specific criteria. As a result, discovering new relationships and patterns becomes easier. This capability enables researchers to quickly locate datasets relevant to their studies, enhancing the efficiency of their work.
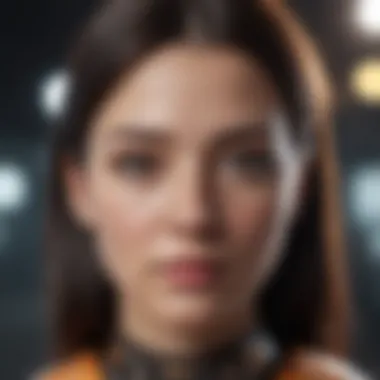
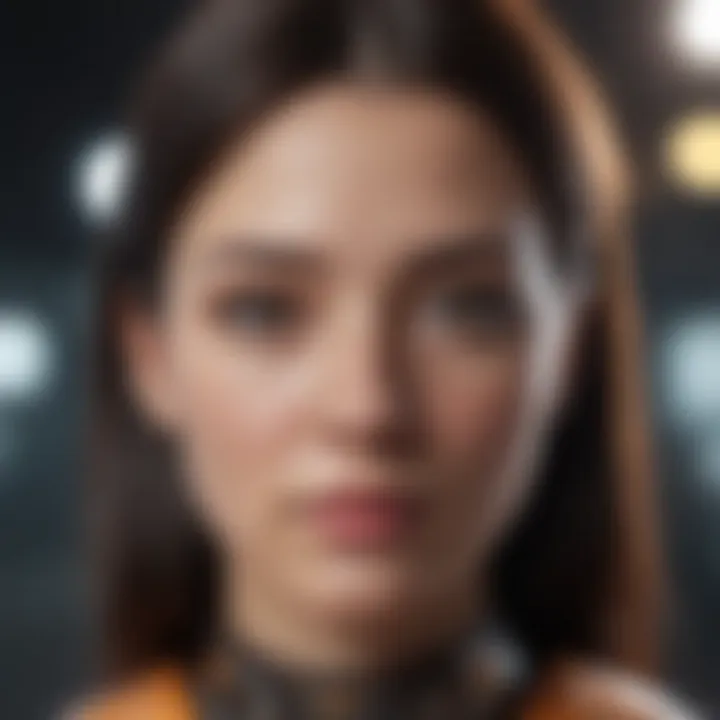
Enhancing Research Collaboration
Collaboration is a cornerstone of scientific advancement. The Satori Knowledge Graph facilitates collaborative efforts by allowing researchers to share findings and insights across disciplines. By integrating various sources of information, Satori provides a common platform where researchers can discover relevant work conducted by others.
The ability to easily access and share data fosters interdisciplinary dialogue. Researchers can build on each other's work, leading to innovative ideas and breakthroughs. In this atmosphere of shared knowledge, scientific communities become more connected. Ultimately, this enhances the pace of discovery and contributes to a more comprehensive understanding of complex research problems.
Supporting Evidence-Based Decision Making
Decision-making in scientific research often hinges on the availability of reliable data and insights. The Satori Knowledge Graph supports evidence-based decision-making by providing comprehensive access to nuanced information. Researchers can harness the graph to evaluate the quality and relevance of the data they encounter.
The interactive nature of the graph allows researchers to visualize data relationships, leading to more informed conclusions. As a result, researchers can align their hypotheses and experiments with established data, thus increasing the validity of their findings. Satori empowers researchers to make decisions based on a solid foundation of evidence rather than assumptions, ultimately strengthening the research process.
"Knowledge is power, and the Satori Knowledge Graph enhances this power by bridging the gap between data and insight".
Impact on Knowledge Dissemination
The Satori Knowledge Graph serves as a vital instrument in the modern landscape of knowledge dissemination. Understanding how information is shared and consumed is fundamental in todayβs information-rich environment. This section examines the mechanisms through which the Satori Knowledge Graph simplifies the process of knowledge dissemination, highlights its ability to bridge gaps in information, and emphasizes the significance of these functions for different stakeholders, including researchers and educators.
Simplifying Complex Information
One of the notable features of the Satori Knowledge Graph is its capability to simplify complex information. Research data can be intricate, often filled with jargon and dense figures. The Satori framework translates this complexity into more digestible formats. It does this by aggregating diverse datasets and offering a unified visual representation. This allows users to quickly grasp essential concepts without getting lost in details.
Through techniques like semantic search, the Satori Knowledge Graph enhances the relevance of search results. Users can query multi-layered data with simplicity and receive comprehensive insights tailored to their particular needs. This ultimately facilitates a smoother learning experience for students.
Additionally, the use of natural language processing (NLP) enables the Graph to interpret queries more naturally. The result is an interface that feels more intuitive and user-friendly.
Bridging Information Gaps
Another key function of the Satori Knowledge Graph is its role in bridging information gaps. In many research fields, silos of information can hinder collaboration and progress. Satori consolidates disparate sources, creating a cohesive body of knowledge. This merger is crucial in disciplines like biotechnology or environmental science, where inter-disciplinary research is paramount.
Furthermore, the Graph supports real-time updates. This ensures that users have access to the latest data and trends. For instance, researchers can view recent studies alongside historical data, offering a multidimensional view of their area of interest. It encourages a more holistic understanding, where stakeholders are not simply learning from one isolated stream of information but instead are exposed to a broader narrative that interconnects various insights.
"Knowledge, when shared wisely, can catalyze the pace of innovation. The Satori Knowledge Graph exemplifies this principle by minimizing barriers to information access."
Lastly, by enhancing data accessibility and visibility, the Satori Knowledge Graph empowers users to make informed decisions based on comprehensive insights. This fosters a culture of inquiry and drives forward thinking in research and education.
In summary, the Satori Knowledge Graph not only simplifies complex information but also bridges crucial gaps in knowledge dissemination. These factors contribute significantly to its impact on education and research, making it a vital tool for today's scholars and professionals.
Challenges and Limitations
Understanding the challenges and limitations of the Satori Knowledge Graph is essential for anyone engaging with its vast capabilities. While the graph offers innovative features, it also confronts obstacles that can hinder its efficiency and usability. This section examines two key problems: data quality and integration issues, alongside scalability and maintenance concerns. Each element plays a significant role in how effectively the Satori Knowledge Graph can operate and serve its users.
Data Quality and Integration Issues
Data quality is paramount in knowledge graphs. The Satori Knowledge Graph draws from multiple data sources, but the quality of this data can vary significantly. If inaccurate or outdated information is integrated, it can lead to misleading conclusions. For instance, flawed data can affect the reliability of research outcomes, thereby impacting decision-making processes.
Complicating this issue are integration challenges. Merging diverse data sets requires not only technical capabilities but also a deep understanding of different domains. Discrepancies in formats, standards, and terminologies can slow down the process of data integration. When data from widely varying sources is combined, maintaining accuracy in representation becomes a difficult task. Thus, ensuring both quality and seamless integration of data should remain a priority for developers and users of the Satori Knowledge Graph.
"Quality data is the backbone of effective knowledge representation and retrieval. Without it, the fundamental purpose of the graph comes into question."
Scalability and Maintenance Concerns
Scalability refers to the ability of the Satori Knowledge Graph to handle increasing amounts of data and user queries without loss of performance. As research and information needs expand, the Satori Knowledge Graph must evolve accordingly. A graph that cannot scale may become sluggish, error-prone, or even crash, especially when faced with large volumes of concurrent accesses.
Maintaining the system is equally crucial. As new data sources are added and existing ones are updated, continuous maintenance ensures that the graph remains relevant and efficient. However, frequent updates can introduce bugs or inconsistencies, which again loop back to the issue of data quality. Therefore, a robust maintenance strategy must be developed to regularly audit data integrity and system performance.
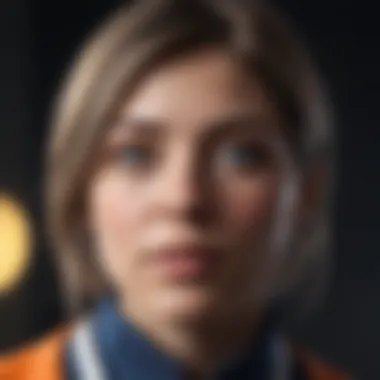
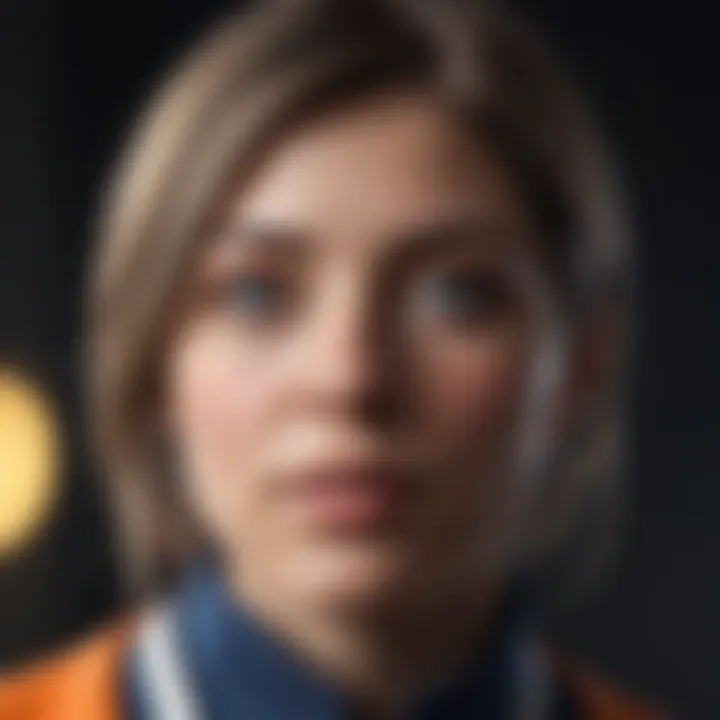
Future Prospects of the Satori Knowledge Graph
The future of the Satori Knowledge Graph is critical. As we progress into an era dominated by data-driven decisions, its continued evolution can have significant impact. This section explores anticipated developments focusing on technological advancements and enhancing user experience, which are paramount for ensuring that the Satori Knowledge Graph remains relevant and effective.
Technological Advancements
Technological advancements are essential for the Satori Knowledge Graph's future. Continued interoperability with various data sources is a core aspect of this development. The integration of machine learning algorithms can improve the graph's data accuracy and relevance. Such advancements create a more intelligent system that can learn from user interactions.
Through natural language processing, Satori can provide clearer insights. This capability will allow the graph to better understand the user's needs and produce more meaningful responses. Improved APIs can also facilitate access, making it easier for developers to create applications that utilize the Satori Knowledge Graph.
Moreover, blockchain technology might become an integral component. It can help establish data integrity and security, especially crucial in sensitive research areas. A future where Satori enhances collaboration across various fields and disciplines is promising.
Potential Enhancements to User Experience
Enhancing user experience is another focus area for the future of the Satori Knowledge Graph. A user-friendly interface is vital. Simplifying navigation through intuitive design can make complex information more accessible. In addition, personalized user experiences can be achieved through customizable dashboards, tailored suggestions, and improved search functionalities.
Real-time updates are also important. Users should rely on the Satori Knowledge Graph to reflect the most current data. This will enhance trust and reliability in the system.
Feedback mechanisms could further refine its performance. By allowing users to report issues or suggest improvements, Satori can evolve based on actual user experiences.
"User-centered design leads to greater engagement and trust in knowledge systems."
In summary, the future prospects of the Satori Knowledge Graph hinge on embracing technological advancements and enhancing user experience. These elements will ensure the graph's continued relevance and impact in knowledge management and research.
Closure
The future looks promising for the Satori Knowledge Graph. As advancements unfold, it's important to stay informed and engaged with these developments.
Comparative Analysis with Other Knowledge Graphs
The exploration of the Satori Knowledge Graph offers an opportunity to understand its place within a broader context of knowledge representation. In this section, we will examine the nuances of the Satori Knowledge Graph in contrast with other well-known knowledge graphs. Understanding these differences is crucial, as it informs how the Satori model can be utilized and what unique advantages it provides.
Differences in Design Philosophy
The design philosophy behind a knowledge graph significantly influences its performance and application. Satori stands out due to its emphasis on flexibility and adaptability. Unlike Google Knowledge Graph, which is predominantly tailored for search engine optimization and user engagement, Satori integrates data from various scientific domains, creating a comprehensive knowledge base aimed at researchers.
Popular knowledge graphs like Microsoftβs Azure Cosmos DB adopt a data-first approach, while Satori requires a more holistic perspective of knowledge. This means that while Satori is designed to connect and correlate fragmented knowledge across disciplines, systems like DBpedia focus on mapping structured information from Wikipedia without such extensive cross-domain integration.
This divergence in philosophy leads to different user experiences. Satori allows researchers to explore intricate relationships between data pieces, facilitating a more nuanced understanding of complex subjects. In contrast, others may prioritize quick access to factual information over in-depth exploration.
Use Cases Across Various Domains
The versatility of the Satori Knowledge Graph allows it to address a wide range of use cases across different domains. Here are a few prominent areas:
- Scientific Research: Satori enhances the discovery of relevant literature and data, and plays a critical role in systematic reviews. This functionality supports evidence-based decision-making.
- Healthcare: In medical fields, the ability to synthesize patient data with up-to-date research findings aids practitioners in providing informed medical advice.
- Education: The educational sphere benefits as Satori facilitates personalized learning experiences. Students can access curated resources tailored to their interests and academic needs.
- Artificial Intelligence: Satori feeds AI models with structured data, allowing for more accurate predictions and analyses in various sectors from finance to environmental studies.
Overall, the Satori Knowledge Graph's distinct approach and diverse use cases demonstrate its potential to redefine knowledge acquisition and utilization. By providing researchers, educators, and professionals with more profound insights, Satori remains a vital tool in the evolving landscape of information management.
"Understanding the Satori Knowledge Graph in relation to other models enriches our grasp of its innovative capabilities in knowledge curation."
The End
The conclusion of this article encapsulates the expansive exploration of the Satori Knowledge Graph, emphasizing its significant role in advancing knowledge organization and retrieval. The Satori Knowledge Graph's integration of diverse data sources provides a robust framework for enhancing understanding across various domains. Several key elements emerge from this discussion, revealing the various benefits and considerations inherent in the effective use of knowledge graphs.
Summary of Key Points
- Integration and Cohesion: The Satori Knowledge Graph successfully integrates varied data sources. This culminates in a cohesive structure that facilitates effective knowledge retrieval.
- Applications in Research: The architecture of the Satori Knowledge Graph supports scientific research significantly by enhancing data discovery, collaboration, and informed decision-making.
- Technical Architecture: Understanding its technical components, such as the data models and data source integration mechanisms, provides insight into its operational efficiency.
- Challenges Noted: Data quality, scalability, and maintenance are challenges that must be addressed to optimize the potential of the Satori Knowledge Graph.
- Future Prospects: The opportunities for further developments in technological realms and user experience highlight the ongoing evolution of knowledge graphs.
Final Thoughts on Future Developments
The future of the Satori Knowledge Graph is poised for evolution. As technological advancements make strides in artificial intelligence and data processing, the functionalities of the Satori Knowledge Graph may expand significantly. Researchers and technologists alike will need to focus on enhancing the user experience. Adopting intuitive interfaces can foster wider accessibility and engagement. Furthermore, the incorporation of more real-time data sources could enrich the quality and breadth of information represented. In summary, as the Satori Knowledge Graph continues to develop, its impact on knowledge dissemination and research collaboration will likely grow, benefiting students, researchers, educators, and professionals in numerous fields.
"The integration of diverse data within the Satori Knowledge Graph represents a pivotal advancement in knowledge management and retrieval." - Anonymous