Exploring the Impact of Medicine Datasets on Healthcare
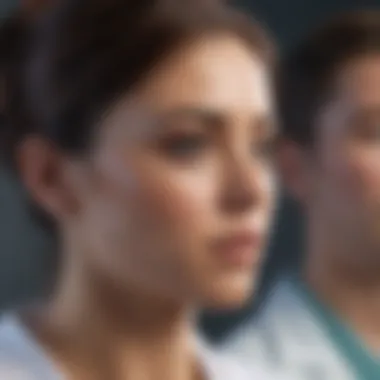
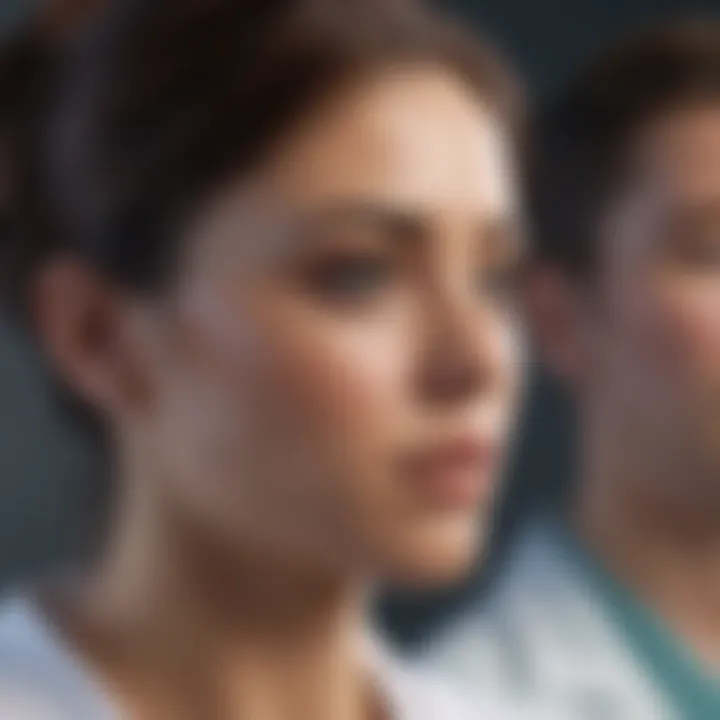
Intro
Medicine datasets have become a cornerstone of modern healthcare and biomedical research. The success of many medical endeavors depends on the quality and accessibility of these datasets. As more medical data becomes available, understanding these datasets is essential for students, researchers, educators, and professionals alike. This section serves as a foundation, offering insight into their importance and implications for the future.
Research Overview
Summary of Key Findings
The exploration into medicine datasets reveals several key findings:
- Diversity of Datasets: There are numerous types of datasets, including clinical trials data, electronic health records, genomics data, and epidemiological datasets. Each type serves a specific purpose and contributes to medical knowledge.
- Technological Integration: Technology plays a vital role in managing and analyzing datasets. Advanced algorithms and machine learning techniques enhance the capacity to derive meaningful insights from complex data.
- Ethical Considerations: The handling of medical datasets raises important ethical questions, particularly surrounding data privacy and consent. Researchers must navigate these challenges carefully to maintain public trust.
By highlighting these elements, it becomes clear that medicine datasets are integral to progressing medical field and improving patient outcomes.
Relevance to Current Scientific Discussions
The dialogue surrounding medicine datasets is more pertinent than ever. With the rise of big data in healthcare, experts now discuss the implications of massive datasets on research and clinical practices. Additionally, this focus includes:
- Interoperability: The ability to share and analyze datasets across platforms remains a hot topic. Standardization is crucial for effective data usage.
- Impact on Policy: As discoveries from data analysis lead to new treatments and improved public health strategies, policymakers must also adapt to these findings.
- Future Innovations: Ongoing advancements in data analytics and artificial intelligence continue to shape the conversation around medicine datasets. The future promises even greater opportunities for enhancements in healthcare delivery.
"Medicine datasets are not just collections of information; they are frameworks that guide the future of health and wellness."
Methodology
Research Design and Approach
To understand medicine datasets better, a systematic approach to research is necessary. This involves:
- Literature Review: Reviewing existing studies allows researchers to gather foundational knowledge about various dataset types and their implications.
- Case Studies: Analyzing specific examples of how datasets have influenced research findings offers valuable insights into effective methodologies.
Data Collection and Analysis Techniques
Collecting data accurately is paramount for any research project. Various techniques include:
- Surveys and Questionnaires: Gathering information directly from healthcare professionals and patients provides rich data for analysis.
- Observational Studies: These studies allow researchers to collect real-world data in natural settings, enhancing the validity of their findings.
- Statistical Analysis: Utilizing software for data analysis ensures that researchers can derive robust conclusions from their datasets.
In summary, a profound understanding of how medicine datasets function, their significance in healthcare, and the methodologies involved in their study is essential. This knowledge will guide future explorations and innovations in the medical field.
Prelude to Medicine Datasets
Medicine datasets represent a critical component in the landscape of healthcare and research. As the volume of data increases, understanding the nature and implications of these datasets becomes essential. They serve as the backbone for many advancements in medical science, offering insights that foster informed decision-making and improved patient outcomes.
In contemporary healthcare, the significance of medicine datasets extends beyond simple analytics. These datasets are integral in enabling healthcare professionals to assess treatment efficacy, design clinical trials, and implement public health interventions. By harnessing the available data, practitioners can derive actionable insights, which are crucial in enhancing overall healthcare delivery and outcomes.
Moreover, the effective management of medicine datasets presents various opportunities. Accurate data collection and analysis allow for tailored healthcare solutions, improving the quality of patient care. Additionally, with the rise of technology, innovative tools are emerging that enhance data usage, driving continual evolution in research methodologies.
Understanding medicine datasets also involves considering the ethical implications. Issues surrounding data privacy and informed consent are crucial, ensuring that sensitive information is handled responsibly. Addressing these concerns while capitalizing on the benefits of medicine datasets requires a delicate balance, which this article will thoroughly explore.
The objective of this section is to lay the groundwork for the subsequent discussions on the diverse types of medicine datasets, their collection methodologies, and the ethical considerations involved. Through this comprehensive guide, readers will gain insights into the fundamental aspects and relevance of medicine datasets in advancing healthcare and biomedical research.
Defining Medicine Datasets
Medicine datasets can be characterized as systematically collected and organized data directly related to health and medical needs. Empirical evidence, clinical records, surveys, and laboratory findings form the base of these datasets. They might include numeric data, text records, or imaging results, all capturing various facets of patient health, treatment protocols, and clinical outcomes.
The complexity of medicine datasets arises from their diversity. Different types of datasets cater to varying research needs. For instance, clinical trials data focus on evaluating new therapies, while electronic health records encompass comprehensive patient histories used for day-to-day healthcare decisions. The increasing interconnectivity of these datasets enhances their value as researchers and practitioners seek comprehensive views of patient care.
Historical Context and Evolution
The evolution of medicine datasets can be traced back to the earliest attempts at systematic observation in medicine. Initially, records were manually compiled in notebooks, focusing on anecdotal evidence. Over time, the need for more structure led to the development of various data collection methods and standards.
The introduction of electronic health records in the late 20th century marked a significant milestone. This shift allowed for quicker access and analysis of patient data, transforming how healthcare providers interact with medical information. Advanced statistical methods and computational tools later emerged, refining data analysis techniques and expanding research capabilities.
Recent years have seen an influx of new technologies, such as artificial intelligence and machine learning algorithms, gaining traction in processing and analyzing medicine datasets. This technological leap promises to further revolutionize the healthcare landscape by offering deeper insights and predictive analytics that were previously unattainable. The continuous evolution in data collection, management, and analysis reflects a broader trend towards a data-driven approach in yet-to-be fully realized ways.
Types of Medicine Datasets
Understanding the types of medicine datasets is vital for enhancing the field of healthcare research and practice. Each type serves distinct purposes and provides unique insights. Knowledge of these datasets aids in making informed decisions that can lead to better outcomes in patient care and medical innovations.
Clinical Trials Data
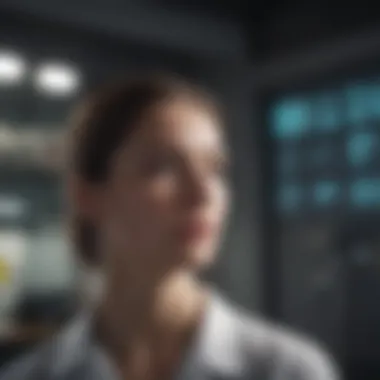
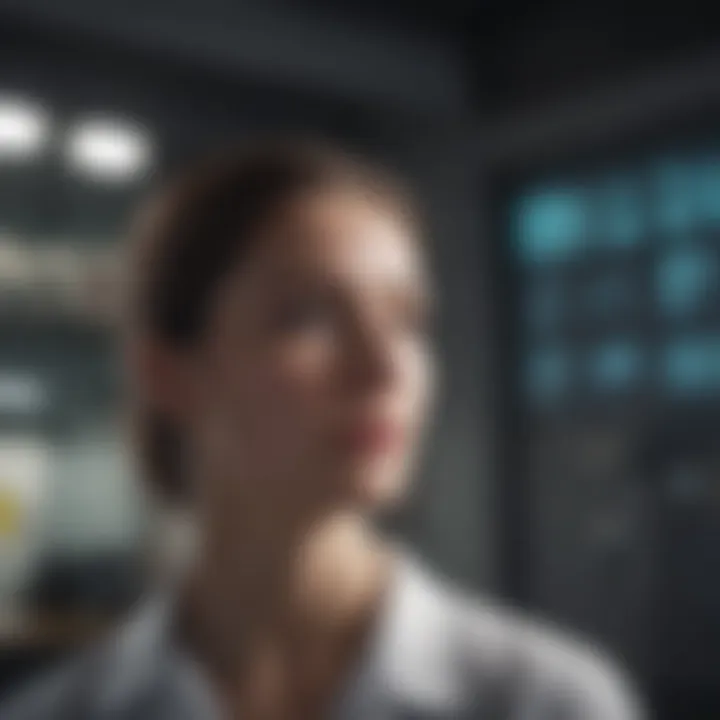
Clinical trials data is one of the most crucial components of medical research. It encompasses the information gathered during the various phases of clinical studies designed to evaluate the safety and effectiveness of new treatments, drugs, or medical devices. The rigorous nature of clinical trials ensures that the data collected follow standardized protocols, thus enhancing its reliability and validity.
The importance of clinical trials data extends beyond initial findings. It is critical for regulatory approvals from agencies such as the Food and Drug Administration (FDA). Moreover, this data provides a foundation for ongoing research, shaping guidelines for clinical practice and influencing healthcare policy decisions. The transparency in reporting trials ensures accountability and trust within the medical community.
Electronic Health Records
Electronic health records (EHRs) have transformed the way patient information is documented and accessed. EHRs contain comprehensive details about patients, including demographics, medical histories, medication lists, and laboratory results. These datasets enable healthcare providers to offer better care through improved coordination and communication among different services.
An essential benefit of EHRs is their ability to facilitate quick access to patient information, which can significantly reduce the chances of medical errors. Additionally, EHRs can support population health management by allowing for the analysis of broad datasets, revealing trends and patterns that can lead to improved public health strategies.
Genomic and Proteomic Data
Genomic and proteomic data represent the cutting edge of personalized medicine. These datasets involve the sequencing of DNA and the examination of proteins within an organism. Such information allows for a deeper understanding of diseases on a molecular level, paving the way for tailored therapies based on individual genetic profiles.
The investment in genomic sequencing has increased, enabling large-scale studies that can identify biomarkers for disease susceptibility and treatment effectiveness. As researchers analyze these vast datasets, there is potential for discovering new drug targets and innovative treatment options that can significantly enhance patient care and treatment outcomes.
Public Health Data
Public health data encompasses information collected from various sources that reflect the health status of populations. This data includes statistics on disease incidence, vaccination rates, and health behaviors. It serves as a vital resource for policymakers and public health officials in strategizing interventions and promoting health policies.
The significance of public health data lies in its ability to support decisions that can lead to improved community health outcomes. By analyzing trends within this data, authorities can identify at-risk populations and allocate resources effectively. Overall, public health data plays a crucial role in shaping health programs and policies that protect and improve public health.
Data Collection Methodologies
In the context of medicine datasets, the methodologies used for data collection are of paramount importance. They lay the foundation for the validity, reliability, and overall utility of the data. Effective data collection enables researchers to accurately understand health trends, evaluate treatment efficacy, and inform policy decisions. By adopting suitable methodologies, one can enhance the cohesiveness and comprehensiveness of medical research.
Survey and Questionnaire Design
Surveys and questionnaires are common tools for collecting data in medical research. They allow researchers to gather information on patient experiences, health behaviors, and treatment outcomes. When designing a survey, clarity and simplicity are key. Questions should be straightforward and avoid technical jargon that could confuse respondents.
- Question Types: Use a mix of open-ended and closed questions to allow for both qualitative and quantitative analysis. Closed questions can provide specific data points, while open-ended questions can yield richer insights.
- Pilot Testing: Before full deployment, pilot tests help identify any ambiguous questions or potential biases that might affect data quality.
- Demographic Considerations: Ensure that questions are appropriate for the target population. Demographics such as age, education level, and cultural background can impact responses and should be considered in the design phase.
Overall, careful survey design can significantly reduce bias and increase response rates.
Observational Studies
Observational studies offer another robust method for data collection. Unlike randomized control trials, these studies do not intervene; instead, they observe subjects in their natural environment. This method is particularly useful for studying behaviors, outcomes, and the effects of treatments when experimental designs are unethical or impractical.
- Types of Observational Studies: These can be categorized into cohort studies, case-control studies, and cross-sectional studies. Each type serves different research needs and has distinct advantages.
- Data Collection Techniques: In observational studies, data can be collected through direct observation, interviews, or existing records. Maintaining detailed notes and systematic approaches ensures that data is reliable.
Observational studies often provide insight into real-world conditions, making them invaluable in understanding complex health issues.
Sampling Techniques
Selection of appropriate sampling techniques is essential for ensuring that collected data accurately represents the broader population. The choice of sampling method can impact the generalizability of the research findings.
- Probability Sampling: This method gives each member of a population an equal chance of being selected, leading to more representative samples. Techniques include simple random sampling, stratified sampling, and cluster sampling.
- Non-Probability Sampling: In situations where probability sampling is not feasible, non-probability sampling methods can be used. This includes convenience sampling and purposive sampling, though these can introduce bias and limit generalizability.
- Sample Size Determination: Having a well-defined sample size is critical. Larger samples typically provide more accurate estimates of the population parameters; however, practical constraints like time and funding must be considered.
In summary, a thoughtful approach to data collection methodologies greatly enhances the quality and relevance of medical datasets, facilitating impactful research and informed decision-making.
Data Management in Medicine
In the complex landscape of healthcare and biomedical research, effective data management is crucial. It encompasses the strategies and practices that enable the collection, storage, retrieval, and analysis of vast amounts of data derived from various sources. Proper data management ensures that this information is not only accessible but also usable and trustworthy. With the increasing reliance on medicine datasets for decision-making and research, the significance of data management cannot be overstated. It is essential for improving patient care, facilitating accurate research outcomes, and maintaining compliance with regulatory demands.
Database Design and Development
The design and development of databases play a pivotal role in the management of medical data. An efficient database should be structured to accommodate diverse data types, including patient records, clinical trial results, and genomic information. Key considerations in this process include:
- Scalability: Databases must handle growing amounts of data without performance issues.
- Usability: The design should facilitate easy access and retrieval of data.
- Security: Protecting sensitive patient information is paramount. Implementing strong security protocols during development is critical.
Effective database design can streamline workflows and improve the accuracy of data retrieval, which directly impacts clinical decisions and research findings.
Data Storage Solutions
Selecting the appropriate data storage solution is a crucial aspect of data management in medicine. With various choices available, it's important to evaluate them based on specific needs and resource availability. Considerations might include:
- Cloud Storage: Offers scalability and flexibility. Services like Amazon Web Services or Google Cloud provide accessible platforms for storing large datasets.
- On-Premises Solutions: May offer more control and security but can come with higher costs and maintenance efforts.
Modern healthcare systems often adopt hybrid approaches, using both cloud and on-premises solutions. This helps balance flexibility, budget, and security concerns.
Data Analytics Tools
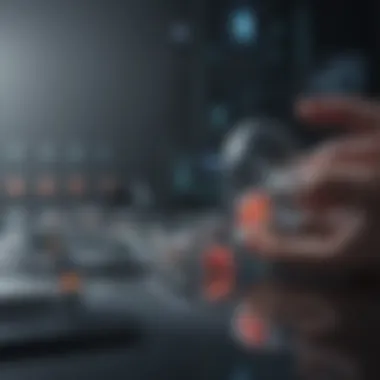
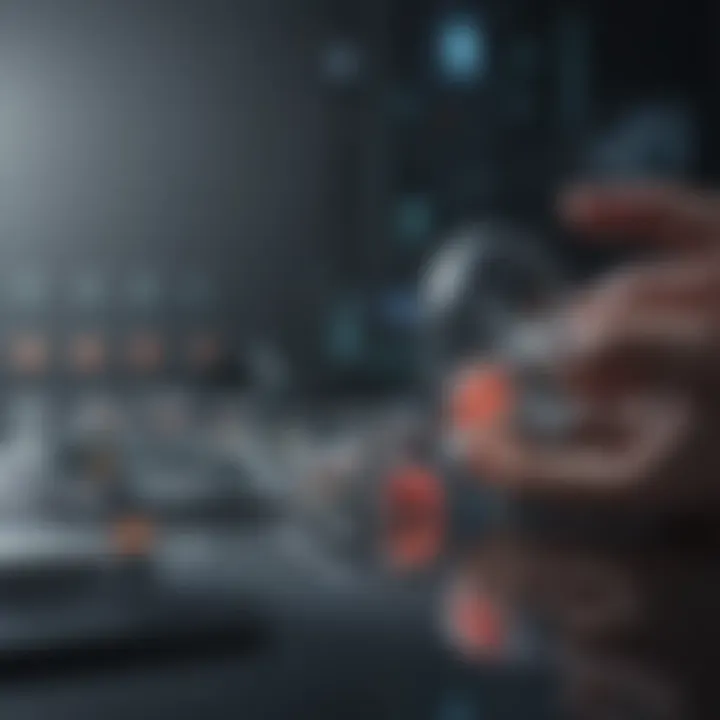
The application of data analytics tools is essential in extracting valuable insights from medicine datasets. These tools allow researchers and clinicians to identify trends, make predictions, and improve decision-making processes. Key functionalities to consider in analytics tools include:
- Statistical Analysis: Enable researchers to test hypotheses and validate findings.
- Machine Learning: Can help in predictive analytics, identifying patterns that may not be immediately apparent to human analysts.
- Visualization: Tools that provide graphical representations of data can enhance understanding and communication of complex findings to various stakeholders.
Investing in robust data analytics tools leads to improved outcomes in patient care and enhanced research capabilities, pushing the boundaries of medical knowledge.
Ethical Considerations in Using Medicine Datasets
The use of medicine datasets in healthcare and research raises important ethical issues. Understanding these considerations is vital for ensuring the responsible handling of sensitive information. Ethical implications touch on various aspects of data collection, usage, and sharing. These factors not only safeguard individual rights but also enhance trust within the medical community.
Informed Consent
Informed consent is a cornerstone of ethical research and data practices. It implies that participants understand the purpose, risks, and benefits of the study before agreeing to participate. Gathering informed consent is particularly crucial in health research that involves personal and sensitive data. Researchers must provide clear, concise information to participants, allowing them to make knowledgeable decisions. This process should also be documented thoroughly.
For instance, effective informed consent practices may include:
- Explaining the study's objectives clearly.
- Describing data collection methods in understandable terms.
- Allowing participants to ask questions and express concerns.
Failing to obtain informed consent can lead to ethical violations and damage the credibility of the research.
Data Privacy Protocols
Data privacy protocols are imperative in ensuring the confidentiality and security of sensitive healthcare information. As medicine datasets often include personal identifiers, the risk of privacy breaches is significant. It is essential to implement strong data protection measures.
These protocols can involve:
- Anonymizing data to remove identifiers.
- Limiting access to datasets only to authorized personnel.
- Compliance with regulations, such as GDPR or HIPAA, which provide guidelines for data protection.
Adhering to privacy standards not only safeguards individuals but also reinforces the credibility of the research initiatives.
Bias and Fairness in Data Usage
Bias in data usage can have profound effects on healthcare outcomes. It is essential to address potential biases inherent in datasets. Bias may arise from several sources, such as demographic disparities in data collection or unequal representation of various populations.
To ensure fairness, researchers must:
- Assess datasets for representation across demographics, including gender, age, and ethnicity.
- Apply analysis techniques that can identify and mitigate biases.
- Develop inclusive policies that ensure diverse participation in studies.
Promoting fairness in the analysis and application of data helps reduce health disparities and contributes to equitable healthcare solutions.
Ethical considerations are foundational to the effective use of medicine datasets. They shape research design, influence public trust, and ultimately affect patient outcomes.
The Role of Technology in Medicine Datasets
Technology plays a critical role in the effectiveness and efficiency of medicine datasets. In everyday medical practice and research, technology enables better data management, supports analytical methods, and enhances data sharing. The evolution of technology has led to more precise collection, storage, and analysis of health-related data. From Artificial Intelligence to blockchain innovations, these technological advancements provide new tools to address existing challenges in data utilization.
Artificial Intelligence and Machine Learning
Artificial Intelligence (AI) and Machine Learning (ML) have transformed the landscape of data analysis in medicine. Utilizing vast amounts of datasets, AI algorithms can discover patterns that human analysts might miss. This capability is particularly significant in predicting patient outcomes and personalized medicine.
Moreover, AI applications improve efficiency in clinical decision support systems. They help in diagnosing diseases by analyzing patient records faster than traditional methods. For example, AI can assess radiographic images to identify anomalies, which assists radiologists in their work.
However, the adoption of these technologies brings challenges. Data used for training models needs to be diverse to avoid bias and ensure reliable outputs. Therefore, researchers must focus on gathering comprehensive datasets that reflect varied populations.
Blockchain for Data Integrity
Blockchain technology offers robust solutions to data integrity concerns in medicine datasets. By creating a decentralized ledger, blockchain ensures that all entries are tamper-proof and traceable. This characteristic is vital when maintaining the confidentiality and authenticity of patient records.
For instance, if a medical practitioner updates a patientβs file, the changes are recorded in a way that is both visible and immutable. This ensures accountability among healthcare providers. Furthermore, patients can have more control over who accesses their medical data, fostering trust in the system.
Nevertheless, implementing blockchain in healthcare poses difficulties such as scalability and interoperability with existing systems. Stakeholders must navigate these challenges to fully realize the benefits of blockchain technology.
Cloud Computing in Medical Research
Cloud computing has revolutionized the storage and analysis of medicine datasets. It enables researchers and healthcare providers to access data from anywhere at any time. This accessibility is particularly useful for collaborative efforts across institutions.
With cloud solutions, there is no need for maintaining extensive physical storage systems. This leads to cost savings and efficient resource use. Additionally, the ability to process large datasets on cloud platforms enhances research capabilities. Researchers can run complex analyses much faster than with traditional computing methods.
It's important to note that cloud solutions must comply with regulations like HIPAA to protect sensitive health information. Security measures should also be implemented to safeguard data against breaches.
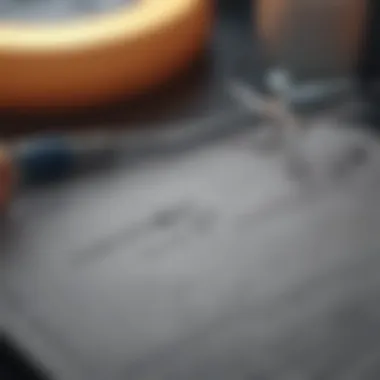
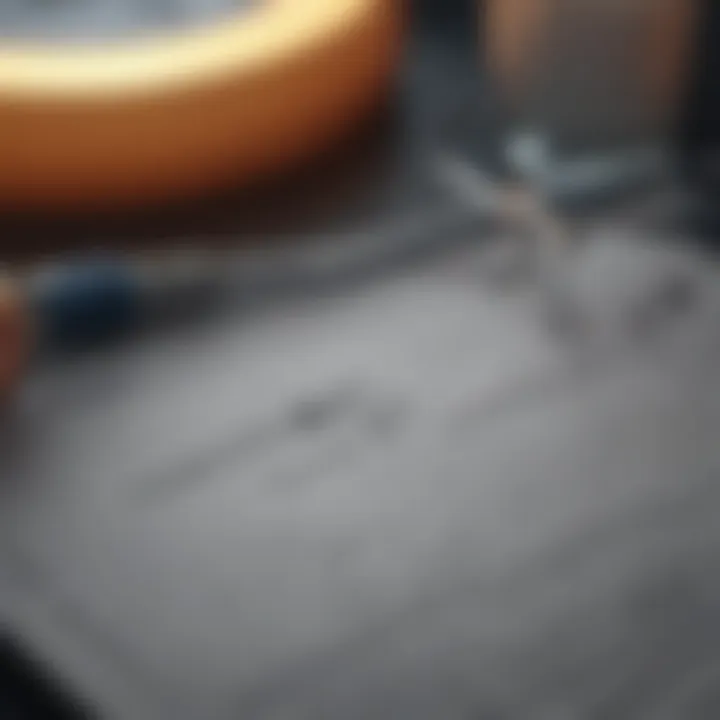
"Technology in medicine datasets is not merely an aid; it's essential for advancing healthcare outcomes and practices."
Impact of Medicine Datasets on Healthcare
Medicine datasets have a profound impact on healthcare systems, driving numerous improvements in patient care, medical research, and public health strategies. The analytical abilities and insights gained from these datasets allow healthcare professionals to make informed decisions that enhance patient outcomes and optimize resource allocation. It also enables researchers to uncover new trends and develop innovative treatments based on evidence collected through varied sources. Overall, the integration of medicine datasets fundamentally reshapes how healthcare operates and evolves.
Improving Patient Care
Improving patient care is one of the most significant advantages derived from the use of medicine datasets. Electronic Health Records (EHRs), for instance, provide comprehensive and organized patient information that helps medical teams track health trends over time. The availability of accurate data enables healthcare providers to personalize treatments according to individual patient histories and needs.
Additionally, predictive analytics can identify at-risk patients, allowing for early intervention. When healthcare professionals can anticipate issues before they arise, they can develop proactive care plans, which improves patient safety and reduces hospital admissions. This tailored approach leads to higher patient satisfaction and better health outcomes.
Facilitating Medical Research
Facilitating medical research stands out as another critical aspect of medicine datasets. Researchers use vast amounts of data to explore correlations between treatment efficacy and patient demographics. This research can help identify effective therapeutic methods and streamline clinical trials. By analyzing datasets from previous studies, researchers can avoid redundant efforts, saving time and resources.
Furthermore, collaborative platforms using shared datasets enhance transparency and reproducibility in research. Access to diverse datasets promotes inclusivity in clinical studies, thereby ensuring that findings are applicable across varied populations. Thus, medicine datasets play a vital role in advancing scientific knowledge and healthcare solutions.
Public Health Surveillance
Public health surveillance is a vital area significantly bolstered by the analysis of medicine datasets. These datasets allow public health officials and epidemiologists to monitor disease outbreaks in real-time. By assessing patterns in population health, officials can issue alerts about potential threats, such as infectious disease outbreaks or environmental hazards.
Moreover, these insights guide public health interventions. Strategies that promote vaccination, health education, or resource allocation can be informed by data-driven insights. In this way, medicine datasets serve as essential tools in shaping effective public health policies and improving community health on a broader scale.
"The effective use of medicine datasets leads not only to enhanced individual patient care but also strengthens the overall healthcare framework by fostering informed public health decisions."
In summary, the impact of medicine datasets on healthcare spans various dimensions, all of which contribute to enhancing care, accelerating research, and fortifying public health measures. This holistic utilization of data presents powerful opportunities for continuous improvement within the healthcare landscape.
Challenges Facing Medicine Datasets
The effective use of medicine datasets is paramount in advancing healthcare and research. However, several challenges impede their optimal utilization. Recognizing and addressing these challenges is crucial for professionals involved in data management and healthcare innovation.
Data Standardization Issues
Data standardization plays a critical role in ensuring compatibility and reliability across various datasets. Inconsistent data formats can lead to misinterpretations or incorrect conclusions in research and treatment. For example, if one dataset records blood pressure as mmHg while another uses kPa, integrating these datasets becomes problematic. This can result in significant barriers to effective analysis.
Moreover, a lack of universally accepted terminology in healthcare can contribute to data standardization issues. Different medical institutions may adopt varied terms for the same condition, complicating data interoperability. Addressing this requires a collaborative effort from regulatory bodies, healthcare institutions, and data managers to establish common standards.
Data Quality and Integrity Concerns
Data quality is essential for producing reliable research results. Poor data quality, whether from incorrect entries or missing information, can undermine the validity of medical studies. High-quality datasets should prioritize accuracy, completeness, and relevance.
In many instances, the integrity of datasets can be jeopardized by malicious actions such as data breaches or tampering. For healthcare datasets, even small inaccuracies can have serious implications for patient care. Measures to protect data integrity must include robust security protocols and regular audits. Educating staff about data management best practices is also an invaluable part of ensuring data quality and integrity.
Regulatory Compliance
Adherence to regulations is a significant challenge in the landscape of medicine datasets. Laws such as the Health Insurance Portability and Accountability Act (HIPAA) in the United States establish stringent guidelines for data privacy and security. Failing to comply with these regulations can result in severe penalties and harm to a healthcare institution's reputation.
Compliance is especially challenging in multinational research, where differing regulations across countries come into play. Understanding the requirements in each jurisdiction becomes essential for researchers and data managers. Organizations must create comprehensive compliance strategies that include effective monitoring and training to ensure that all personnel understand the legal landscape surrounding data use.
"The path to effective healthcare data management is often obstructed by challenges that require careful navigation and expertise."
In summary, addressing challenges like data standardization, quality and integrity concerns, and regulatory compliance is vital for the successful utilization of medicine datasets. Tackling these issues not only helps in enhancing the reliability of data but also promotes better healthcare outcomes.
Future Directions for Medicine Datasets
The future of medicine datasets is on the brink of transformative change. As the healthcare landscape becomes more data-driven, understanding the trends and future directions in this field is essential. Medicine datasets will increasingly shape clinical decisions, public health strategies, and personalized medicine approaches. The relevance of exploring emerging trends, interoperability, and advancements in sharing policies cannot be overstated. These elements not only improve efficiency but also enhance patient outcomes.
Emerging Trends in Data Utilization
As technology evolves, we witness various trends in data utilization within the medical field. One significant trend is the integration of comprehensive datasets that include social determinants of health. These factors play a critical role in overall health outcomes. By incorporating this type of data, healthcare providers can achieve a more holistic understanding of patient needs.
Additionally, the utilization of real-time data analytics is becoming more common. This can enable immediate decision-making in clinical environments, thus potentially improving patient care. Furthermore, the incorporation of wearables and remote monitoring devices adds another layer of data collection. This shift allows patient data to be collected continuously, offering clearer insights into their health status.
Interoperability of Healthcare Systems
Interoperability is a vital aspect of medical datasets. It refers to the ability of different healthcare systems to communicate and share data seamlessly. Achieving interoperability can lead to significant benefits, including reduced duplication of services and improved care coordination.
However, many healthcare systems still operate in silos, which hinders the flow of information. Moving toward standardized data formats and protocols is crucial. A commitment from multiple stakeholders, including providers and policymakers, will foster an interoperable future. This can enable easier access to patient records across various platforms, ultimately improving healthcare delivery.
Advancements in Data Sharing Policies
The concept of data sharing in healthcare has gained momentum. As more datasets become available, advancements in data sharing policies are crucial. This includes developing frameworks that ensure data is shared ethically and responsibly, while maintaining patient privacy.
Regulatory measures that support data sharing can facilitate collaboration among researchers. This can lead to more comprehensive studies and enhanced innovation in medical practices. Furthermore, organizations are increasingly looking toward decentralized data sharing models. These allow data ownership to remain with the individuals while sharing insights for research and public health.
"With responsible data sharing, we can harness the power of collaborative research and drive advancements in medicine."