Galaxy RNA Sequencing Analysis: Techniques and Applications
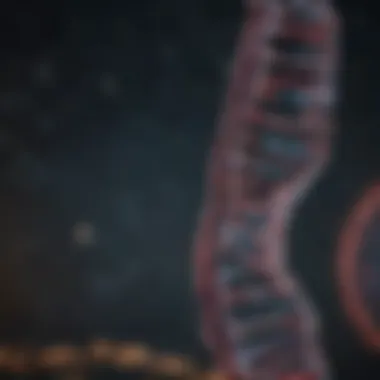
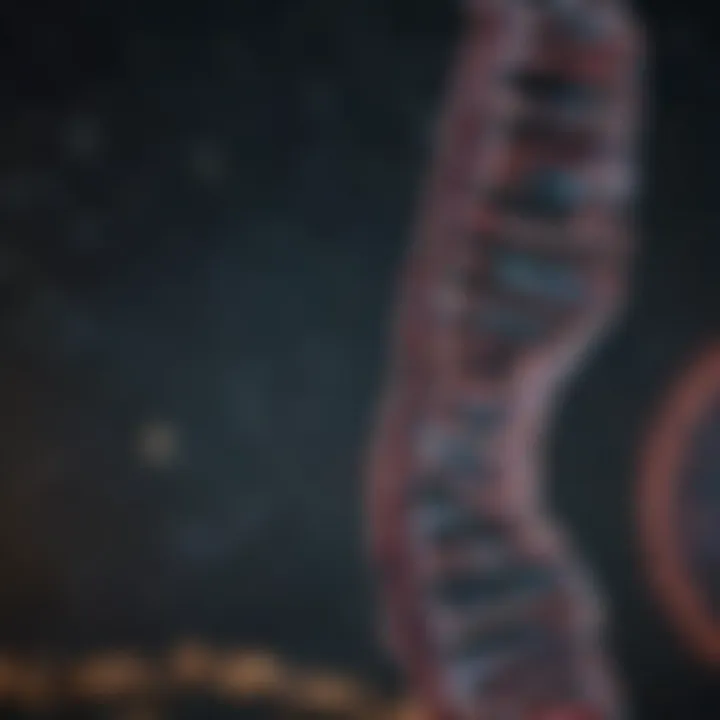
Intro
RNA sequencing has transformed how researchers investigate gene expression. Among the various platforms available, Galaxy stands out for its intuitive design, making it accessible for users without extensive bioinformatics training. The ability of Galaxy to streamline complex workflows allows scientists to focus more on interpreting results rather than wrestling with technical challenges. This article aims to dissect Galaxy RNA sequencing analysis, covering its methods, applications, and significance in genomic research.
Research Overview
Understanding the capabilities of Galaxy RNA sequencing is vital for contemporary research.
Summary of Key Findings
Recent studies illustrate that Galaxy not only simplifies RNA sequencing analysis but also enhances reproducibility and collaboration among researchers. Key findings suggest that utilizing Galaxy can lead to more accurate data interpretation.
The user-friendly interface is pivotal. It allows users to visualize their data, making it easier to draw insights from raw sequences. Its integration with various compatible tools expands its utility, facilitating workflows across different scientific domains.
Relevance to Current Scientific Discussions
As genomic studies expand, RNA sequencing plays a crucial role in various fields, from understanding basic biological processes to personalized medicine. The ongoing discussions on best practices in RNA sequencing underscore the necessity for platforms like Galaxy, which can adapt to emerging needs and technologies.
Methodology
An effective methodology is central to achieving reliable outcomes in RNA sequencing analysis. Understanding Galaxy's approach helps in comprehending its utility in research.
Research Design and Approach
The design of RNA sequencing studies using Galaxy involves multiple steps. Initially, raw sequencing data is uploaded and processed through a series of tools available on the platform. Galaxy implements a workflow-based design that allows users to customize their analyses according to specific research goals.
- Uploading Data: Researchers input their RNA sequencing data in FASTQ format.
- Quality Control: Quality assessments are performed using tools like FastQC, providing insights into data integrity.
- Alignment: The RNA sequences are aligned to a reference genome using software like Hisat2 or STAR.
- Quantification: Tools such as featureCounts are used to quantify gene expression levels.
Data Collection and Analysis Techniques
Data collection in Galaxy is straightforward due to its structured and modular nature. Users can select different analysis components based on their analytical needs. After establishing the workflow, results are generated and can be further examined.
Analysis techniques often include statistical assessments of gene expression data. Differential expression analysis can be performed using DESeq2 or EdgeR, providing insights into gene behavior across conditions.
Preface to RNA Sequencing
RNA sequencing, or RNA-Seq, represents a powerful technique fundamental to modern genomics. This method involves sequencing cDNA generated from a sample's RNA, producing a comprehensive view of the transcriptome. The importance of RNA sequencing lies not just in its ability to quantify gene expression, but also in its capacity to uncover alternative splicing, gene fusions, and novel transcripts. In this article, we will delve into the nuances of RNA sequencing to illustrate its critical role in advancing genomic research and biotechnology.
RNA sequencing allows researchers to explore the complexity of the transcriptome with unprecedented depth. The information gathered can lead to significant insights into gene function, regulation, and interaction within biological networks. Furthermore, the advent of high-throughput sequencing technologies has enabled quick and massive data generation, facilitating a broader scope of investigation. Researchers can now analyze samples across diverse conditions and treatments, leading to a more thorough understanding of biological processes. The benefits of RNA sequencing are vast, encompassing basic research, clinical diagnostics, and therapeutic development.
However, it is essential to recognize the considerations associated with RNA sequencing. While the data obtained can be immensely informative, the analysis process is complex, involving various steps ranging from sample preparation to data interpretation. Challenges include ensuring data quality, dealing with large volumes of information, and addressing reproducibility issues. Given these factors, a systematic approach through an intuitive platform like Galaxy can significantly enhance the RNA sequencing analysis, making it accessible even to those with limited computational expertise.
In this article, we will cover critical aspects related to RNA sequencing, including its definition, historical context, and the various applications it supports. By establishing a clear understanding of RNA sequencing and its significance, we aim to highlight its pivotal role in genomics as well as in clinical practices.
Understanding the Galaxy Framework
Understanding the Galaxy Framework is pivotal to grasping the full potential of RNA sequencing analysis. Galaxy serves as an open-source platform that allows researchers to conduct complex analysis workflows without the need for programming expertise. As bioinformatics becomes more integral to biological research, the importance of accessible, user-friendly tools cannot be overstated. Galaxy meets this need by providing a visual interface for data workflows. This lowers the barrier of entry for researchers from various backgrounds.
The versatility of Galaxy is one of its most significant benefits. It supports a wide array of tools and resources, facilitating not just RNA sequencing but a multitude of genomics applications. Furthermore, the collaborative nature of the Galaxy platform allows users to share tools and workflows easily, fostering community and knowledge exchange.
Another essential consideration is the way Galaxy integrates with existing data ecosystems. Researchers can import and export data from various formats, ensuring seamless interaction with diverse genomic datasets. This flexibility enhances data management efficiency, an often underappreciated aspect of genomic research. At its core, the Galaxy framework empowers researchers to focus on their hypotheses and results, rather than getting lost in the technical complexities of data analysis.
Overview of Galaxy
Galaxy's primary function is to enable users to perform computational biology without needing in-depth programming skills. This web-based interface allows for the easy selection and integration of various bioinformatics tools. Users can build custom workflows tailored to their specific needs. Essentially, Galaxy democratizes bioinformatics by making advanced techniques available to all researchers.
The platform is built on a framework that accommodates a wide range of bioinformatics methodologies. Users can access tools for data pre-processing, analysis, and visualization. This accessibility helps bridge the gap between computational science and biological inquiry.
Core Features and Tools
Several core features distinguish Galaxy from its peers:
- Workflow Management: Galaxy allows researchers to create, edit, and share workflows visually. This feature simplifies the process of designing analysis pipelines, making them reproducible and adjustable as research needs evolve.
- Extensive Tool Repository: The platform integrates numerous tools for various bioinformatics tasks, including RNA-Seq analysis, variant calling, and metagenomic analysis. Users can browse and select tools based on their specific experimental design.
- Data Integration: Galaxy supports the integration of different data types, facilitating comprehensive analyses across multiple datasets. This feature is crucial for multivariate studies, where multiple sources of data yield richer insights.
- Result Visualization: The ability to visualize results within Galaxy is a significant benefit. Users can generate plots, graphs, and other visual outputs to interpret their data effectively.
- Community Collaboration: The Galaxy community is robust, offering support and sharing valuable resources. By connecting users worldwide, Galaxy fosters an environment where best practices for RNA sequencing analysis can flourish.
Understanding these elements equips researchers to leverage the Galaxy platform effectively. A solid grasp of the Galaxy framework ultimately enhances the reliability and robustness of RNA sequencing studies.
Importance of RNA Sequencing Analysis
RNA sequencing analysis has emerged as a pivotal tool in the field of genomics, impacting various scientific disciplines. Understanding this importance rests on several key pillars, including technological advances, diverse applications in research, and significant clinical implications.
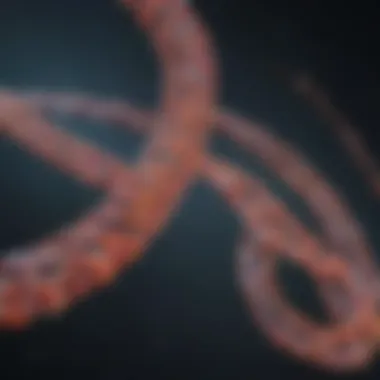
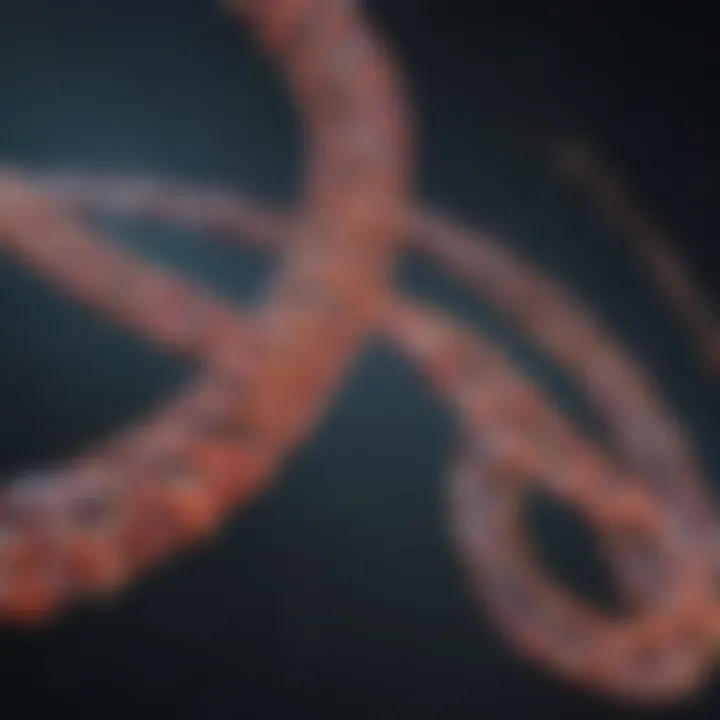
Advancements in Genomics
RNA sequencing is not merely a method of data collection; it represents a leap forward in our ability to comprehend the complexity of the transcriptome. Through precise quantification of RNA molecules, researchers can identify gene expression patterns that were previously challenging to detect. This technology enables an unprecedented depth of understanding regarding how genes are regulated and expressed under various conditions. A few advancements include:
- High-throughput techniques that generate massive amounts of data efficiently.
- Single-cell sequencing, allowing for more nuanced insights into cellular heterogeneity.
- Enhanced computational tools that improve data analysis and interpretation, increasing reproducibility and reliability of results.
These advancements are crucial for advancing research in genetics, evolution, and many other biological sciences.
Applications in Research
The versatility of RNA sequencing makes it applicable across various fields of research. This includes but is not limited to:
- Basic Biology: Understanding cellular processes and signaling pathways.
- Ecology: Studying how organisms adapt to changes in their environment.
- Agriculture: Enhancing crop varieties through functional genomics.
- Microbiology: Analyzing microbial communities and their interactions in ecosystems.
The increasing breadth of applications highlights the essentiality of RNA sequencing analysis as a fundamental research tool that drives innovation and discovery.
Clinical Implications
RNA sequencing also holds substantial clinical relevance, giving rise to personalized medicine approaches. By analyzing RNA profiles from patients, healthcare professionals can tailor treatments based on individual genetic backgrounds. This capability leads to several critical outcomes:
- Identification of biomarkers for disease detection and prognosis.
- Understanding the mechanisms of disease at a molecular level.
- Facilitating drug discovery by pinpointing therapeutic targets.
These clinical implications underscore the broader impact of RNA sequencing on healthcare, making it a key area for investment and research in the coming years.
RNA sequencing is redefining our understanding of biology and medicine, making it indispensable in contemporary research and clinical settings.
Overall, the importance of RNA sequencing analysis cannot be overstated. It fuels advancements in knowledge, supports diverse research applications, and enhances clinical practices, solidifying its role as an essential aspect of modern science.
Galaxy RNA Seq Analysis Workflow
The workflow of Galaxy RNA sequencing analysis is crucial to ensuring that the data is processed effectively. This step-by-step approach facilitates seamless handling and interpretation of RNA-Seq data, emphasizing reliability and reproducibility. Given the complexity and volume of genomic data today, adopting a systematic workflow not only maximizes the strengths of the Galaxy platform but also enhances user experience. Researchers benefit from Galaxy's interactive tools that streamline processes. Understanding this workflow is essential for students, researchers, and educators alike.
Data Input and Preparation
Data input and preparation are the foundational steps in the Galaxy RNA sequencing analysis workflow. This stage involves collecting raw sequence data from various sources, such as NGS platforms like Illumina and PacBio. Once the data is managed, it is typically in the form of FASTQ files, which contain the sequence information along with quality scores. Preparing the data includes several critical tasks:
- File Organization: Structuring files in a recognizable manner promotes efficiency.
- Metadata Documentation: Recording relevant metadata ensures traceability and better understanding of the experiment.
- Trimming and Filtering: Removing low-quality reads and trimming sequences to standard lengths are basic practices to enhance data quality before analysis. This prepares the input for downstream processes.
Processing RNA-Seq Data
Processing RNA-Seq data in Galaxy involves several tools that transform raw sequences into meaningful biological insights. The Galaxy platform offers a range of workflows and applications to manage this transformation effectively. Key steps in processing RNA-Seq data may include:
- Alignment: Mapping reads to a reference genome or transcriptome is essential. Tools like TopHat or STAR could be utilized to perform this task with high accuracy.
- Quantification: After alignment, the next step is quantifying the expression levels of genes. Using tools like Cufflinks can provide estimates of gene abundance.
- Differential Expression Analysis: Identifying genes that exhibit significant changes in expression is vital in many studies. This can be facilitated through packages such as DESeq or EdgeR, available within Galaxy.
Quality Control Measures
Quality control is a necessary step in any RNA sequencing analysis. It helps to assess the data quality and ensure that reliable results are produced. Within the Galaxy framework, several quality control measures can be implemented:
- Raw Data Assessment: Initial examination of raw FASTQ files using tools such as FastQC can reveal potential issues, including unexpected sequencing artifacts.
- Post-Alignment Quality Checks: After mapping, it is important to conduct checks for read distribution, coverage depth, and potential biases. Tools in Galaxy can visualize these aspects effectively.
- Filtering Criteria: Setting stringent criteria for filtering out poor-quality data is fundamental. Removing outliers and ensuring data integrity is necessary to guarantee accurate downstream analysis.
The emphasis on quality control not only fortifies the reliability of findings but also enhances the credibility of subsequent interpretations and conclusions.
Data Analysis Techniques in Galaxy
Within the context of RNA sequencing, the data analysis techniques employed in the Galaxy framework are pivotal. They enable researchers to extract meaningful insights from large and complex datasets. Two primary techniques that warrant attention are Differential Gene Expression Analysis and Gene Set Enrichment Analysis. Both techniques serve distinct purposes but together contribute significantly to the broader understanding of gene expression patterns in biological systems.
Differential Gene Expression Analysis
Differential Gene Expression Analysis (DGEA) is a fundamental tool used to assess the changes in gene expression levels between different conditions or groups. This technique is crucial for identifying genes that are overexpressed or underexpressed in a particular sample. In the context of Galaxy, DGEA is advantageous due to its user-friendly interface which allows researchers to perform complex analyses without extensive programming skills.
The primary steps in DGEA include:
- Normalization of Data: This involves adjusting the raw sequencing counts to account for variations in sequencing depth and other factors. Normalized data provides a more accurate representation of true biological differences.
- Statistical Testing: Various statistical methods, such as the t-test or ANOVA, are applied to determine whether the differences in expression levels are statistically significant.
- Interpretation of Results: The results can be visualized using various plots, helping researchers to comprehend the significance of their findings.
Key benefits of DGEA in Galaxy include:
- Accessibility: Researchers at all levels can easily navigate the Galaxy platform, making DGEA approachable even for beginners.
- Integration: DGEA can be seamlessly integrated with other analysis workflows within Galaxy, facilitating comprehensive data exploration.
- Flexibility: The platform supports various statistical methodologies, allowing researchers to choose the most appropriate approach for their data.
"The power of Differential Gene Expression Analysis lies in its ability to unveil the biological significance of RNA sequencing data, making it a cornerstone of genomic research."
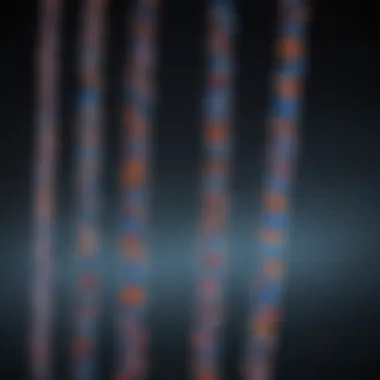
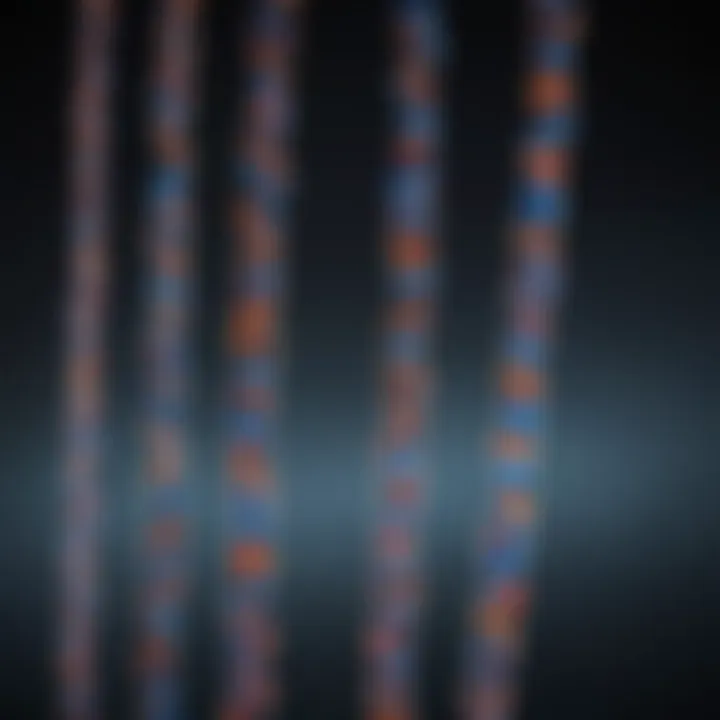
Gene Set Enrichment Analysis
Gene Set Enrichment Analysis (GSEA) serves as a complementary technique that builds on the results obtained from DGEA. It focuses on identifying whether predefined sets of genes show statistically significant differences in expression between two biological states. This technique is valuable for understanding the functional implications of the changes observed in gene expression.
The GSEA process typically involves the following steps:
- Predefining Gene Sets: Researchers utilize curated lists of genes associated with specific biological pathways or processes. These sets can be sourced from various databases, enhancing their relevance to the research question at hand.
- Ranking Genes: Genes are ranked based on their expression differences. This ranking helps in assessing the extent to which the gene sets are enriched in the lists of differentially expressed genes.
- Statistical Assessment: GSEA employs statistical methods to evaluate whether the observed enrichment is greater than would be expected by chance.
The advantages of GSEA in the Galaxy framework include:
- Biological Relevance: By focusing on gene sets rather than individual genes, GSEA provides a context that may reveal important biological insights.
- Comprehensive Analysis: GSEA enables integrative analysis of gene expression data and has the potential to highlight pathways or processes that may otherwise be missed.
- User-Friendly Execution: Like DGEA, GSEA in Galaxy allows researchers to perform complex analyses without requiring extensive computational background or programming knowledge.
Overall, the data analysis techniques in Galaxy empower researchers to extract and interpret valuable biological information from RNA sequencing data, making these methods critical for advancing genomic research.
Interpreting RNA Sequencing Results
Interpreting RNA sequencing results is crucial for deriving meaningful conclusions from genomic studies. As RNA sequencing methods generate vast amounts of data, effective interpretation becomes a challenge and necessity. Misinterpretation can lead to incorrect insights, which deeply impact research outcomes. Thus, careful consideration must be given to analyzing and contextualizing the data collected.
Analyzing Output Data
When analyzing output data from RNA sequencing, researchers must follow a systematic approach. The analysis typically begins with assessing the quality of the sequence reads. Programs like FastQC allow users to visualize raw data and identify potential issues, such as low-quality bases or overrepresented sequences.
Once quality is ensured, raw reads are aligned against a reference genome using alignment tools like STAR or HISAT2. The alignment step produces a file that marks the location of each read, providing insight into gene expression levels.
Quantifying gene expression is the next task. Common tools include DESeq2 and EdgeR, which help in normalizing data and determining differential expression. It's critical to adjust for factors such as library size and sequencing depth to ensure accurate findings. Additionally, understanding statistical noise in the data can aid in refining results.
Some key steps in analyzing output data include:
- Quality assessment of raw reads
- Alignment of reads to the reference
- Quantification of gene expression
- Statistical analysis for significance
Effective data analysis will lead to more robust interpretations, facilitating the connection of RNA sequencing results to biological phenomena.
Visualizing Results within Galaxy
Visualization tools within the Galaxy platform play a vital role in understanding RNA sequencing output. Galaxy offers a user-friendly interface that simplifies the process of creating visual representations of complex data. Users can generate plots, graphs, and charts to reveal gene expression patterns and differences.
Common visualization techniques include heatmaps, volcano plots, and PCA plots. Each provides unique insights:
- Heatmaps offer a way to visualize gene expression levels across different samples, highlighting patterns of co-expression.
- Volcano plots display the relationship between statistical significance and fold changes, which aids in identifying genes of interest.
- PCA plots show how samples cluster based on expression profiles, revealing underlying relationships.
Such visualizations not only enhance data understanding but also facilitate communication of results in research settings. By leveraging Galaxy’s capabilities, researchers gain deeper insights into their findings.
The effectiveness of RNA sequencing relies not solely on data generation but significantly on how results are interpreted and visualized.
In summary, interpreting RNA sequencing results involves thorough analysis of output data and effective visualization using Galaxy. Both processes are interconnected and contribute to drawing meaningful conclusions that advance research in genomics.
Case Studies of Galaxy RNA Seq Analysis
Case studies serve as a critical component in understanding the practical applications of Galaxy RNA sequencing analysis. They provide tangible examples of how theoretical concepts manifest in real-world research settings. These studies demonstrate the methodology's effectiveness and versatility in addressing varied scientific problems.
Using case studies allows researchers and practitioners to glean insights from the successes and challenges faced in prior projects. Specifically, they highlight how data is collected, processed, and interpreted, providing a clear pathway for others to follow. Additionally, the reflections on these studies bring to surface important considerations regarding reproducibility, ethical implications, and the necessity for detailed documentation.
In this section, we will examine two major applications of Galaxy RNA sequencing analysis: its role in cancer research and its use in developmental biology studies. Each of these areas showcases the relevance and potential of RNA sequencing in deriving meaningful biological insights.
Application in Cancer Research
Cancer research has witnessed a significant transformation with the advent of RNA sequencing technologies. By applying Galaxy RNA sequencing analysis, researchers can delve into the molecular underpinnings of various cancers. This approach facilitates the identification of differentially expressed genes, enabling the understanding of tumor behavior, classification, and prognosis.
Several case studies demonstrate how Galaxy enables the analysis of complex tumor samples to uncover mutations and gene expression changes that correlate with patient outcomes. For instance, a study focusing on breast cancer revealed distinct gene expression profiles that could predict resistance to specific therapies. This finding underscores the importance of personalized medicine, where treatments can be tailored based on individual genetic profiles.
"The use of RNA sequencing in oncology has not only enhanced our understanding of tumor biology but also paved the way for targeted therapies that can significantly improve patient outcomes."
Moreover, studies explore the tumor microenvironment and its influence on cancer progression. By integrating RNA sequencing data from both primary tumors and surrounding tissues, researchers can identify how the immune response is modulated in cancer settings. This holistic view can establish innovative therapeutic targets and improve patients' immune responses during treatment.
Role in Developmental Biology Studies
Developmental biology studies significantly benefit from the insights gained through Galaxy RNA sequencing analysis. Researchers employ this methodology to understand the gene regulatory mechanisms that govern organismal development. The detailed data from RNA sequencing allows for the examination of gene expression variations during crucial developmental stages.
For instance, one case study investigating embryonic stem cells demonstrated how specific gene clusters are activated at defined stages of differentiation. This type of analysis reveals key regulatory genes that orchestrate the transition from pluripotency to lineage commitment.
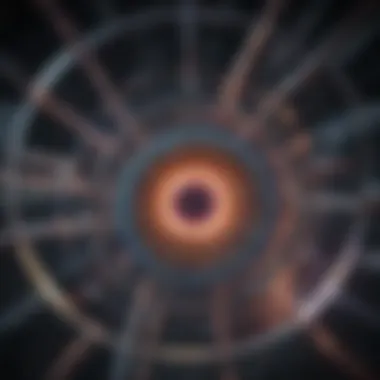
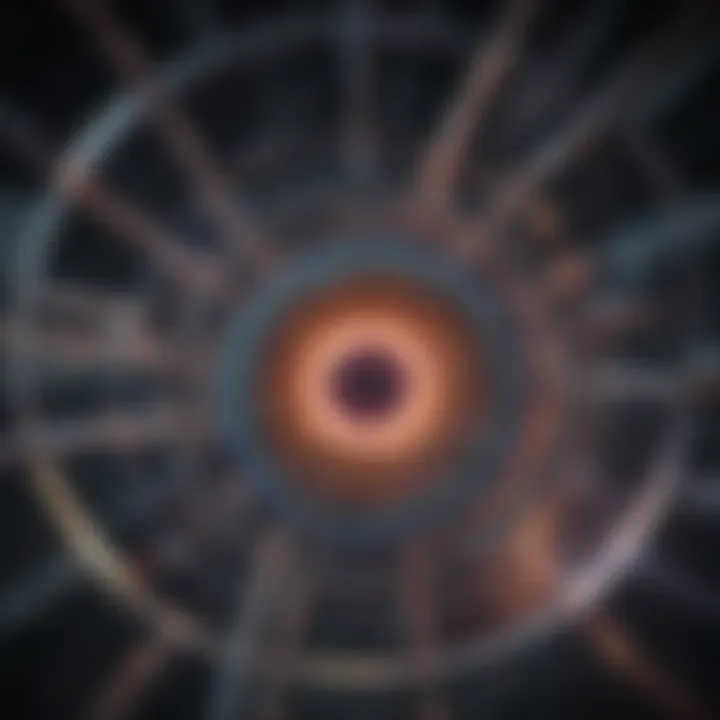
Furthermore, developmental biology researchers leverage RNA sequencing to study evolution and species-specific traits. By comparing RNA profiles across different organisms, scientists can identify conserved and divergent pathways that underpin developmental processes.
As developmental biology continues to evolve, Galaxy's role will remain foundational in deciphering complex regulatory networks and advancing our knowledge of biological development. Each case study not only illustrates methodology application but also inspires further research into the implications of gene regulation across life forms.
Future Directions in RNA Sequencing Analysis
Understanding the future directions of RNA sequencing analysis is crucial for researchers and professionals in genomics and molecular biology. This section looks at emerging technologies and the integration of RNA sequencing with other omics data. These trends will shape the way RNA sequencing is utilized in various fields and how data from RNA sequencing can be enhanced through advanced technologies.
Emerging Technologies
New technologies are constantly emerging in the field of RNA sequencing. These innovations improve accuracy, reduce costs, and increase throughput. Significant developments include long-read sequencing and single-cell RNA sequencing.
- Long-Read Sequencing: Technologies such as Pacific Biosciences and Oxford Nanopore have revolutionized how we approach RNA sequencing. They offer longer reads that can capture full-length transcripts, thus providing a more comprehensive view of transcriptomes. This capability is particularly beneficial for examining complex genes and alternative splicing events.
- Single-Cell RNA Sequencing: This technique allows for the analysis of gene expression at a single-cell level. It has great implications for understanding cellular heterogeneity in tissues. This technology addresses the need to differentiate between the various cell types present in a biological sample, thereby offering insights into how specific cell populations respond in disease or development.
Incorporating these emerging technologies into ongoing RNA sequencing practices not only enhances the resolution of data but also facilitates deeper biological insights.
Integration with Other Omics Data
Integrating RNA sequencing data with other omics data, such as genomics, proteomics, and metabolomics, represents a critical step forward. This multi-omics approach allows for a more holistic understanding of biological systems.
This integration has several benefits:
- Comprehensive Insight: By combining RNA data with genomic and proteomic information, researchers can build a more complete picture. For instance, correlating transcriptomic data with protein expression levels can elucidate the mechanisms behind gene regulation.
- Cross-Validation of Data: Integrating various data types helps validate findings across platforms. For example, discrepancies between gene expression and protein levels can shed light on post-translational modifications or differences in protein stability.
- Enhanced Disease Understanding: In clinical research, multi-omics data can identify biomarkers and therapeutic targets more effectively. For instance, studying both RNA and metabolites may reveal how diseases progress at a molecular level, leading to novel interventions.
In summary, future directions for RNA sequencing analysis promise to refine methodologies and expand capabilities, making it essential for the forward movement of genomic science and its applications in health and disease.
Challenges in RNA Sequencing Analysis
The process of RNA sequencing presents various challenges that can significantly impact the outcomes of genomic research. Addressing these challenges is crucial for ensuring the validity and reliability of the analysis. As RNA sequencing becomes increasingly fundamental in both academic and clinical settings, understanding the complexities involved can help optimize the analysis process. This section delves into two primary challenges: data complexity and volume, and reproducibility issues.
Data Complexity and Volume
RNA sequencing generates massive amounts of data, which can overwhelm computational tools and analysis platforms. This complexity arises from various factors, including:
- Diverse RNA species: RNA molecules differ widely in sequence length and abundance, leading to a complex sequencing landscape. The presence of messenger RNA (mRNA), non-coding RNA (ncRNA), and small RNA requires sophisticated handling strategies to ensure meaningful interpretations.
- High-throughput technologies: Advances in sequencing technologies, such as Illumina and PacBio, yield extensive datasets. While they provide depth and breadth of coverage, this also results in increased analysis demands. Processing and filtering out noise from irrelevant reads or low-quality sequences adds another layer of difficulty.
- Biological variability: The inherent variability in biological samples means that even technical replicates may yield different results. This variability complicates the comparison of data across experiments or conditions.
Effectively managing these challenges is vital for successful RNA sequencing analysis. Practitioners must employ robust statistical methods and bioinformatic tools to process the high volume of data efficiently. The use of Galaxy's platforms can facilitate the management of data complexity through its user-friendly interface.
Reproducibility Issues
Reproducibility is foundational to scientific research, yet RNA sequencing analysis often faces hurdles that hinder replicability. Key factors contributing to this challenge include:
- Sample handling: Variations in sample preparation, storage, and processing can introduce biases. Even slight differences in how samples are treated can impact the final results. Researchers must adhere to stringent protocols to minimize variability and ensure consistent sample handling.
- Bioinformatic pipelines: Diverse analytical pipelines can produce different results depending on the methods and parameters used. The lack of standardized protocols across studies complicates comparisons and validation of findings. It is essential to document the analytical methods comprehensively to promote reproducibility.
- Data interpretation: The subjective nature of interpreting RNA sequencing data can also lead to inconsistencies. Different researchers might draw varying conclusions from the same dataset, which can obscure the validity of results.
"Ensuring reproducibility in RNA sequencing analysis is as important as the initial data collection. Both stages must align to build credible and reliable scientific findings."
Addressing these reproducibility challenges is crucial. Establishing standardized protocols and providing clear reporting of methods can significantly enhance the reliability of RNA sequencing studies. Leveraging Galaxy can also help streamline and standardize workflows, making it easier for researchers to replicate results and expand upon previous studies.
Overall, recognizing and tackling these challenges is integral for advancing RNA sequencing analysis, enhancing the quality of genomic research, and ensuring the creation of reliable scientific knowledge.
The End
In this section, we delve into the significance of the conclusion regarding Galaxy RNA sequencing analysis. This part of the article emphasizes the overarching themes and findings presented throughout. The importance of synthesizing the key insights cannot be overstated, as it ensures that the reader retains a clear understanding of the methodology, tools, and applications discussed.
One primary benefit of drawing a conclusion is that it condenses complex information into digestible points. This allows readers to reflect on the implications of RNA sequencing within the broader context of genomic research. Moreover, understanding how Galaxy facilitates RNA sequencing analysis positions it as a vital tool in both academic and clinical environments.
It is also important to consider the growing challenges faced in bioinformatics. Inevitably, as RNA sequencing data continues to expand, the methodologies and platforms must evolve correspondingly. Thus, the conclusion serves as a point of contemplation for future directions in RNA sequencing analysis, pointing towards potential advancements and integrations with emerging technologies.
"In essence, a well-crafted conclusion not just summarizes but also invites readers to explore further, sparking curiosity and continued engagement with the material."
Summarizing Key Insights
The article highlights several key insights regarding Galaxy RNA sequencing analysis. These insights outline the critical components and functionalities of the platform. Notable points include:
- User-Friendly Interface: Galaxy’s design supports both novice and experienced users, making complex tools accessible.
- Hosting Diverse Tools: The platform integrates a range of bioinformatics tools, allowing for comprehensive data analysis without extensive programming knowledge.
- Importance in Different Fields: RNA sequencing techniques discussed here have many applications, from cancer research to developmental biology.
- Best Practices: Following best practices in data analysis is essential to maximize research output and ensure reliable results.
These insights collectively reinforce the value of Galaxy as an analytical tool in the fast-evolving field of genomics, ensuring that users can navigate complexities with greater ease.
Final Reflections on Galaxy RNA Seq Analysis
In concluding this exploration of Galaxy RNA sequencing analysis, reflections on its impact must be addressed. The platform has significantly reshaped how researchers approach RNA sequencing, facilitating not only data processing but also the integration of analyses into larger biological inquiries. One of the transformative aspects of Galaxy is its ability to connect critical datasets with practical applications, underscoring the importance of computational tools in biological research.
Moreover, as the landscape of genomics continues to evolve, Galaxy must adapt to keep pace with technological advancements. This adaptability is crucial for maintaining its relevance in a field that increasingly relies on high-throughput sequencing technologies.
Ultimately, the ultimate takeaway from this discussion is that Galaxy, as an analysis framework, holds the promise of enabling deeper insights into the complexities of RNA biology. As researchers harness its capabilities, they contribute not just to individual projects, but also to the collective understanding of genetic phenomena. Reinforcing the significance of these analyses in both scientific inquiry and clinical implications ensures that the dialogue surrounding RNA sequencing continues to flourish.