Exploring the RNA Database: An In-Depth Overview

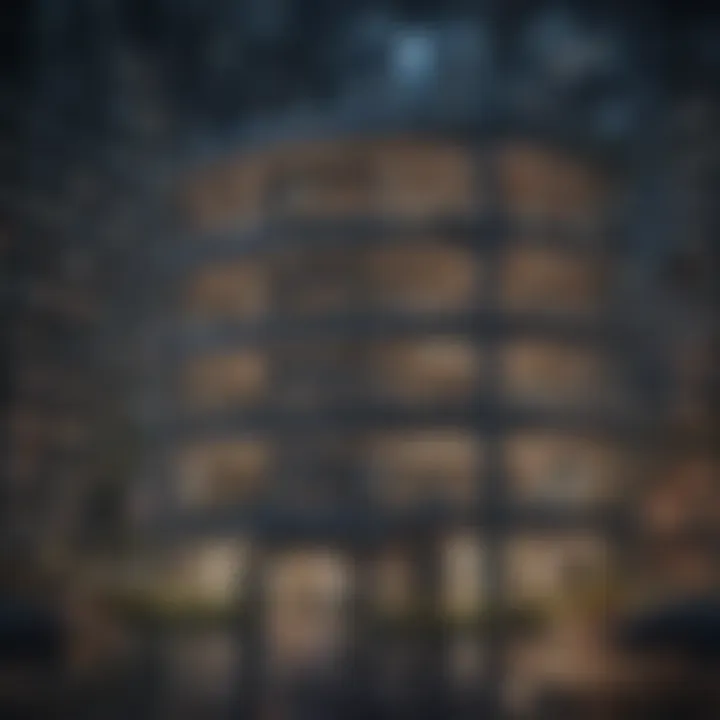
Intro
The realm of genetics and molecular biology has witnessed transformative advancements, particularly with the advent of RNA databases. These databases serve as repositories of vital genetic information, allowing researchers to access and manipulate data crucial for understanding cellular processes. RNA databases are inherently complex, yet their purpose is straightforward: to facilitate research that aims to decode the myriad functions of RNA within biological systems.
As scientists work to unravel the intricacies of genetics, understanding RNA’s role becomes pivotal. This article thoroughly explores the RNA database, examining its structure, significance, and the applications it holds for researchers across various fields. We also consider the inherent challenges present in handling large volumes of data and the implications these databases manifest for future research.
Research Overview
Summary of Key Findings
The exploration of RNA databases reveals several fundamental aspects:
- Structure: RNA databases are typically organized to support both raw data and processed information, which are crucial for various analyses.
- Function: Essential for gene expression studies, RNA databases facilitate a deeper understanding of molecular interactions.
- Applications: They are widely used in areas such as drug discovery, disease modeling, and evolutionary studies, showcasing their versatility.
- Challenges: Data standardization and integration remain persistent obstacles. The dynamic nature of RNA also complicates data curation.
The significance of these databases extends beyond mere data storage; they are instrumental in driving innovation and intellectual progress in genetics. Researchers can now access a wealth of information that influences experimental design and hypothesis generation.
Relevance to Current Scientific Discussions
The discussions surrounding RNA databases are increasingly relevant as genomic research progresses. The recent focus on personalized medicine amplifies the need for precise and comprehensive RNA analysis. Through RNA databases, scientists can identify biomarkers for diseases, which leads to more targeted treatment options.
Moreover, the evolution of RNA sequencing technologies makes RNA databases invaluable. The integration of high-throughput sequencing data into databases enables more extensive and detailed investigations of RNA functions. The scientific community is engaging in robust conversations about data sharing ethics and accessibility, marking a significant step toward collaborative research efforts.
Culmination
Prolusion to RNA Databases
RNA databases serve as invaluable resources for researchers and academics in the fields of genetics and molecular biology. They provide a structured framework for storing and accessing vast amounts of data related to ribonucleic acids. The importance of RNA databases goes beyond mere data collection; they are pivotal tools for analysis, interpretation, and innovative research in understanding the complex roles of RNA in biological systems.
In this article, we will explore the essential components of RNA databases as well as their applications. Insight into the definition and importance of these databases will set the stage for a more in-depth examination. Understanding how RNA databases function serves various benefits for researchers, including streamlining the research process and facilitating data sharing across scientific communities. Their ability to integrate large datasets enables more thorough investigative approaches, allowing for advancement in scientific knowledge and application.
Definition of RNA Databases
RNA databases are specialized repositories where information related to RNA molecules is systematically organized and made accessible. This definition encompasses various forms of data, including nucleotide sequences, structure data, and functional annotations. Unlike general biological databases, RNA databases focus specifically on RNA, encompassing a wide range of elements such as data on mRNA, rRNA, tRNA, and non-coding RNAs.
These databases can be populated with data derived from experimental findings or computational predictions. Both types of data enhance the overall richness of the resources available. Significant databases like GenBank and Rfam contribute positively to the understanding of RNA by offering clear interfaces and comprehensive datasets that cater to diverse user needs, from novices to experienced scientists.
Importance in Molecular Biology
The relevance of RNA databases in molecular biology cannot be overstated. RNA plays critical roles in the process of gene expression and regulation. Through these databases, researchers can access essential information that aids in elucidating the functions and mechanisms underlying RNA operations. This insight is crucial for multiple areas of scientific inquiry, including evolutionary biology, genetics, and medical research.
Moreover, RNA databases assist in identifying RNA-related biomarkers that can guide disease diagnosis and treatment. By aggregating and analyzing RNA sequences and structures, researchers can uncover patterns associated with specific disorders.
A few key points to consider regarding the importance of RNA databases are:
- Facilitating Research: They provide the backbone for research initiatives aimed at understanding RNA roles in life processes.
- Data Sharing: They enable the sharing of knowledge across research institutions, fostering collaboration.
- Supporting Innovation: RNA databases help drive innovations in fields such as synthetic biology and biotechnology, where understanding RNA is crucial.
- Education and Training: These databases serve as educational resources for students and professionals seeking to deepen their understanding of RNA biology.
This resource facilitates the advancement of RNA research by ensuring accessible and reliable data, crucial for scientific progress.
In summary, the introduction of RNA databases into the molecular biology landscape marks a significant turning point for research and innovation. As we progress through this article, we will delve deeper into the various types and applications of these databases, showcasing their essential role in driving the field forward.
Types of RNA Databases
Understanding the various types of RNA databases is crucial for researchers and professionals in the field. Specifically, these databases serve as repositories of RNA sequence information, structural details, and functional annotations. By categorizing RNA data, scientists can efficiently access relevant information that aids in research, development, and educational activities. This section will delineate the three predominant types of RNA databases and illuminate their individual importance to the scientific community.
Sequence Databases
Sequence databases are essential assets in RNA research. They store nucleotide sequences that provide the foundational data for investigations into RNA biology. Examples include GenBank and Ensembl. These platforms allow researchers to retrieve sequences from different organisms, enabling comparative analyses.
Key features of sequence databases include:
- Wide coverage of RNA sequences from various species,
- User-friendly search tools for quick access to specific RNA sequences,
- The ability to download sequences for further analysis.
Researchers can utilize these databases to identify novel RNAs or investigate the variations in known RNA sequences. This, in turn, helps in understanding evolutionary relationships, gene regulation, and metabolic pathways.
Structural Databases
Structural databases focus on the three-dimensional structures of RNA molecules. Resources like the RNA STRUCTURE and the PDB (Protein Data Bank) provide detailed structural information that is critical for understanding the function of RNA. This is particularly important because the shape of an RNA molecule greatly influences its behavior in biological contexts.
Some important aspects of structural databases are:
- Visual representations of RNA structures, making it easier to comprehend complex molecules,
- The ability to access quality metrics for the structures, ensuring reliability in data use,
- Inclusion of complementary data, such as associated proteins or ligands.
These databases assist researchers in predicting how RNA will behave in different environments, enabling better insights into RNA function and interaction.
Functional Databases
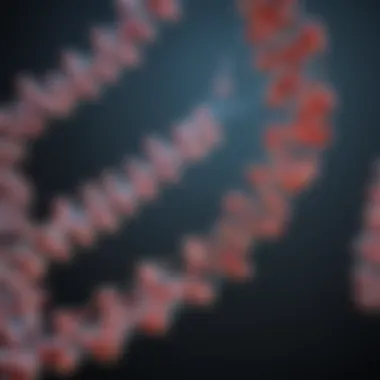
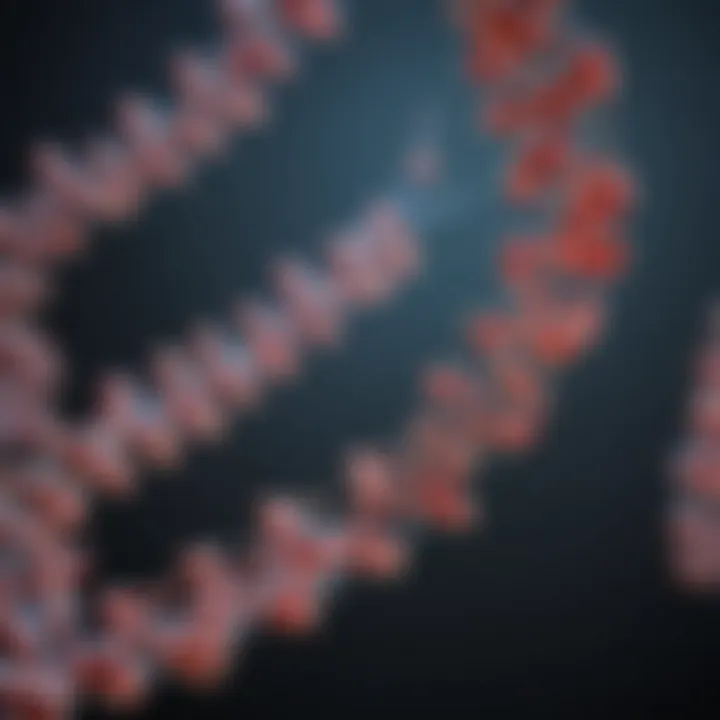
Functional databases catalog the roles that specific RNAs play within biological systems. They provide insights into RNA functions like gene expression regulation and involvement in various cellular processes. Examples of such functional databases include Rfam and MODOMICS.
Important characteristics of functional databases include:
- Detailed annotations regarding RNA function and its interactions with other biomolecules,
- Information on phenotypic effects associated with various RNA sequences,
- Integration of experimental data, facilitating the connection between structure and function.
Understanding functional aspects of RNA is vital for advancements in genetic research and therapeutic developments.
In summary, each type of RNA database plays a pivotal role in the overall research landscape. By providing specific kinds of information—sequence, structure, or function—these databases enhance our ability to conduct comprehensive analyses in molecular biology. As researchers navigate these databases, they gain critical insights that foster innovation and deeper understanding within the scientific community.
"The true value of RNA databases lies in their integration and the potential they offer for advancing scientific knowledge."
Through careful exploration of these database types, researchers can maximize their contributions to the field and continue pushing the boundaries of RNA research.
Key RNA Databases
RNA databases serve as essential resources for researchers in molecular biology and genetics, providing a repository for vast amounts of RNA sequence data. Familiarity with key RNA databases helps scientists access reliable information for their research projects. Understanding these databases can significantly enhance research outcomes and lead to new discoveries.
GenBank
GenBank, developed by the National Center for Biotechnology Information (NCBI), is among the most comprehensive and widely used databases for molecular biology data. It provides an annotated collection of all publicly available DNA sequences. This includes both coding and non-coding RNA sequences, making it an invaluable resource for gene discovery and functional annotation.
Researchers can leverage GenBank for multiple purposes. For instance, it aids in comparative genomics by allowing users to analyze sequence similarity across different species. Furthermore, GenBank often provides links to related literature, enhancing the utility of the database beyond simple retrieval of sequences. Quality control and regular updates ensure the data remains current and relevant for scientific use.
Ensembl
Another prominent RNA database is Ensembl, which is jointly developed by the European Bioinformatics Institute (EBI) and the Wellcome Trust Sanger Institute. Ensembl focuses primarily on genomic data from various organisms, including humans, and includes comprehensive RNA data. The platform integrates multiple data types, providing a holistic view of genes, their structures, and their relationships across different species.
One important feature of Ensembl is its advanced visualization tools. These allow scientists to view the genomic context of RNA sequences in relation to genes, regulatory elements, and evolutionary conservation. Researchers often appreciate the ability to browse through transcript variants, which can be critical in understanding the functional implications of RNA molecules in various biological processes.
Rfam
Rfam specializes in the classification and annotation of RNA families. It provides a curated collection of RNA sequence families, describing their secondary structures and associated motifs. Rfam offers tools that assist researchers in identifying known RNA families in their sequences, which can be particularly beneficial for studying non-coding RNAs.
The database plays a critical role in predicting RNA function, as it integrates different methods to provide high-quality alignments and models of RNA family structures. Rfam supports comparative analyses across related sequences, enabling researchers to investigate evolutionary relationships among RNA molecules. With its focus on RNA families, Rfam complements the broader sequence data available in GenBank and Ensembl, fostering a comprehensive understanding of RNA's role in biology.
Data Collection Methodologies
The methodologies for collecting data in RNA research are crucial. These methods impact the quality and reliability of the data stored in RNA databases. Understanding these methodologies not only helps researchers in data acquisition, but also ensures consistent and comprehensive data integration into existing databases. The advantages of well-thought-out methodologies are multiple.
Effective data collection can lead to higher accuracy in research findings. Scientists utilize various methods to gather data, each with its own strengths and weaknesses. It is imperative for researchers to select the most appropriate techniques based on their specific needs and contexts.
Experimental Techniques
Experimental techniques are fundamental in generating primary data for RNA databases. They encompass various laboratory methods used to study RNA molecules. Common experimental methods include RNA extraction, RT-PCR, and RNA sequencing.
- RNA extraction involves isolating RNA from biological samples, which is a critical first step. This process must be performed cautiously to avoid RNA degradation.
- RT-PCR (Reverse Transcription Polymerase Chain Reaction) allows researchers to amplify RNA sequences for further analysis. This technique is pivotal in understanding gene expression levels.
- RNA sequencing offers a more comprehensive view, enabling scientists to analyze the entire RNA population in a sample. This method provides insights into not just the quantity of RNA, but also the diversity of RNA types present.
Each of these techniques provides data that can be stored in databases like GenBank or Rfam. The quality of data obtained is highly contingent on the precision of these experimental approaches.
Computational Approaches
In the realm of RNA data collection, computational approaches play an equally significant role. They are essential for managing the vast amounts of data generated through experimental techniques. Key computational methodologies used include bioinformatics tools and machine learning models.
Bioinformatics tools enable researchers to analyze sequences, predict RNA structures, and evaluate functional aspects. For example, tools like BLAST facilitate sequence alignment, which helps identify homologies between RNA sequences.
- Machine learning models are increasingly being used to predict RNA functions from structural data. They can identify patterns that might not be visible through traditional analytical methods.
- Moreover, these models assist in data integration from multiple sources, allowing for a more holistic view of RNA biology.
When combined, these computational approaches complement experimental techniques. Together, they bolster the process of data collection, ensuring that RNA databases are up-to-date and comprehensive.
In summary, data collection methodologies in the context of RNA research involve a blend of experimental techniques and computational approaches. Both are vital in maintaining data integrity within RNA databases, thus supporting advancements in molecular biology and genetic research.
Applications of RNA Databases
RNA databases play a crucial role in the advancement of genetic and molecular biology research. Their applications are manifold, influencing key areas such as genetic research, disease studies, and pharmaceutical development. Each subsection sheds light on specific aspects that highlight the importance and versatility of RNA databases in the scientific community.
Genetic Research
In genetic research, RNA databases serve as essential tools for understanding gene expression patterns and their regulatory mechanisms. Researchers utilize data from databases like GenBank and Rfam to analyze RNA sequences, which aids in identifying potential gene functions and interactions. Moreover, RNA-seq data, stored in these databases, allows scientists to quantify transcript abundance across various conditions, providing insights into cellular responses. This comprehensive genetic information enhances our knowledge of evolutionary biology and comparative genomics.
Disease Studies
RNA databases are invaluable for disease research, particularly in understanding the molecular basis of various conditions. By correlating RNA expression profiles with disease states, researchers can identify biomarkers for early diagnosis and prognosis. For instance, analyzing data on non-coding RNAs has led to discoveries regarding their roles in cancer and other chronic diseases. Furthermore, studying differential gene expression patterns contributes to targeted therapies, enhancing patient outcomes. Thus, integrating RNA data with other omic technologies improves the capacity to investigate complex diseases.
Pharmaceutical Development
The pharmaceutical industry leverages RNA databases extensively during drug discovery and development processes. By examining RNA interactions with drug molecules, scientists can predict efficacy and safety of new therapies. The databases enable researchers to screen for genetically modified organisms or cells that may reveal new drug targets, expediting the research timeline. Additionally, RNA sequencing data can inform the development of innovative RNA-based therapeutics, such as mRNA vaccines, that have gained prominence recently. Therefore, RNA databases are instrumental in driving pharmaceutical innovation and improving therapeutic strategies.
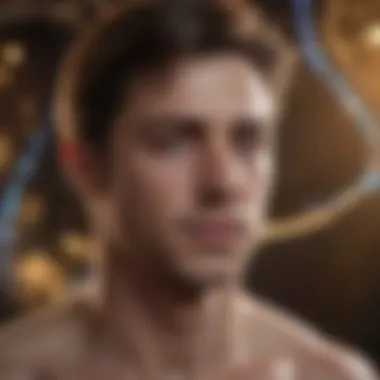
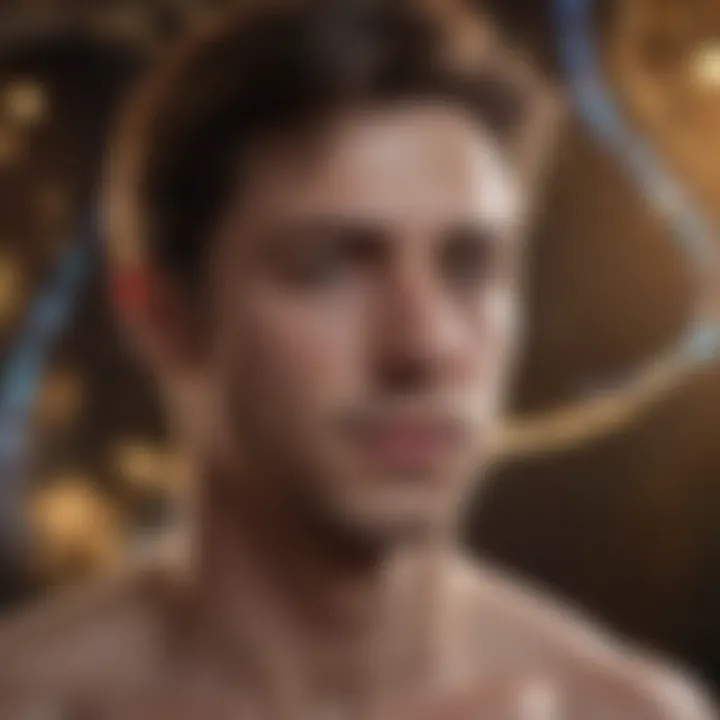
"The accessibility of RNA databases accelerates advancements in biomedical research, fostering collaborations that transcend traditional disciplinary boundaries."
In summary, the applications of RNA databases enrich the fields of genetic research, disease studies, and pharmaceutical development. They not only enhance the understanding of biological processes but also facilitate the translation of scientific findings into clinical practice. As the volume of RNA data continues to grow, the ongoing integration of these databases into research workflows will undoubtedly yield significant benefits in addressing complex biological questions.
Integration with Other Databases
RNA databases serve as crucial resources in molecular biology, but their potential is significantly enhanced through integration with other databases. This combination allows for a more comprehensive analysis, supporting research across various biological fields. Connecting RNA data with other data types fosters a better understanding of gene expression, protein interactions, and genomic landscapes.
Connecting RNA and Protein Databases
The relationship between RNA and protein databases is pivotal. RNA is a fundamental intermediary in gene expression, translating genetic information into functional proteins. By integrating RNA sequence data from databases like GenBank with protein databases such as UniProt, researchers can uncover valuable insights.
For example, knowing the mRNA sequence helps in predicting the resulting protein structure and function. This crossover aids in functional annotation of genes, enabling researchers to delve into the specific roles proteins play in cellular processes. Moreover, it allows for the tracking of mutations in RNA that may lead to changes in protein function, which is essential in studying diseases. The associated benefits of integrating RNA and protein databases include:
- Improved Gene Annotation: Provides a clearer picture of gene functions and interactions.
- Enhanced Predictive Models: Supports models in bioinformatics that predict protein structures and interactions based on RNA sequences.
- Facilitated Drug Discovery: Helps identify potential drug targets by understanding protein functions derived from RNA data.
Cross-referencing with Genomic Databases
Cross-referencing RNA databases with genomic databases is another critical area. Genomic databases, such as Ensembl, house rich genetic information relevant to the DNA sequences from which RNA transcripts are derived. This integration provides insights into how genes are regulated and expressed across different conditions.
When RNA databases are cross-referenced with genomic data, several advantages become evident:
- In-depth Analysis of Regulatory Elements: It allows researchers to identify promoter regions, enhancers, and other regulatory elements that influence RNA production.
- Variant Analysis: Researchers can better investigate the effects of genomic variants on RNA transcripts and their consequent impact on protein synthesis.
- Comprehensive Pathway Mapping: By understanding both genomic and transcriptomic data, scientists can map biological pathways more accurately.
"Integrating RNA databases with genomic and protein information is essential for advancing our understanding of biological systems."
The future of molecular biology relies on such integrations. They not only streamline research but also enhance collaborative efforts between researchers from different disciplines. As technologies evolve, the potential for more effective integration will likely increase, promoting more robust advancements in the field.
Emerging Technologies and RNA Databases
Emerging technologies significantly transform the landscape of RNA databases. They offer innovative tools and techniques for researchers to analyze and interpret complex data. As the demand for high-throughput sequencing and data analysis increases, understanding advancements in this field becomes essential.
Next-Generation Sequencing
Next-Generation Sequencing (NGS) has revolutionized the way we gather RNA sequence data. Traditional sequencing methods were time-consuming and limited in scope. NGS enables rapid sequencing of millions of fragments simultaneously, providing a comprehensive view of the RNA landscape. This technology enhances the identification of novel RNA molecules, including non-coding RNAs that play crucial roles in regulation and cellular function.
The benefits of NGS are numerous:
- High Throughput: Enables sequencing of large volumes of RNA samples in a short period.
- Cost-Effectiveness: Reduces the cost per base of sequencing, promoting broader access for researchers.
- Enhanced Precision: Improves accuracy in identifying mutations and variations in RNA sequences.
Moreover, NGS can be paired with other methodologies, such as RNA-Seq, to yield information on gene expression levels. This integration allows for better understanding of how genes are regulated at the RNA level. Insight gained from NGS fuels research in genetic disorders, cancer biology, and developmental biology.
Artificial Intelligence in Data Analysis
Artificial Intelligence (AI) is another cutting-edge technology that impacts RNA databases. It offers powerful algorithms for analyzing vast amounts of RNA data efficiently. By utilizing machine learning models, researchers can uncover patterns and relationships that would otherwise remain hidden in complex datasets.
AI brings several specific advantages to RNA data analysis:
- Predictive Modeling: Anticipates the behavior of RNA molecules based on existing data, enabling hypothesis generation.
- Data Integration: Combines diverse datasets from multiple sources, providing a holistic view of RNA functions and interactions.
- Automated Annotation: Facilitates quicker identification of RNA types and their attributes, streamlining data curation processes.
Through the synergy of AI and RNA databases, researchers can navigate the challenges of data overload. AI algorithms sift through noise and identify relevant information, thus enhancing the utility of RNA databases in research.
The integration of emerging technologies like NGS and AI into RNA databases highlights the need for continual adaptation in research practices. As these technologies evolve, they will pave the way for groundbreaking discoveries in molecular biology and genetics.
Data Quality and Standards
The reliability of RNA databases hinges on data quality and standards. High-quality data is fundamental for rigorous scientific research. If data is flawed or inconsistent, it can lead to misleading conclusions and hinder advancements in molecular biology. Thus, establishing robust quality control measures and adhering to standardized protocols are essential for maintaining data integrity.
Quality Control Procedures
Quality control procedures involve systematic checks to ensure the accuracy and reliability of data within RNA databases. These procedures may include:
- Data Validation: Verifying that the data sets comply with predefined standards before inclusion in the database. This may encompass checking sequence accuracy and matching entries against established references.
- Regular Audits: Conducting routine audits to identify errors or discrepancies. These can highlight areas needing revision or removal from the database.
- User Feedback Mechanisms: Providing platforms for users to report errors or suggest improvements contributes to continuous quality improvement.
Implementing these procedures not only enhances the credibility of the database but also supports the wider scientific community by offering reliable data for research.
Standardization Challenges
While standardization is vital, it presents significant challenges. The RNA research landscape is diverse, with various formats, protocols, and systems used by researchers. Key challenges include:
- Diverse Data Formats: RNA sequences can come in different formats, complicating the integration across databases.
- Protocol Variability: Variations in the methods used to obtain RNA data can lead to inconsistencies, making it hard to standardize.
- Global Collaboration Issues: In a worldwide research community, differing standards can hinder collaborative efforts. Cross-referencing databases becomes more difficult when varying standards exist.
These challenges necessitate ongoing discussions and collaborations among databases worldwide to improve standardization efforts. By addressing these issues, the RNA databases can ensure data quality and enhance their overall usability in research.
Ethical Considerations in RNA Research
Ethical considerations in RNA research are increasingly critical. As research advances, the handling of RNA data raises significant questions about integrity, privacy, and responsibility. Given the potential uses of RNA data in various fields, such as genomics, drug development, and disease prevention, it becomes essential to navigate these ethical dilemmas thoughtfully. Ethical frameworks must guide researchers to ensure that both scientific progress and respect for individual rights coexist.
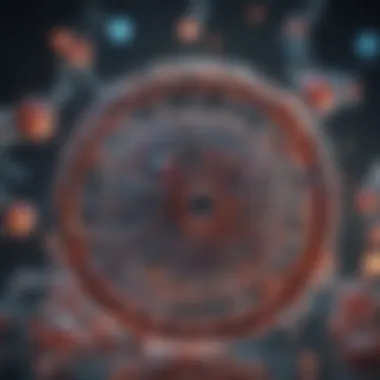
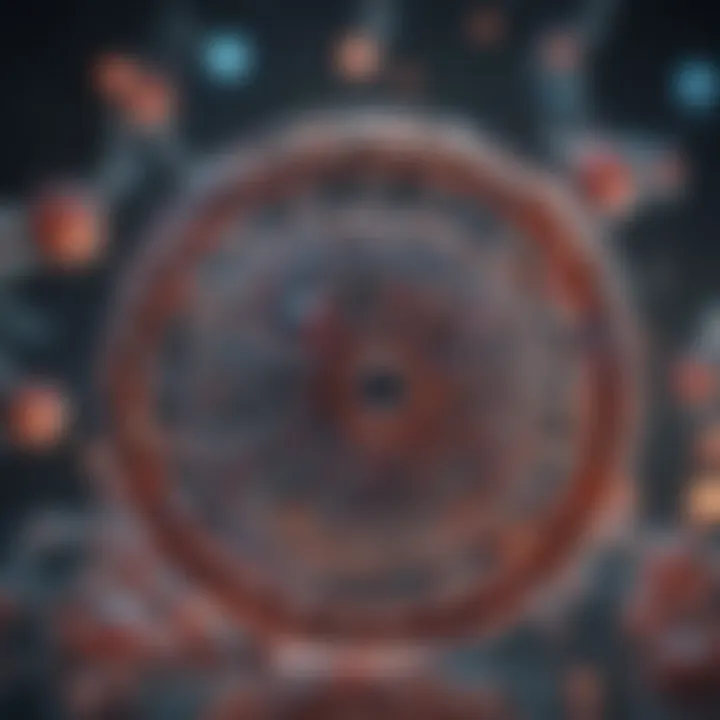
Data Privacy Issues
Data privacy in RNA research is a multifaceted concern. Researchers often deal with sensitive information that could impact an individual's privacy. When RNA sequencing data is linked to personal identifiers, it presents risks of unauthorized access and misuse. The potential for re-identification of individuals from genomic data raises alarms about consent and confidentiality.
To mitigate these risks, best practices include:
- Informed Consent: Ensure participants are fully aware of how their data will be used.
- Anonymization: Remove identifiable information to protect personal identities.
- Data Security: Implement strong data security measures to prevent breaches.
The increasing visibility of RNA databases creates a pressing need for stringent privacy standards. Ensuring robust data protection frameworks is not just a legal obligation, but also a moral one that researchers must uphold.
Responsible Use of RNA Data
Responsible use of RNA data encompasses not just ethical considerations but also the potential consequences of data misapplication. Misusing RNA data can lead to wrongful conclusions, stigmatization of populations, or flawed research outputs. Researchers must approach data analysis with care, ensuring their findings contribute positively to scientific discourse and societal benefit.
Key elements that support responsible data usage include:
- Transparency: Clearly communicate methodologies and findings to the scientific community.
- Peer Review: Engage in thorough peer review to validate findings and minimize biases.
- Ethical Review Boards: Collaborate with institutional review boards to examine research proposals critically.
By fostering a culture of responsibility and ethical scrutiny in RNA research, the scientific community can advance knowledge while safeguarding individual rights and public trust.
Ethical considerations in RNA research are vital to balance scientific exploration and individual rights. Researchers play a crucial role in shaping the future of RNA data usage.
Challenges Facing RNA Databases
The evolving landscape of RNA databases presents both opportunities and challenges for researchers. Understanding these challenges is essential for optimizing data utilization and improving research outcomes. The importance of addressing these challenges lies in their potential impact on the efficacy of scientific inquiries and innovation in the field of molecular biology.
Data Overload
The phenomenon of data overload has become increasingly prevalent in RNA databases. As sequencing technologies advance, the volume of RNA sequences and related information expands dramatically. This deluge of data can lead to difficulties in managing and interpreting the information effectively. Researchers may find themselves overwhelmed by the sheer amount of data available, which can hinder their ability to extract meaningful insights.
To combat data overload, effective data management strategies are necessary. These strategies might involve:
- Implementation of sophisticated algorithms for data filtering.
- Development of intuitive user interfaces for better navigation.
- Integration of machine learning techniques to assist in data analysis.
The challenge also lies in ensuring that data quality is sustained amid the overload. Poor-quality data can lead to inaccurate conclusions, which is not acceptable in scientific research.
Data Accessibility Issues
Another significant challenge is data accessibility. Despite the vast amounts of data stored in RNA databases, not all researchers can access the information they need. This limitation stems from various factors, including:
- Proprietary restrictions that prevent public access to certain datasets.
- Even when data is publicly available, technical difficulties can arise. These may include slow download speeds, complex access protocols, or lack of compatible tools for different operating systems.
- Differences in institutional resources, where well-funded laboratories may have greater access to advanced tools and data analytics compared to smaller or underfunded research groups.
Efforts to improve accessibility can take various forms. For example, enhancing public databases with clearer user guidelines can promote broader usage. Furthermore, fostering collaborations between research institutions and database curators can create better infrastructure for data sharing.
Thus, tackling data accessibility issues is vital for fostering inclusivity in RNA research and ensuring that all researchers can contribute to advancements in the field.
Future Directions for RNA Databases
The evolution of RNA databases is poised at a critical juncture, where advancements in technology and data management can significantly enhance their performance and utility. Several key elements highlight the promising future of RNA databases, which is crucial in supporting the increasing complexity of molecular biology research. As these databases grow and evolve, the potential benefits of improved data retrieval and integration of diverse data types will greatly impact research capabilities.
Enhancements in Data Retrieval
One major direction for future RNA databases is the enhancement of data retrieval methods. Current databases often struggle with the sheer volume of data, making it challenging for researchers to find relevant information quickly. Future databases can adopt
sophisticated indexing and search algorithms to facilitate faster and more accurate data retrieval. Additionally, incorporating user-friendly interfaces will enable researchers to navigate complex datasets more efficiently.
Another aspect of improving data retrieval involves the use of machine learning algorithms. By applying artificial intelligence, RNA databases can learn user preferences and optimize search results based on past queries. This adaptability not only saves time but also increases the accuracy of the information retrieved, allowing scientists to focus on analysis rather than data scraping.
- Improved indexing
- User-friendly interfaces
- Machine learning application
"The future of RNA databases lies in their ability to provide precise and relevant information with unprecedented speed and efficiency."
Integration of Experimental and Computational Data
The future of RNA databases also involves a closer integration of experimental and computational data. As experimental techniques yield more extensive datasets, merging this data with computational analyses can offer deeper insights into RNA structure and function. This integration allows researchers to validate computational predictions with empirical evidence, enhancing the reliability of results.
Moreover, cross-disciplinary collaborations are essential for achieving this integration. By bringing together biologists, bioinformaticians, and data scientists, RNA databases can evolve to be more comprehensive and user-centric. The ability to incorporate different perspectives and expertise can foster innovative solutions for complex biological questions.
- Cross-disciplinary collaboration
- Validation of computational predictions
- Enhanced comprehensive databases
Closure
The conclusion section synthesizes an understanding of RNA databases and underscores their pivotal role in molecular biology and genetics. RNA databases are not merely repositories of data. They serve as the backbone for extensive research, allowing scientists to unravel complex biological questions. By providing access to various types of RNA, including mRNA, rRNA, and non-coding RNAs, these databases enhance the ability to conduct genetic analysis, functional studies, and comparative genomics.
Summarizing key points is essential here. The article has elucidated several crucial aspects:
- Types of RNA Databases: Different classifications like sequence, structural, and functional databases were discussed, highlighting how each type contributes to specific research needs.
- Data Collection Methodologies: Emphasis was placed on experimental and computational approaches that ensure the quality and relevance of data within these databases.
- Applications of RNA Databases: Key uses in genetic research, disease studies, and pharmaceutical development affirm their importance in practical settings.
- Challenges and Future Directions: Future enhancements and integration of data retrieval systems point towards the evolution of how these databases will function in the rapidly advancing field of molecular biology.
To encapsulate these points, RNA databases not only store genetic information but also foster collaboration and innovation in research disciplines. They are indispensable in the quest to understand biological systems and health-related phenomena better.
The Role of RNA Databases Going Forward
Looking ahead, RNA databases are expected to undergo significant transformations driven by technological advancements. The integration of Next-Generation Sequencing (NGS) and artificial intelligence (AI) can enhance data analysis capacity and accessibility. Here are a few considerations on how RNA databases might evolve:
- Enhanced Data Retrieval: Innovative search algorithms and user-friendly interfaces may allow researchers to access relevant RNA sequences more efficiently, shaping targeted research outcomes.
- Interconnectivity with Other Databases: Future RNA databases will likely see improved interoperability with protein and genomic databases, leading to a more holistic view of biological processes.
- Support for Collaborative Research: As global scientific partnerships increase, RNA databases could serve as platforms for sharing findings, thus accelerating breakthroughs in understanding diseases.