Exploring Open Source Analysis: Insights and Impacts
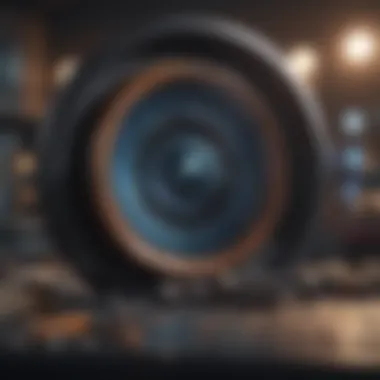
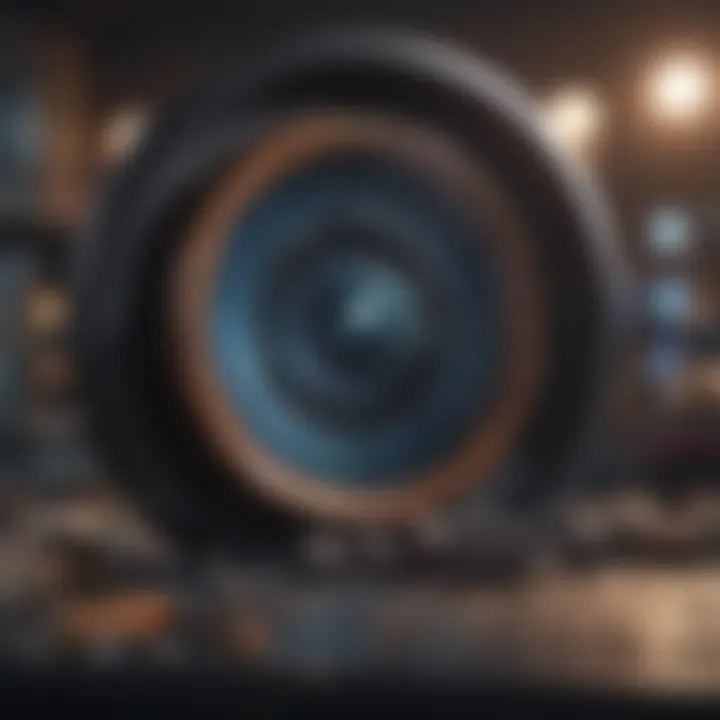
Intro
In todayâs interconnected world, the practice of open source analysis has taken center stage across various sectors. With rapid advancements in technology and an increasing need for transparency, this approach has morphed into a pivotal tool for extracting insights from publicly available data. Whether it's in cybersecurity, data intelligence, or even scientific research, understanding the multifaceted nature of open source analysis is crucial. It's like mining for gold; while the initial prospect may seem overwhelming, with the right techniques, valuable resources can be unearthed.
As we peel back the layers of this intricate domain, we will explore how professionals are leveraging open source methods. Weâll sift through both opportunities and concerns, uncovering the ethical ramifications that undoubtedly arise in this landscape. The goal here isnât just to skim the surface; weâre diving deep into the nitty-gritty of how these methods apply across diverse disciplines and practices. So, grab your analytical hats, and letâs navigate this compelling terrain.
Prelude to Open Source Analysis
Open source analysis has quietly emerged as a pivotal component in various fields, adding depth to our understanding of data and how it can be interpreted. Today's world bombards us with information, and sifting through the noise to find actionable insights has become a necessity. From cybersecurity to market research, the principles of open source analysis provide a structured approach to harnessing information for informed decision-making. Here we will cover how it not only aids in problem-solving but also brings ethics and legality to the forefront, especially as data privacy becomes more critical.
Defining Open Source Analysis
Open source analysis refers to the practice of collecting and interpreting publicly available information from diverse sources. This may include anything from social media posts to governmental databases, and academic publications to news articles. Itâs like mining gold from a river â one has to know where to look and how to sift through the sediment to find valuable nuggets. The versatility of open source analysis lies in its methodologies which range from quantitative to qualitative approaches, each suited for different types of inquiries.
It plays a central role in various sectors, especially those requiring situational awareness and strategic insight. Importantly, the data does not come from secretive or proprietary databases; rather, it thrives on transparency and accessibility. Therefore, one can glean information not only from classic sources but also from user-generated content, making the analysis rich and often more relatable.
Historical Context and Evolution
The roots of open source analysis can be traced back to military intelligence in the second half of the 20th century. Initially, countries relied heavily on clandestine methods to gather intelligence, which often limited their information pool. The shift began with the rise of the internet in the late 20th century. Suddenly, vast amounts of data were at oneâs fingertips, allowing analysts to pivot towards a more accessible, open-source framework.
As technology advanced, so did the tools available for analysis. In the early days, one had to rely on manual methods and basic databases. Fast forward to today, and we find sophisticated analytics tools that harness machine learning and AI to process and analyze this treasure trove of data more efficiently. With information dissemination becoming a lightning-fast endeavor, the importance of timely open source analysis cannot be understated. It allows organizations to stay ahead of trends and threats, adapting swiftly to the ever-changing landscape of information.
In essence, open source analysis evolved from a niche practice to a fundamental pillar across various domains. The integration of diverse data types enhances the scope and reliability of insights produced, providing a more nuanced understanding of complex issues. Following this historical tapestry, one can appreciate both the evolution of the practice and its ongoing relevance in an increasingly interconnected world.
Key Concepts and Terminology
In the realm of open source analysis, grasping key concepts and terminology is crucial. Understanding these essential elements not only enhances comprehension but also empowers practitioners across various fields to effectively leverage open source data for their specific needs. Moreover, familiarizing oneself with this vocabulary improves communication among professionals, fostering an environment of clarity and collaboration.
Open Source Intelligence (OSINT)
Open Source Intelligence, abbreviated as OSINT, refers to the process of collecting and analyzing publicly available information from various sources. This encompasses traditional media, social media platforms, websites, and even academic publications. The significance of OSINT lies in its ability to provide actionable insights without breaching any privacy laws or ethical boundaries. By tapping into this wealth of information, organizations can maximize their situational awareness, enhance decision-making, and identify emerging trends or threats.
Creating a robust OSINT framework involves several key components:
- Source Identification: Recognizing reliable sources of information is fundamental. Not all data available online holds the same weight or credibility. Citing authoritative sources adds legitimacy to the findings.
- Data Gathering Techniques: Familiarity with various online tools such as web scraping, API access, or even manual searches can vastly improve the efficiency and scope of data collection.
- Analysis and Interpretation: Once data is gathered, transforming it into meaningful insights requires analytical skills and understanding of contextual relevance.
In an era where information is at our fingertips, OSINT is not just useful; it is becoming indispensable for professional analysts.
Data Sources and Repositories
The landscape of open source analysis is cemented by a myriad of data sources and repositories. These platforms serve as the backbone for extracting relevant insights. Key sources include:
- Social Media: Platforms like Twitter, Facebook, and Reddit can provide real-time discussions, sentiment analysis, and trend tracking. Monitoring these channels can reveal public opinion and emerging narratives.
- Government Publications: Various governmental bodies publish reports, statistics, and datasets. Accessing these can aid in understanding demographic trends and regulatory landscapes.
- Open Databases: Websites like data.gov and open-data.eu offer vast repositories of publicly available datasets covering different sectors from health to finance.
Since the integrity of analysis largely hinges on the quality of data, being discerning about sources remains imperative.
Analytical Tools and Techniques
When diving into the depths of open source analysis, utilizing the right analytical tools and techniques can make a significant difference in the efficacy and accuracy of insights derived.
Some widely used tools in the OSINT community include:
- Maltego: This tool is particularly valuable for visualizing complex relationships between data points, making it easier to draw connections and patterns.
- Hunchly: Designed specifically for web-based research, this tool helps in capturing online sources systematically, organizing them efficiently for analysis.
- IBM Watson Discovery: A powerful tool that leverages AI and machine learning to uncover insights within large datasets, thus enhancing the analytical process.
Specific analytical techniques include quantitative analysis for numerical data while qualitative analysis focuses on understanding subjective elements like opinions or sentiments. The synergy between qualitative and quantitative approaches can yield a more comprehensive perspective on any research question.
"The best insights often come from a blend of various analytical lenses, honing in on both the numbers and the human narratives behind them."
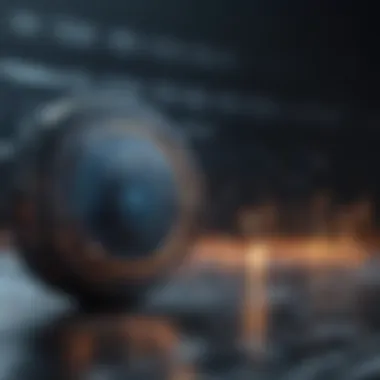
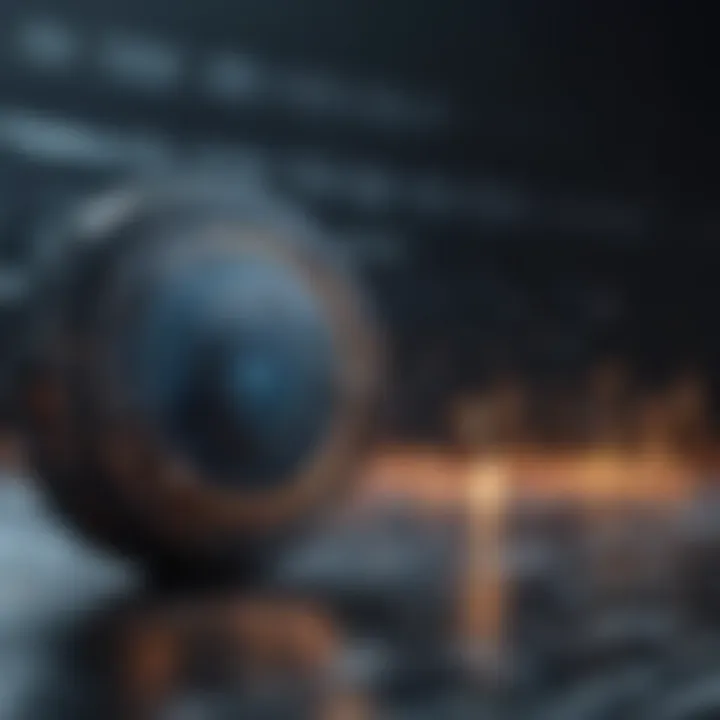
Through an awareness and application of these key concepts, terms, and tools, those engaged in open source analysis can efficiently navigate the vast ocean of information available, translating it into actionable intelligence.
Methodologies in Open Source Analysis
Understanding the methodologies in open source analysis is vital for anyone wishing to harness the power of publicly available information. The landscape of data is continually expanding, and several methodologies have been created to sift through this vast ocean of information, cultivating rich insights and guiding actionable decisions.
The methodologies serve as a compass. They not only define how to approach open source data but also emphasize the significance of structured analysis. By applying these methods, practitioners can avoid the pitfalls of information overload, ensuring their analyses are both comprehensive and coherent. Also, recognizing the methodologies enhances both the efficiency of the process and the reliability of the outcomes, a must in todayâs fast-paced world.
Qualitative vs Quantitative Approaches
When we delve into the world of open source analysis, itâs crucial to differentiate between qualitative and quantitative approaches. Each method brings a unique set of strengths to the table.
- Qualitative Approaches: These focus on understanding the underlying patterns and themes within the data. They often involve subjective analysis, relying heavily on the interpretation of context, tone, and implications. For example, analyzing discussions on platforms like Reddit or Facebook allows analysts to capture the mood of public opinion regarding a specific issue rather than just hard numbers.
- Quantitative Approaches: These methods are more concerned with numerical data and statistical relationships. They often employ tools for data mining to analyze trends, such as web traffic analytics or survey results. This approach can provide a clear picture of what is happening, supported by figures and trends that reveal broader patterns.
In practice, the choice between qualitative and quantitative approaches should be dictated by the specific questions posed. Thereâs merit in a blended approach, where qualitative insights inform numerical data interpretations, allowing for more nuanced conclusions.
Frameworks for Effective Analysis
Effective frameworks are what transform raw data into useful outcomes. In open source analysis, a strong framework can act as a roadmap, guiding the researcher through their exploration while ensuring consistency and repeatability.
Several frameworks have emerged, but they generally share common features:
- Clear objectives: Defining goals is paramount. Analysts must ask what they hope to achieve from their analysis.
- Data collection processes: Establishing consistent methods for sourcing data ensures reliability. Using platforms like en.wikipedia.org or britannica.com can be effective, as they are reputable sources of verified information.
- Analytical techniques: Identifying the right tools to interpret data is essential. From visualization tools to statistical software, employing suitable techniques can enhance understanding.
- Evaluation and iteration: Constantly reviewing findings allows for adjustments to be made mid-process, ensuring outcomes meet objectives.
Using frameworks helps maintain clarity in analysis, allows for replicability in approaches, and facilitates better communication of findings.
Integrating Open Source with Traditional Methods
The integration of open source methodologies with traditional methods can yield robust analyses, fostering a more comprehensive understanding of data. While traditional methods have their strengths, such as rigorous experimental design and established protocols, they can be complemented effectively by open source approaches.
- Combining Data Sources: Traditional methods often rely on controlled environments and limited datasets. Open source analysis expands the horizons. By integrating publicly available data, researchers can get a more holistic view.
- Enhanced Contextual Understanding: Traditional methods might miss out on societal factors influencing data. Open source provides a narrative that can inform these external contexts.
- Accelerated Research Processes: Traditional research can be slow and cumbersome. By leveraging open source tools, researchers often find they can gather and analyze data much more swiftly.
In summary, integrating these methodologies not only boosts efficiency but also enhances the depth of the analysis. The combined insights gleaned from both domains result in a richer, more informative understanding of the researched topic, providing a better foundation for decision-making.
Applications Across Various Fields
Open source analysis has blossomed into a versatile tool used across a multitude of disciplines. Its capacity to harness publicly available data not only strengthens research methodologies but also creates powerful insights that can lead to impactful decisions. When we delve into the applications of open source analysis, itâs essential to recognize how each field distinctly benefits from these practices. From cybersecurity to market research and even scientific inquiry, the implications and capabilities of this analysis can make a significant difference.
Cybersecurity and Threat Assessment
In an age where cyber threats loom large over organizations and individuals, open source analysis has become a game-changer in cybersecurity. By exploiting data gathered from social media, forums, and other digital channels, analysts can track potential threats much before they materialize. The ability to glue together various snippets of information offers a clearer picture of an evolving threat landscape.
- Threat Hunting: Cybersecurity professionals utilize open source intelligence (OSINT) to hunt down possible vulnerabilities and threats actively. The intelligence gathered can pinpoint specific attack vectors or identify potential attackers.
- Incident Responses: During incident response, open source methods provide crucial information that can help in understanding the context of a breach or an attack. By analyzing chatter, posts, or even code repositories, experts can piece together actions and intentions of malicious actors.
Itâs akin to being a detective, piecing together clues from vast spaces of information. By using open source analysis, security teams can not only react to incidents but can also proactively strengthen defenses.
Market Research and Competitive Intelligence
For businesses looking to carve out their niche in competitive landscapes, open source analysis can be the secret sauce. Knowing what competitors are up to or emerging market trends can light the path for strategic planning. Here, a wealth of public data gives companies a leg up.
- Consumer Insights: Companies sift through reviews, forums, and social media to gather insights into consumer behavior. Understanding customer sentiment can guide product development and marketing strategies.
- Competitive Analysis: Keeping tabs on competitor movements is paramount. Open source tools allow businesses to track competitor announcements, blog posts, and interactions with customers, providing a comprehensive understanding of their strategy.
By analyzing these data datasets, organizations can make informed decisions about how to position themselves in the market. The intelligence gathered can shape product offerings and tailor messaging that resonates with potential customers.
Scientific Research and Data Gathering
In scientific fields, open source analysis facilitates research while fostering collaboration among scientists. This method of gathering data from various sources enriches research outputs and speeds up discovery processes.
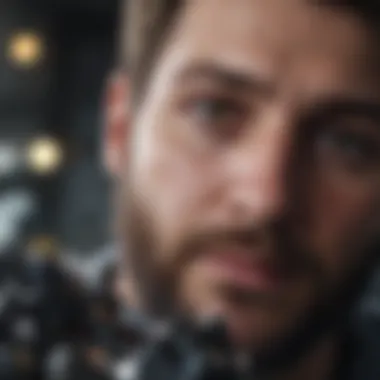
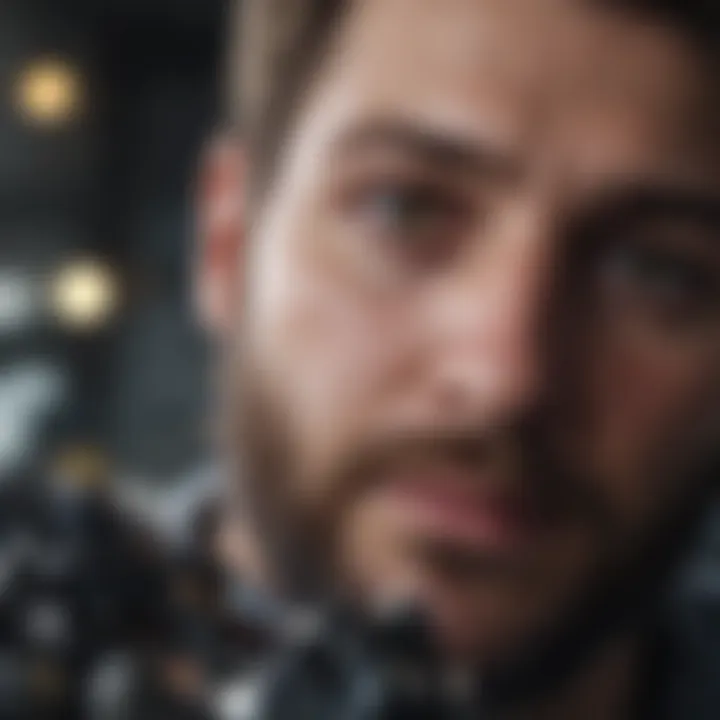
- Data Sharing: The accessibility of public datasets means researchers can dive deep into historical data or recent studies without reinventing the wheel. This pooling of knowledge often leads to innovative solutions.
- Collaborative Projects: Open collaboration streams, such as shared GitHub repositories, make it possible for scientists across the globe to contribute to projects and share findings. This can enhance data quality and validity.
In essence, open source analysis acts as a bridge, connecting diverse research efforts in a shared web of knowledge. By utilizing these tools, the scientific community can accelerate advancements and contribute to global knowledge in significant ways.
Ethics and Legal Considerations
In the realm of open source analysis, where insights can be gathered from a multitude of easily accessible public resources, the ethical and legal frameworks governing these actions are critical. Practitioners involved in this analytical practice must navigate a labyrinth of regulations that aim to protect personal privacy, respect intellectual property, and uphold ethical standards. Understanding these principles is not merely good practice; it's essential for maintaining the integrity of the analysis and ensuring that the gathered data is used responsibly.
Privacy and Data Protection
With the ever-increasing volume of data available online, the importance of privacy and data protection cannot be overstated. Individuals are often unaware of how their information is utilized, making it paramount for analysts to adopt rigorous privacy measures.
- Data Minimization: When collecting data, it's prudent to only gather what is necessary to meet the objective of the analysis. This principle ensures that personal information is not unduly exposed or misused.
- User Consent: Gaining explicit permission from users whose data may be collected or analyzed is a crucial step in ensuring compliance with data protection regulations.
- Anonymization: When possible, transforming identifiable data into non-identifiable formats can provide a layer of security for sensitive information.
Individuals and organizations should be aware of GDPR (General Data Protection Regulation) and other regional laws that impose strict guidelines. Ignoring these laws could not only lead to legal repercussions but may also damage reputation and trust.
Intellectual Property Issues
The digital age has blurred the lines between public and proprietary data, forcing analysts to tread carefully when using information sourced from the internet. Recognizing and respecting intellectual property rights is essential to maintain ethical standards in open source analysis.
- Fair Use: Understanding the distinction between fair use and infringement is vital. Analysts should familiarize themselves with copyright laws to determine what can be legally used without permission, especially with materials like images, texts, or data sets.
- Attribution: When utilizing someone elseâs work, proper credit must be given. This not only complies with legal standards but also fosters a culture of respect within the analytical community.
- Licensing Agreements: Many open source materials come with specific licensing agreements. Reading and adhering to these agreements ensures that usage remains compliant and ethical.
A well-rounded analysis respects these boundaries and does not exploit proprietary information, ensuring all work is above board and informed by ethics.
Ethical Implications in Analysis
Beyond legal compliance, the ethical ramifications of open source analysis are weighty and multifaceted. Analysts hold a moral responsibility to conduct their work in a manner that is fair and just.
- Accuracy: Analysts are tasked with the duty to report findings truthfully. Misleading conclusions drawn from improperly verified data can have dire consequences, damaging credibility and trust.
- Bias and Objectivity: Being aware of oneâs biases is crucial. Analysts must strive to approach their work with an objective lens, avoiding the temptation to selectively present data that aligns with preconceived notions.
- Impact on Society: The outcomes of open source analysis can shape public perception, influence policy, or even put individuals at risk. Therefore, it is imperative that analysts consider the broader implications of their work on various communities.
"Ethical analysis is not merely an added layer but the very foundation on which robust analysis stands. Without it, the integrity of findings is compromised."
In summary, the ethical and legal considerations within open source analysis forge a significant pathway toward responsible practices. Adopting an understanding of privacy laws, intellectual property rights, and ethical practices not only enhances the quality of analysis but also contributes to a more trustworthy environment for all spectators in this expansive domain.
Challenges in Open Source Analysis
When we dive into the complexities of open source analysis, we invariably stumble upon various challenges that significantly influence its effectiveness. These hurdles, ranging from data reliability to the sheer amount of information available, shape the methodology and thought process of analysts. Addressing these challenges is essential not just to bolster the integrity of the insights derived, but also to ensure that stakeholders can make informed decisions based on solid foundations. Let's break down some of these core issues.
Data Reliability and Verification
In the realm of open source analysis, one of the fundamental pillars is the reliability of the data being scrutinized. Given the vast oceans of information available onlineâfrom social media to public databasesâensuring the authenticity of these sources becomes imperative.
Analysts must question where the data comes from, who produced it, and whether it's being presented in a context that maintains its integrity. Verifying data entails cross-referencing information from multiple platforms, considering both governmental websites and user-generated content. It's not just about conducting a simple fact-check; itâs about ensuring every aspect matches up across the board.
Some key steps analysts can take include:
- Develop a framework for source evaluation that considers both authority and accuracy.
- Utilize tools or platforms that specifically assist with fact-checking, adding an extra layer of credibility.
- Implement peer review processes when gathering analysis to ensure that another set of eyes evaluates the data chosen.
By focusing on these strategies, analysts can significantly reduce the noise and hone in on credible data capable of supporting valid conclusions.
Overcoming Information Overload
With a wealth of information at one's fingertips, there's a paradox in open source analysis; while the abundance of data should ideally enhance understanding, it often leads to information overload. This overwhelming influx can drown analysts, making it challenging to extract actionable insights.
To combat this, analysts should:
- Prioritize quality over quantity in data selection; sometimes fewer, more relevant pieces of data can yield better insights than a mountain of unnecessary details.
- Employ organizational tools like spreadsheets or specialized software that can filter and categorize data.
- Set specific objectives for the analysis. Establishing clear goals can help cull irrelevant information and keep the focus on what truly matters.
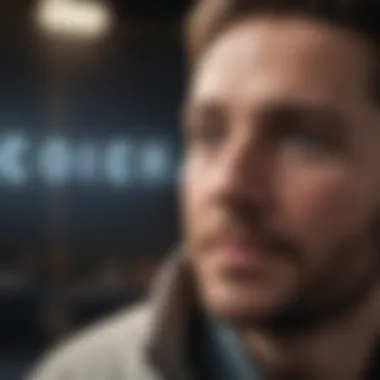
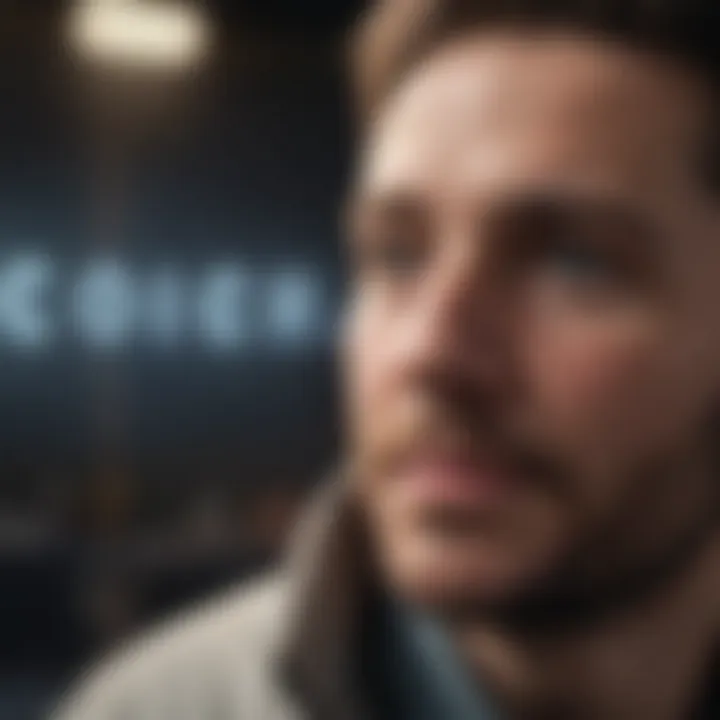
By laying out a plan and narrowing the scope, analysts can effectively streamline the data they engage with, turning potential chaos into clarity.
Navigating Misinformation and Bias
In an age where misinformation proliferates at an alarming rate, open source analysts must be adept at recognizing and navigating these pitfalls. Misinformation can come in many formsâbe it deliberate lies or simple errorsâand identifying bias in sources is equally important. The risk is that misinformation can skew public perception or lead organizations astray.
To mitigate these risks, analysts should:
- Continuously educate themselves on common misinformation tactics and trends in digital media.
- Diversify their sources; relying on a variety of viewpoints can help minimize bias and give a more rounded perspective.
- Regularly reassess the credibility of established sources, as reputations can change quickly in a rapidly shifting landscape.
By applying these principles, analysts will be better equipped to navigate the tricky waters of open source analysis. Ultimately, acknowledging these challenges and proactively addressing them is essential for producing reliable and insightful analysis, which can significantly influence decision-making in various fields.
Future Trends in Open Source Analysis
As the digital landscape continually unfolds, open source analysis is set to evolve in fascinating ways. This sector remains significant not only for its adaptability but also for its role in shaping decision-making across various domains. Exploring future trends helps us understand the transformative forces at play and the potential advantages and considerations inherent in utilizing these innovative methodologies.
Emerging Technologies and Tools
Open source analysis is intersecting more with cutting-edge technologies, giving rise to tools that enhance data gathering and interpretation. For instance, Blockchain technology is making waves. It's not just about cryptocurrency anymore; the integrity of data stored in a blockchain can be invaluable in ensuring the reliability of open source information.
- Data Visualization Tools: Tools like Tableau and Power BI assist analysts by beautifully presenting complex datasets, making them easier to understand and derive insights from.
- Natural Language Processing (NLP): With the rise of tools that utilize NLP, sifting through large volumes of textâwhether tweets, comments, or reportsâbecomes more efficient. These tools can help in sentiment analysis, giving a clearer picture of public opinion surrounding certain events or topics.
"Emerging technologies are not just trends; they are pathways to redefining how we perceive and utilize information in our decision-making processes."
The Role of Artificial Intelligence
Artificial Intelligence (AI) is no longer just a futuristic concept; it is increasingly intertwined with open source analysis. AI algorithms can sift through data sets at lightning speed, identifying patterns or anomalies that may be difficult for human analysts to catch.
- Predictive Analytics: AI can forecast trends by analyzing historical data, helping organizations prepare for future scenarios.
- Automated Reporting: By harnessing AI, practitioners can automate the reporting process, freeing up valuable time for focused analysis and strategic thinking.
Moreover, with AI allowing for real-time data analysis, decision-makers can respond to developments as they unfold, boosting the responsiveness of intelligence operations.
Potential Growth in Collaborative Analysis
The future of open source analysis is bound to feature a marked increase in collaboration. We already see various entitiesâbe it organizations, governments, or individualsâcoming together to share insights and data.
- Open Source Communities: Networks like GitHub enable coders and researchers to collaborate on projects, thereby enriching the analytical frameworks used in open source analysis.
- Cross-Disciplinary Approaches: Engaging with multiple disciplines can uncover unique perspectives and solutions. For example, combining insights from sociology with data analysis can lead to innovative ways of interpreting social media data.
The implication for future research and operations is clear: collaborative analysis will not only increase efficiency but also enhance the quality of insights derived from shared data.
Culmination and Reflection
The examination of open source analysis comes full circle in this section, where we grapple with the implications of key findings. Grasping the nuances of open source analysis isn't just a matter of conceptual knowledge; itâs about recognizing its real-world significance in various fields. As practitioners and enthusiasts alike contribute to a growing body of knowledge, they stand at the forefront of innovation. This article not only highlights the breadth of methodologies employed but also underscores the ethical considerations that serve as the backbone of responsible analysis.
In todayâs information-rich world, having access to advanced tools and inclusive data sources is paramount. But itâs equally essential to ensure that this information is reliable and utilized ethically. Practitioners must navigate a complex landscape, acknowledging the benefits of open source while making critical decisions that avoid the pitfalls of misinformation and bias. Understanding the intersection of open source methods and traditional practices opens avenues for more informed decision-making, ultimately leading to better outcomes in research, cybersecurity, and beyond.
"Informed decisions stem from careful analysis of the available data, and our understanding of that data begins with clear methodologies."
Summarizing Key Findings
The article has traversed various dimensions of open source analysis, shedding light on its methodologies, its practical applications, and its ethical implications. Key findings include:
- Diverse Applications: Open source analysis serves myriad purposesâfrom cybersecurity threat assessments to market research. Each field demands a tailored approach that maximizes the usefulness of data.
- Methodological Rigor: Qualitative and quantitative methodologies both play essential roles in ensuring robust analysis. Combining these methods enhances the depth and accuracy of findings.
- Ethics Matter: Navigating the open source landscape comes with advantages and drawbacks. Attention to privacy and data protection, as well as intellectual property rights, cannot be overlooked.
- Future Possibilities: Emerging technologies and the increasing role of artificial intelligence set the stage for further evolution in open source analysis methodologies.
Through the synthesis of these aspects, itâs clear that open source analysis isn't merely a toolâitâs a critical enabler of informed decision-making in a world where data flows abundantly.
Implications for Future Research
The insights offered by this article beckon further inquiry. As open source analysis evolves, several implications for future research emerge:
- Integration of AI Technologies: The increasing incorporation of artificial intelligence can facilitate deeper data insights and improved accuracy in analyses.
- Evolving Ethical Standards: Continued dialogue around ethical challenges will be necessary as technology advances, emphasizing the need for policies that guide responsibly utilizing open source information.
- Focus on Data Verification Methods: Future research should aim to develop and refine methodologies for verifying data reliability, thereby minimizing the impact of misinformation.
- Collaborative Research Models: Exploring how collaboration among researchers can enhance the quality and breadth of open source analysis presents an exciting avenue for future inquiry.
Itâs important for scholars and practitioners to remain engaged with these themes, ensuring that the open source landscape is navigated not just with curiosity but also with caution and integrity.